AIMinds #037 | Scott Stephenson, CEO at Deepgram
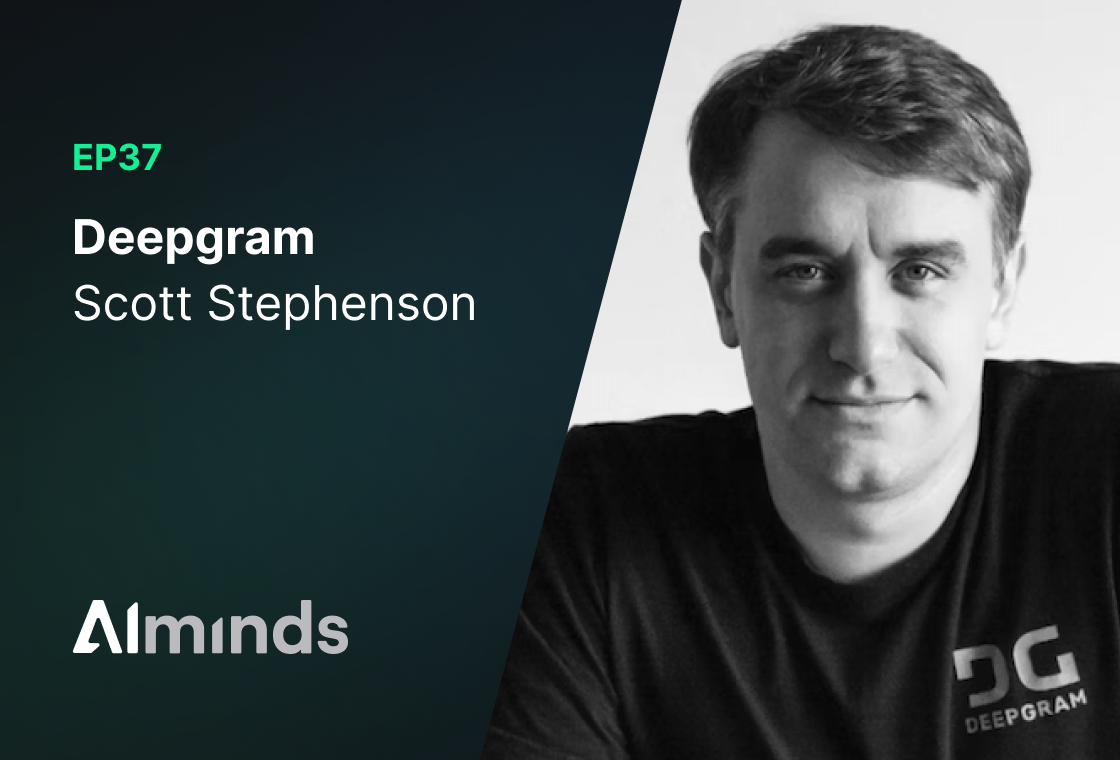
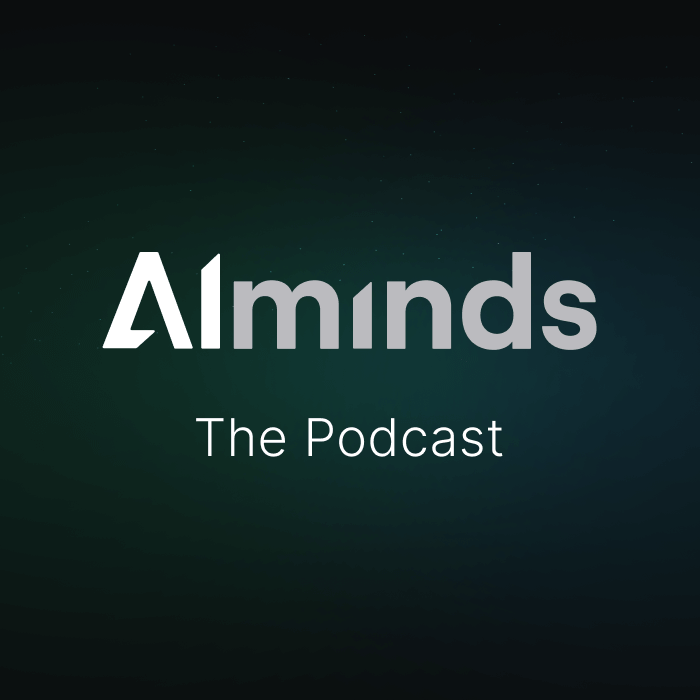
About this episode
Scott Stephenson is the CEO of Deepgram, transitioning from dark matter physicist to deep learning entrepreneur. He earned a PhD in particle physics from the University of Michigan, where he built a lab two miles underground for dark matter detection.
Scott left his postdoctoral research position to co-found Deepgram, focusing on innovations in speech-to-text technology.
Listen to the episode on Spotify, Apple Podcast, Podcast addicts, Castbox. You can also watch this episode on YouTube.
In this episode of the AI Minds podcast, we welcome a very special guest in Scott Stephenson, CEO and co-founder of Deepgram.
Scott shares with us his journey from particle physicist working on dark matter detection experiments to leading a high-growth AI startup.
He goes on to reveal how Deepgram emerged from an interest in analyzing personal audio data, unveiling a significant gap in advanced audio AI technologies.
Scott contrasts academia and startups, highlighting how startups can achieve greater efficiency and innovation due to reduced fundraising time. He also shares unique aspects of Deepgram's culture, including an engaging onboarding video that fosters creativity and a strong emphasis on documentation to support their remote-first policy.
Scott describes Deepgram's first-principles approach to innovation in speech and audio processing, focusing on long-term scalability and continuous reinvention. He explains how the company ensures its technology meets current needs while remaining future-proof, potentially supporting thousands of languages and improving processing speeds.
The conversation covers the strategic use of machine intelligence to enhance human capabilities, balancing rapid innovation with reliability, and illustrating how deep tech and machine learning can tackle complex challenges in the AI domain.
Fun Fact: To set a memorable tone during the onboarding process, Scott Stephenson created an onboarding video where he can be seen eating a ghost pepper. This unique method highlights his willingness to embrace extreme and stressful situations for the company.
Show Notes:
00:00 Leading speech-to-text API, expanded to multiple products.
04:55 Quick analysis via machine learning and simulation.
08:29 Start from first principles; identify inefficiencies and innovate.
10:29 Bet on scalable technology for long-term solutions.
15:10 Innovate constantly for faster, impactful company existence.
17:17 Incorporating machine intelligence can significantly accelerate progress.
21:13 Discipline, customers, deadlines, teamwork, machine intelligence, future adaptability.
24:06 Promote continuous learning to keep intelligent employees.
29:27 Intelligent tools aid information retrieval in businesses.
30:32 Store ideas now; revisit and use later.
More Quotes from Scott Stephenson:
Transcript:
Demetrios:
Welcome back to the AI Minds podcast. This is a podcast where we explore the companies of tomorrow built AI first. I'm your host, Demetrios, and this episode is brought to you by Deepgram, the number one speech to text and text to speech voice API on the Internet, trusted by the world's top conversational AI leaders, enterprises, and startups, some of which you may have heard of. I'm talking about Spotify, Twilio, NASA, and Citibank. Today, we are joined by a very special guest, the CEO and co founder of Deepgram itself. What's going on, Scott? How you doing.
Scott Stephenson:
Man? Not much. We're a lot, depending on how you look at it. I'm doing great. Thanks for having me on. Yeah.
Demetrios:
Well, I'm excited to chat with you all about the inception of Deepgram, all the way into what it is today and the culture of Deepgram, because as we were talking about before we hit record, we've both got our point of views on the company. And so I think it would be really cool to talk about the different lived experiences that we've had. Maybe you can start us off with your journey and where you started in order to get Deepgram to become a thing like it came from an idea. What was that?
Scott Stephenson:
Yeah, we're nine years old as a company now, and the first about four years of that was a deep research phase before we released our product at scale. And that's what a lot of people know us for. Our speech to text in real time and batch mode and 30 plus different languages. And we worked on that from 2015 all the way up to 2018, 2019, and then released it. And that time period, it was just a really small number of people, like eight people or less. And then after that was the high growth phase of Deepgram, where we're hiring a lot of folks. There's a lot of people using us as a speech to text API.
Scott Stephenson:
And then now we're the number one speech to text API in the world. And we have now expanded to other products as well, like text to speech. And now, just in the last few days, we've released our agent API as well. But what got me started down this path was I was a particle physicist, so I built deep underground dark matter detectors, and I was a graduate student working on an experiment called Panda X. And it was a joint experiment between the US and China. And I was at University of Michigan doing my PhD there in physics, and we were partnering with universities in China, and the experiment was located in China as well. There are a lot of advantages to that. Things can move fast in China.
Scott Stephenson:
Also, material cost is cheap in China, etcetera. And a lot of the folks who would actually build the lab and all the infrastructure around it, it can move a lot faster, and you can keep the costs low in China. Because of that, we were able to make the world's most sensitive detector in the mass range that we were looking at for this dark matter detector. And we did it in about four years, which is basically three times faster than hurt us, any of our competitors. Yeah. And I was so lucky to be part of that. We were a really small team of around maybe 15 to 25 people. It kind of grew over time, but I didn't realize that.
Scott Stephenson:
I wasn't really quite aware, cognizant at the time of what was happening. But we had a budget of around like 20-25 million, and it's 15 to 25 people working over the span of four years to build the world's best thing in a deeply technical area. Sounds like a startup, doesn't it? And that's basically what it was. It was like this startup dark matter experiment that started from nothing, where we had to negotiate and figure out where to put the experiment, get the lab built. We had to do like the h vac, like the air conditioning and heating and all sorts. We had to figure out everything. We had to get electricity there. We had to run our own fiber Internet.
Scott Stephenson:
We had to set up our own compute cluster underground. And underground is hot. We're 2 miles underground, and it's 90, 95 degrees. And then you have machines running in there, and so you have to get the heat out. And so anyway, you're in this very interesting situation. We had to problem solve and figure it all out. We had to simulate the experiment, then build the experiment, then run the experiment, then take data, then analyze the data, and then see if we have a world class result. And we did have a world class result.
Scott Stephenson:
So that was really awesome. We were able to do it in such a short period of time. But one of the reasons that we were able to do it in such a short period of time is that the analysis phase didn't need hundreds or thousands of people to do it like a, like many particle physics experiments would need. We used, we only had a few people doing the analysis, and we used a lot of machine learning to help us. We simulated data, and we used a lot of machine learning in order to understand this large amount of data that we were taking with this experiment. And one very interesting thing about it is the type of data that we were taking was waveform data. It looks a lot like an audio signal. It wasn't an audio signal, but it was analog signals that looked a lot like an audio signal.
Scott Stephenson:
And so we had all this experience just working with machine learning and this type of signal processing and trying to find interesting things happening in this data. And I thought it was just so cool that we were doing this. Like, who gets to do this? We're basically in a James Bond lair 2 miles underground, building all this stuff. This is, this is insane. And I'm just thinking we should like document this somehow, you know? And so that was myself and my co founder Noah thinking about this. And we built a little device with a microphone, a battery, a Wi Fi antenna, and just a little chip that is basically made out of a raspberry PI for anybody that's familiar with that. And we just recorded audio all day, every day, 24/7 always going. And then it would upload to the cloud and just store the audio there.
Scott Stephenson:
After we did this for months, we accrued hundreds or over a thousand hours of audio. We had a problem, which is we're not going to go back and listen to our lives again. That would take your entire life to do it. So that put us in analysis mode then. Well, how could we analyze this few hundred hours or 1000 hours of data? And maybe folks would think your first instinct is like, well, let's build something. But actually, as scientists, you're usually very pragmatic. You think, is there some other tool out there in the world that already does this? And so we went looking for that tool, and we found some of the legacy solutions. We found some of the large hyperscalers that were working on certain things.
Scott Stephenson:
But when we looked at the capability of them, we were like, this doesn't match up with our experience in particle physics. What we were doing in particle physics is way more advanced than this speech technology that we're seeing. So after noticing that, we thought, wow, this is a massive opportunity for a company to start an end to end, deep, learning based, data driven audio AI company that can understand what's inside audio and then eventually produce it, and then eventually also just have entire conversations that are automated using this entire technique. We started working on that in 2015. We were a YC company, got investment for our seed for that, and then we built for those four years like I talked about. And then once we release the product, now we're in this like high growth mode where we have a. Like we raise a series a, then we raise a series b. And, you know, now you see the company, how it is.
Scott Stephenson:
Now we're around 100 people, and I think a really great group of folks who are just tackling a very difficult problem.
Demetrios:
So there was inevitably a whole lot of complex problems that you had to figure out when you were putting a bunker underground and testing these physics particles. How do you go about solving these complex problems?
Scott Stephenson:
One of the most valuable tools in a physicist toolkit is just starting from first principles. You just say, like, what are the laws of nature here? What are the possible energy scales or dollar scales or human hour skills, or. And then look at it from a practical perspective to what processors are available, how much memory storage is available, what has already been built by other people, et cetera. And you just kind of squint and look at the world and just say, okay, I think there's massive inefficiencies in this area. And so the areas where I think actually the world's kind of efficient, given the first principles way of looking at it, or the tools that have already been built or whatever, don't go innovate there. Don't do that. But then this other area where we see an order of magnitude or two orders of magnitude or three orders of magnitude of inefficiency, that's where we're going to go. But usually where there is ten x or 100 x or 1000 x efficiency gain, and it hasn't been done yet, that means that there's some kind of roadblock in the way, because otherwise people would have already done it.
Scott Stephenson:
And so that means you're signing up for, like, this hard battle. And for us, it was, you know, inventing the techniques for doing end to end, deep learning for speech. But what that meant was not using any of the tools that were already built. So anybody who's familiar with speech from that time period would know that gaussian mixture models were very popular. Ngram models were popular for language models, there were way to find it. State transducers were popular. They're all these mixtures of different components in a pipelined approach, and you basically just have to say, we're going to throw it all away. We're not going to use any of it.
Demetrios:
This was after testing. This was after doing research, seeing that those weren't working.
Scott Stephenson:
We try all the different systems, and we try to extract as much as we can in a short period of time, work on it for a few weeks, maybe a few months or something, right? And say, hey, can we definite state transducers do what we need? But it's not just do what we need today. It's do what we need ten years from now, five years from now, because we want to bet on an underlying technology that will scale over a long period of time. It's not just can we solve somebody's problem right now? If we were thinking about it from that perspective of can we just solve somebody's problem right now? Anybody else can do that, you know, the hyperscalers or whatever, that's what they're doing. We're thinking, no, we're starting a totally different company here, which is a deep scientific technical bet that data driven, end to end deep learning is going to revolutionize how all this works. It's going to give you ten x 100 x 1000 x, like different scaling. And if what we're working on doesn't have the potential to do that, why are we working on it? That doesn't make sense. I think there is. In any type of technology, there's like a scientific phase, and then there's an engineering phase.
Scott Stephenson:
And in the scientific phase, you're talking about orders of magnitude, and in the engineering phase, you're talking about percents. And we're looking at the problem and saying, if this is just an engineering problem, there's no reason for us as scientists to be doing it. That doesn't make any sense. Right. But if this is a scientific problem and we're discovering the fundamental laws of intelligence and we're applying them, you know, using first principles, you know, and thinking about the energy spend to do it, and just all these principles, basically, then, you know, now that's something that's a job for us to do. If it's just, you know, getting the next 30%, squeezing the next 30% out of a certain architecture or something like that, that, I don't know, like, that's, that's, that's not my expertise. We have plenty of people now at Deepgram that, that do that kind of thing. Because our products, like our, for instance, like our speech to text, is now nine years mature from where we started back then.
Scott Stephenson:
But even then, we're still reinventing the architectures now, getting another order of magnitude or two x or something like that. And that's the science phase, and then there's an engineering phase after that that extracts the next bit of it. But, yeah, we wouldn't pursue this problem if there wasn't a ten x involved, basically.
Demetrios:
So one thing people recurrently tell me they love about Deepgram is how fast it is. I think that is something that is undoubtable. Was that, in your mind, from the beginning or when did you realize that was something that needed to be paramount to what you're doing?
Scott Stephenson:
Yeah, it was the most strike. So going back to the early days in Deepgram, and even now, it's one of the most striking things that you see when you try to use any tool, even like a professional API that was available then, or like an open source tool, or you try to build it yourself using old techniques that use a beam search and that kind of thing, and you're like, oh my God, this is so slow. It's taking an hour to process an hour, or it's taking 20 minutes to process an hour. How is this like a thing? There are many other more complicated things that we do in the world that do not take that amount of time to process. Also, just if you're thinking about what the world is going to need five years from now, ten years from now, 20 years from now, everybody is spending tons of compute to understand, just like a 1 hour meeting or something like that. It just doesn't make sense. It doesn't make sense from a dollar perspective. It doesn't make sense from a time perspective.
Scott Stephenson:
So you have to just kind of trust that. One way I like to think about it is, 30 years from now, do you believe that it's going to take 20 minutes to process an hour of audio? I do not believe that, no.
Demetrios:
No way.
Scott Stephenson:
Now, 30 years from now, do you believe that it's going to be just as like bad as it is now? I do not believe, like, from a, from an accuracy perspective, I do not believe that. 30 years from now, do you think that these services are only going to support 30 languages or accents? No, I think it'll support thousands. Right. So you, you start to, I think the 30 years timeline really helps you with breaking out of these like percent type, you know, improvements and you say, and then another good way to think about it as a company is, well, 30 years from now, all of those things are going to be there. Are we going to be the company to create it? You know, and, because somebody's going to do it, you know, somebody's going to do it. But are we the ones to create it? Okay. And if we are the ones to create it, we're the accelerators of creating it. Sure, 30 years from now, that may be the case.
Scott Stephenson:
Just naturally. If, if we don't exist as a company, it might be 30 years from now that all that exists. Naturally. But what if we can make it exist in ten years? You know, how much can the benefit how much can the world benefit in the 20 year gap that happened there? And these are the types of dynamic range thoughts that I love to think about in the leadership, in Deepgram, but also just across the company for folks to like. You have your day to day of you're trying to achieve a certain goal, get a certain model out, get a certain product out, help certain customers get going, et cetera. You have to exist as a company. And not only that, you learn from your customers in this process, et cetera, but the whole time, you should have a background process running saying, okay, in the next six months, we're going to throw all of this away, and we're going to invent a totally new architecture for how this will work, and it will work the way we want it to work, not just are able to make it work right now. You have to constantly be reinventing and disrupting yourself and innovating in the company.
Demetrios:
Yeah, it's almost like the idea that came to my mind was thinking about what it would be like or what are almost like guarantees in your mind in 30 years. But then can you compress that down and do the whole accordion type thing and say, yeah, instead of 30 years, can we do it in five, or can we do it in six months? Can we get something that is just so unreal you would think it came from the future?
Scott Stephenson:
Yeah, yeah. And take, you know, take the that thought process, like, really seriously and say, you know, for instance, if you say, hey, we want to compress that down. Assume you have the same number of human hours to produce it, right? So you say, okay, over time, you organically, like, build a company, and it goes from, you know, ten people to 50 people to 100 people to 200 to 500 to 1000 to whatever. Okay, well, you need to compress the hiring of all of those people. That might be your first thought, right? Then you say, well, do we really need to compress the hiring of people? We're an intelligence company. We're in a new phase. We're in the intelligence revolution, actually. Machines can provide intelligence and humans can provide intelligence.
Scott Stephenson:
So we don't have to just use artisanal intelligence to build the company. We can use the machine intelligence to build the company as well. So could that be the thing that accelerates the timeline? And now if you're thinking that way, you back up in the system and say, okay, well, if we had the humans creating all the models and writing the documentation and doing all this, because this is what happens in companies, then what pieces of this are our biggest road blockers what portions of it can we automate? What portions of it can we insert machine intelligence in? What portions can we add, essentially like a machine learning exoskeleton to the people who are in those positions in the company? And is that the thing that's going to accelerate us? And is it going to accelerate us 10%, 50%? 2x 10x 100 x? What's it going to be? Right. And so if you but one of the most exciting things in the last decade that has been developed and especially the last two years, I think everybody in the world is starting to realize that you can have agents, you can have machine learning. I think a great way to think about this is it used to be that like 90 or, you know, 90%, maybe 60% to 90% of people in a company were not managers. Now everybody in a company is a manager. They're a manager of AI Systems. And that's just what the definition of an intelligence company is going to be.
Scott Stephenson:
So constantly thinking about these things and trying to reinvent your blockers and say, okay, these are a blocker. If we only use human intelligence to do it. Well, what if we don't use, only, only use human intelligence to tackle that problem? Okay, then we can come at it from a different angle. And again, I think it really helps to think in that 30 year time horizon. Well, if we don't, somebody else will, you know, so, so any kind of like, you know, momentum that you have as a company that says, well, we have a great hiring pipe, this is just one example, but, you know, like, we have a great hiring pipeline set up and we could just keep going that way, and we know that we would keep, you know, winning on that side for the next three years or whatever. Yeah, but three years in the, in AI timeline is like ten years in normal tech company timeline, you know, and if, if you're not building the machine intelligence acceleration into your company over those three years and you're just doing it the old way, then you will, you will just like be blindsided later on by a company that is doing it that way. So, this is just one example, but there, there are many in the company, in go to market and product building, in customer success and all sorts of areas.
Demetrios:
So. Exactly. There's so many different vectors that you could pull on and try and think, how can I do, like that fold time, or where you have the line that's going across the page and you say, yeah, it can actually touch the back if you just fold the page type thing I want to go into, because you're talking about machine intelligence and you're talking about how to basically level up the company. One thing that I find fascinating about that is machine intelligence is one thing, but at Deepgram, it's probably one of the most humane places that I've worked at. Like, the human is very much considered in the working environment. And so when did the cultural aspect start to become something that was so important to you?
Scott Stephenson:
We're a learning company, and if we cease to be a learning company, then kind of the spirit has died at Deepgram. And I'll explain to you what I mean by that. Our humans learn, and we're constantly learning. In order to have humans learning, constantly be learning. You have to afford the opportunity for that. You have to afford the opportunity for mistakes. A lot of times, mistakes teach us a lot. You have to set aside budget to go after problems that are maybe too big and hairy, and you have to dial them back and whatnot.
Scott Stephenson:
And then there are certain things where you have to be disciplined and think more from a hygiene perspective or something like, hey, it's great to have artists and scientists and things like this, but also we have projects and we have customers, and things need to end up on time. And so basically, you're kind of bookended and you're doing this, you're doing this dance of working with your customers, having them help guide the product. And then they can, through providing value to them, they fund the development and the rest of the company, and then we reinvest that back in. But I think you just got to look at the def. I know. I was just talking about machine intelligence and human intelligence, and there's going to be a massive switch to a lot of intelligence being done by machines. But still, I believe that companies are, at least for the next 30 years. Beyond that, I'm not sure, but at least for the next 30 years, companies just look at the name of the company, literally the word companies, a group of people trying to accomplish a task that is still going to be the thing.
Scott Stephenson:
And again, index back to what I was saying before about everybody's a manager now. You know, that's still going to be the case that the novel, unique things in the world, humans are going to gravitate toward controlling, or that's where they're going to put their intellect into it. And so just like 200 years ago, a lot of folks might swing us a sledgehammer to lay down railroad tracks or something like that. But now we built machinery to do that. And then that increases the productivity of the world. Yes, it's true that there are people that were laying the railroad tracks then that immediately, if you just designed a machine that did that for them, you'd have an unemployment problem for that particular sector. But these things happen gradually, and then people retool, and also generations happen, and their kids learn about new things, et cetera. And then we all benefit from the productivity gain over time, on average.
Scott Stephenson:
I'm constantly thinking about that in Deepgram. How do we. So how do we, like, how do I myself in my daily job, how do I put myself out of work with intelligence, but then learn a new thing? How do I delegate to others? Because I'm constantly trying to find world class executors in Deepgram so that people in the company don't think Scott would be the best person for that job. No, like, I want me to be at least, for every individual piece of Deepgram, there should be at least a person that everybody's like, whoa, whoa, whoa. You don't want Scott doing that. You want the expert doing that. You want the expert doing these things. You want that.
Scott Stephenson:
And I think you want everybody in the company thinking that way, because then it propels you forward in this much faster arc. But I think also, when you employ highly intelligent people, they're very satisfied in that environment then, because it isn't like, hey, it's three years later and I'm still doing the same job. What could I, you know, what am I going to learn? What am I going to do with my life? Because you're talking about really highly intelligent people. Tons of horsepower. How do you keep them satisfied? Will you keep them learning? Going back to what I was saying, we're a learning company. Our machines learn, our humans learn. We're learning from our customers, our customers are learning from us, we're educating them, et cetera. And so we can't.
Scott Stephenson:
We can't ever lose that thread. But, yeah, hopefully that gives you an idea of, like, where I come from. But then how does the. How do you actually build a culture for that? I think we are lucky in that. Myself, Adam Sypniewski, our CTO, Noah, my other co founder, several other people in Deepgram, especially in the first cohort of ten or 20 people that worked at Deepgram, we all came from a very academic background, PhDs and postdocs and that kind of thing. And that type of environment. It is a very similar type of environment where learning is time for learning is afforded, crazy ventures are accepted, et cetera. But then you also need to deliver on timelines.
Scott Stephenson:
One thing that I think that is a little bit different about startups, though, is that in academia you have to spend like 75% percent of your time begging for money. And in startups, you know, it's like 10% or less. So you actually, the fraction of time that you get to spend on like pushing the frontier is way higher in startups compared to academia. I wish that would change for academia. There's like some structural things that have to happen there. I would, I would love for folks to not be saddled with that, but at least in startups, you don't have to do that. And so things can move very quickly. And especially in intelligence, you can see that now, like, it's hard to compete with the startups in machine intelligence.
Scott Stephenson:
If you're in academia, you basically have to focus on small datasets or novice small architectures or something like that, which are very valuable. But yeah, hopefully that gives you an idea. I would love to hear actually your thoughts on it, though, because you're in it too. I can say what I think about it.
Demetrios:
Well, from my perspective, you set the tone on the day that I joined and I was given a video that said, here's the CEO, here's what the company's about. Watch this video. And it was you eating like a ghost pepper and then trying to explain what the company is about and what we do and what the mission is. And it was almost like you, you saw an episode of hot ones and you were like, I'm going to do that to myself. I think that's what our onboarding video should be. Am I wrong there? Did you?
Scott Stephenson:
Pretty much, yeah, it was that and the same thing for customers, you know, try to get attention of the customer, you know, go to extremes, basically. Right. What are some extremes that are acceptable, right. And that are relatable, you know, but, but yeah. And then also showing that, like, I'm not, I'm willing to put myself in a stressful situation for Deepgram, you know, as hopefully I should be, you know, but also, you know, that is allowed at Deepgram to do some, to do some pretty, you know, out there things like we send that video to prospects, you know, we still send that video to prospects and, yeah, and, but it is one of those things that's memorable, you know, saying, you know, for folks thinking like, there's something off about that company, but I like it. Like, it's not the same. Just like, you know, cookie cutter, like down the fair way, you know, way to do things. Like, no, we have that kind of artist, scientist, academic, like bend to what we do.
Scott Stephenson:
Yeah.
Demetrios:
It immediately gave me permission to be myself. That's the feeling that I took from. It was like, wow, if that's what our CEO is doing, then I can do what I do and, like, I don't have to try and fit into some mold that I think is needed or the idea of the corporate whatever just went out the window. And so I really appreciated that as soon. And like I said, it was setting the tone from day one. The other piece that I'm absolutely in love with is it's remote first. And it has such a strong writing culture. And so you can always find docs.
Demetrios:
People are always asking for docs, referencing programs or referencing things. And that is such a blessing when you are remote for first culture, like the ability to not have to get on a call with someone when they need your help with things as the default. But as, like, once you've exhausted all the other possibilities for me is huge.
Scott Stephenson:
And then the fact that that enables information retrieval, especially through, like, new intelligence systems. You know, before it might have been a standard search, right? But now you can use rag type systems or, you know, embeddings and whatnot. And so, yes, we have large company, lots of documentation, many things going on. We record all of our internal meetings and everything as well. And so information is all over the place. But a lot of times you can still find what you're looking for because of the intelligence tools that we either adopt from others that are building them, like our customers or other companies that are out there in the world, or that we built ourselves internally in order to allow that. The one, one thing that I really, really hate, like there's a, you know, that I just can't shake. And, like, it's, it's something that I chase all the time, which is when you have a great idea, but you don't have a place to put it, or you don't have a place to see if other people have had that same idea or if you know, this is partially one of the reasons that we have so many slack channels in Deepgram as well, that, like, this, this is, this is a great idea.
Scott Stephenson:
We maybe should not do it right now. Okay. And I want everybody in Deepgram to be able to have this way of thinking, right? And then you put it in the ice box, you put it in the parking lot, you know, whatever, whatever analogy you want to think about, but don't lose it because those are like the little brilliant pieces that maybe it's like this moment is not the exact time to do it. But maybe a week from now, maybe a month from now, maybe two years from now, et cetera, is the time to enact that. And then you kind of, this is a stupid analogy, but the cicadas rise out of the earth 17 years later all at the same time or whatever. If you actually have those little pieces of information stored around, you have them documented, you have people aware that it was talked about. You have all of this going on, then when the moment is there, then you can have it all come up at once. For example, this is what happened with our TTS last year when we had lots of different threads going on, but we tried not to lose any of the information over time.
Scott Stephenson:
And then as soon as we're like, okay, the market is ripe now for TTS, let's go, then. That was what allowed us to release something very quickly with good quality.
Demetrios:
Well, Scott, this has been amazing. Thank you so much for coming on here. This is really cool to have you doing this and sharing your origin story all the way to how you build in the company, and it gave me a whole new perspective on many different things.
Scott Stephenson:
Well, yeah, thanks so much for having me. You know, we are this remote first culture, but also we have an office in the Bay area. We have an office in Ann Arbor, Michigan, near University of Michigan, and then we have an office in Texas, too, in Austin. And so we're always happy to meet great people in those physical locations or online as well. We have a community that people can join like a discord server and meet us, talk about using the service or just meet us in general. I'm pumped about the community that we're building there, and thanks for having me on.