AIMinds #041 | Mo Asady, CTO at Convirza
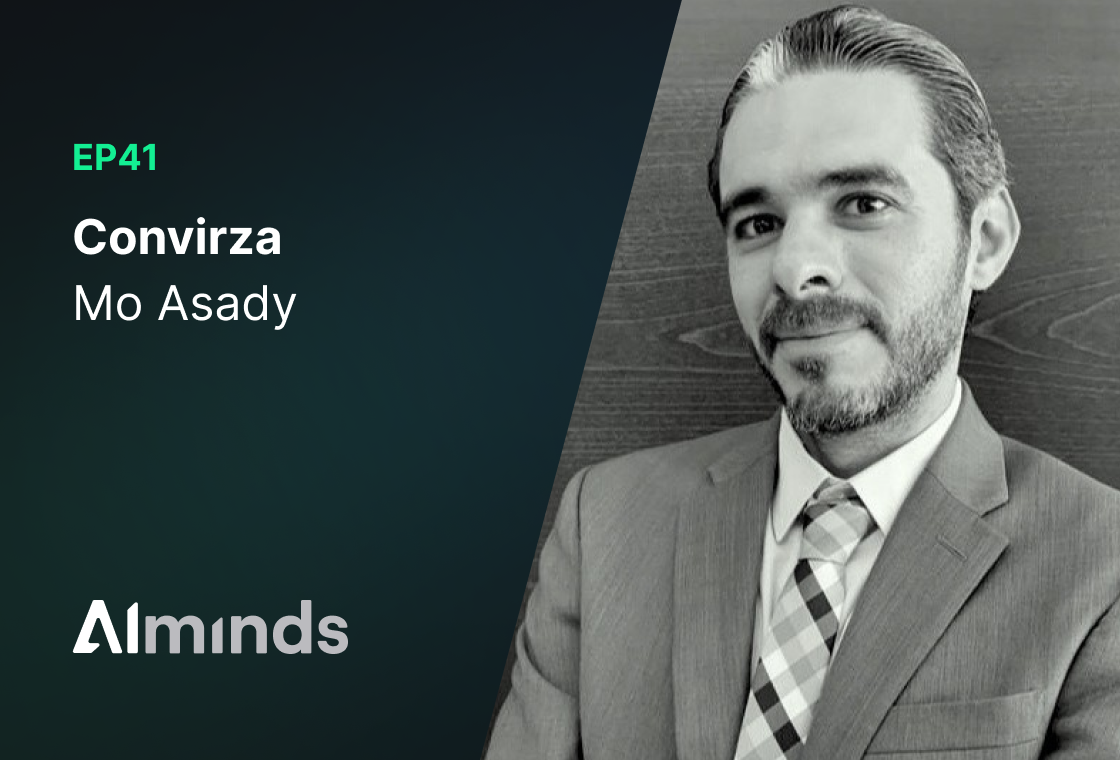
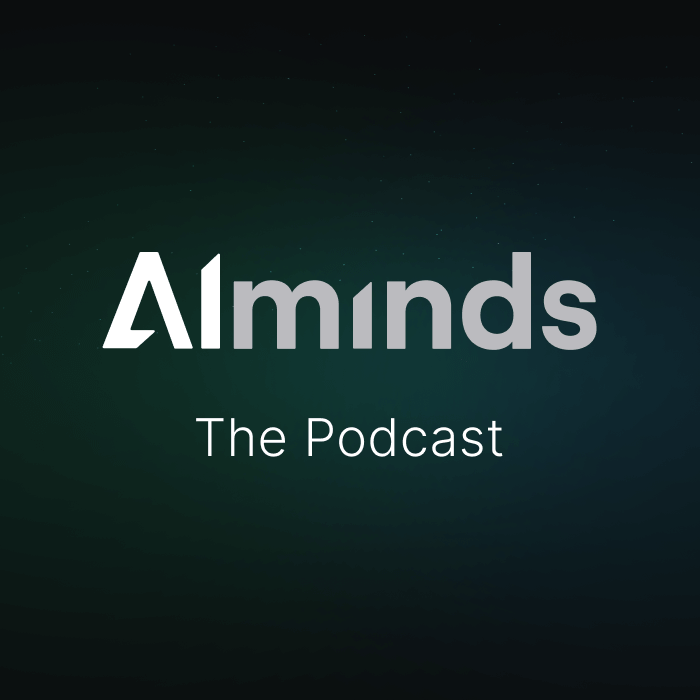
About this episode
Mo Asady has over 20 years IT experience. He has worked for companies including Microsoft Dynamics and FreightOn, in manager, VP and C-Suite roles.
Mo has demonstrated strong leadership skills, with technical experience from 3 continents, from mega corporations to startups. He currently is the CTO of Convirza, a Software company that helps businesses maximize revenue from phone calls.
Listen to the episode on Spotify, Apple Podcast, Podcast addicts, Castbox. You can also watch this episode on YouTube.
In this episode of AI Minds, Mo Asady, CTO of Convirza, shares his wide-ranging career in technology - from learning to code in the early ‘80s to his current work in conversational AI.
Mo recounts his experiences across the globe, including pivotal roles at Microsoft and innovative blockchain projects in Dubai aimed at streamlining logistics. He describes his work at Convirza, where he has guided the company through major changes, including the use of AI tools like Large Language Models (LLMs) to enhance customer call analysis. Mo also discusses how Convirza faced the rapid rise of technologies like ChatGPT, which initially threatened their business approach. By adopting smaller, purpose-driven LLMs, Convirza improved both their accuracy and efficiency while keeping costs in check.
This episode offers insights into the adaptability needed in today’s tech industry and explores how AI can reshape real-time customer communication in meaningful ways.
Fun Fact: The company Convirza, where Mo serves as CTO, started over two decades ago with the founder manually recording calls on tape in call centers to analyze customer service quality—a striking contrast to the highly automated, AI-driven service it offers today.
Show Notes:
00:00 Excited to discuss your diverse, global career.
06:09 Self-taught engineers built successful streaming company.
07:02 We enhance customer conversations, boosting revenue significantly.
12:12 Dynamic adapters enhance versatile LLM functionality efficiently.
14:03 Questions vary: yes/no, numeric, agent empathy.
17:26 Fast decisions prioritize short- and long-term goals.
20:25 Trained small model with data, improved accuracy.
25:01 Switched to Deepgram for cost savings, efficiency.
27:01 Mo's insightful Convirza evolution amid disruption.
More Quotes from Mo Asady:
Transcription:
Demetrios:
Welcome back to the AI Minds podcast. This is a podcast where we explore the companies of tomorrow Built AI first. I'm your host, Demetrios. And this episode, like every episode, is brought to you by Deepgram, the number one text to speech and speech to text API on the Internet today. Trusted by the world's top conversational AI leaders, enterprises and startups. Some you may have heard of like Spotify, Twilio, NASA and Citibank. And this episode is very special. We are with Mo, the CTO of Convirza.
Mo Asady:
How you doing today, man?
Demetrios:
I'm doing great, how are you?
Demetrios Brinkmann:
Yeah, I'm excited to chat with you because you have such a colorful background and you've been in so many different positions throughout your highly decorated career, I would say, and globally, you've lived in so many different areas. So the cultural aspects do not pass me. Me as an expat from the US now living in Europe, I can already tell you I'm probably going to resonate with a lot of what you talk about. But let's start with where did you first get your hands on the keyboard? You started as a developer and why is that?
Demetrios:
Well, it started actually way before that. First time I got my hands on a keyboard was at the age of 7, in the 1980s, so early 80s. Started writing code and by the time I got to university studying engineering, I was doing better than my tutors at that time. So it was really good. And it was basic back then. But then professionally my first job as a developer was in London where I worked for just a webshop where we were working with hotels and building online booking systems.
Demetrios Brinkmann:
Nice. And what year was that in the 90s?
Demetrios:
That was. No, in the 2000s. 2004 was when. When I did that gig.
Demetrios Brinkmann:
Okay. And I know you had a stint at Microsoft too. Can you talk to me about that?
Demetrios:
Yep. Yeah, I worked for Microsoft. I was a technical project manager working with their CRM offerings. So that was in Stockholm, Sweden, and worked on really, really large scale projects for, you know, managed teams of like 50, 60 engineers working on a single implementation.
Demetrios Brinkmann:
Wow. So there definitely needs to be some project management and product management when you have a team that large. How was it when you were there in Sweden, coming from the uk culturally, as I mentioned, Anything to point out.
Demetrios:
Amazing people. I love Sweden, actually. I hold a few passports because I've been all over the place and I have the luxury of applying for citizenship, but that's the passport I use when I travel just because everyone knows they're neutral and I love the Swedes.
Demetrios Brinkmann:
Yeah, you make friends as someone from Sweden. You spent some time in Dubai also though, what were you doing over there?
Demetrios:
Well, I went there to work on their logistics infrastructure. So DP World and the rta, which is basically the Ministry of Transportation of Dubai, were my customers and we were looking at the trucking industry and how we can uberize that industry. So blockchain was involved a lot of integrations to governmental systems and into the port system. So yeah, it was a very, very interesting project.
Demetrios Brinkmann:
Yeah. So blockchain, this must have been more recent.
Demetrios:
Yeah, yeah, this was right around the pandemic.
Demetrios Brinkmann:
Ah, fascinating. What are some of the challenges you were working on there? Because it seems like uberizing the trucking industry in Dubai, that is a very exciting problem. But what does that actually mean? And like what was your day to day like?
Demetrios:
So you're working with the government and for anyone who's worked with the government before, is slow and got a jumps through a few, a few hoops. But people and processes were the major challenges. I think the technology was mature and ready to go. Then it became harder with implementation. Right. When you take a working class of just truck drivers and you want to enforce technology, it wasn't an easy task. But again, your partner is the government, so they have the luxury of making it a loan.
Demetrios Brinkmann:
Yeah. And I have heard that the United Arab Emirates government, they make things very lucrative for people or there are incentives. They have lucrative incentives, I guess you could say.
Demetrios:
Yep. So it's tax free, but then they have fees. So I mean, you know where I'm going.
Demetrios Brinkmann:
Yeah. Now I know you also spent some time working on streaming and specifically video streaming. What was that?
Demetrios:
So that was, I mean think of your Netflix, your Hulu's, any, any kind of live streaming service and even on demand that you see today.
Demetrios Brinkmann:
The.
Demetrios:
This was a gig in really late 90s, early 2000s and there wasn't any place for you to get an education on live streaming and different video formats and encoding and transcoding. So really self taught with a bunch of really, really smart engineers sitting in a small data center in Malmo, Sweden, building the data center from the ground up, stalling OS and figuring out how to do it. But then it scaled to multimillion viewer service and it was eventually sold. And that was my exit. But I was one of founders of the company. Oh, fascinating.
Demetrios Brinkmann:
That's so cool. So now you're in Florida and you're working with Voice. Talk to me about what you are doing.
Demetrios:
Well, in a nutshell, we help Our customers have meaningful conversations with their customers. That's what it's all about. So I'll give you a little bit of history about Convirza and where we started and what got me here. The company is over 20 years old and it literally started with a tape recorder and a founder that was walking into call centers, putting the tape recorder there to plug it into the analog telephony system, recording these calls, going back, getting a piece of paper and a pen, scoring them manually and going back to the managers and saying, your employees are not doing X and Y and as a result, you're losing money. Wow. So that's where it started. And then with technology, we, we started writing software and this was before my time at the company and built custom models that are trained against tens of thousands of calls to provide these insights on a dashboard. So what we've noticed is our customers increase revenue anywhere from 20 to 30% simply by converting more.
Demetrios:
Hence the name Convirza. Conversations Convert.
Demetrios Brinkmann:
I was thinking it was from the Spanish. Convirza is conversation. But you're converting your conversa that Convirza.
Demetrios:
I get it. Convirza.
Demetrios Brinkmann:
So what an evolution when it comes to being completely analog to then going digital and training your own models. And now you're not in that realm at all.
Demetrios:
No, no. The world changed about 18 months ago. There's this little thing called chatgpt that.
Demetrios Brinkmann:
Shook things up, I imagine.
Demetrios:
It did, it did. So the initial reaction is we're sitting in a qbr, all the executives and everyone is aware of it. And they go, what do we do?
Demetrios Brinkmann:
Because you thought this was business threatening, this was going to end the company 100% at first.
Demetrios:
That was, that was the knee jerk reaction. Like, this is not good. Everybody's going to be able to do what we do by writing a few lines of codes. And man, I was wrong. I was wrong. And here's why. You cannot take a generic LLM and have it answer business specific questions. So what we notice when we start using the LLM out of the box is a tire shop, right? A 250 location tire shop.
Demetrios:
Company has very specific needs. We don't like to call it a script, we like to call it a scorecard. So imagine a set of questions. Where did the agent do this? And they use the customer name three times on the call. As a good example, what we've noticed is if you use it exactly three times, no more, no less, your chances of conversion go up by 20%. The data tells that story. Now historically, we were, we were, we Were actually collecting somewhere around 20 to 40 data points that were pre programmed and that's all we had. And then with LLMs and by using our existing data, we've analyzed over a billion calls over the years.
Demetrios:
So we have a lot of data. Obviously a lot of it is anonymized so that some of it is customer specific and then some of it is industry specific. That's where we anonymize the customers just for data privacy reasons. But we use that to train LLMs. So what we did is we took a smaller LLM, an open source, smaller LLM, very, very light, like an 8B.
Demetrios Brinkmann:
Or an 8B or a 70B. An 8B.
Demetrios:
How small? An 8.
Demetrios Brinkmann:
Okay, yeah, real small, real small.
Demetrios:
But we gave it a lot of examples and we said you have one purpose in life and your purpose is to answer this one question. And it outperformed the best models out there. So outperforms ChatGPT 4.0 both in terms of accuracy, pricing, scalability, response time, all of it. You name it.
Demetrios Brinkmann:
And that one question that its purpose in life is for is, did the human agent say the name three times or is it, did the human agent go through these 40 checklists?
Demetrios:
No, it's a single question. In this case it could be did the human agent use the name three times? And then we partner up with this amazing company that has, the way they build technology is you have the base LLM, let's call it llama, let's call it whatever is sitting right there. And then they build a bunch of adapters on top of the base LLM and they're offering to us is an infinite number of adapters so we can ask as many small questions as we want. It's not going to affect the performance. And what their system does is depending on the question we ask, it changes the adapter. It only uses the base LLM and give you that one answer you want in milliseconds.
Demetrios Brinkmann:
It's almost like they're interchanging loras on top of the llama model or whatever the small model is. And then you don't have to worry about do I need to fine tune 40 different models here and then have some kind of routing system going to each one when the question comes in, did you do X, Y, Z? Okay, well this is going to now this fine tuned lawn model. It's, it's a little bit of an abstraction on top of that where it can interchange those adapters. That's a really cool way of looking at it.
Demetrios:
Yeah.
Demetrios Brinkmann:
Okay. And I imagine you are having the calls be transcribed first. Then they're going into that system and you're getting the adapter. And then off the back, it's scoring it. Is that kind of how it comes out? Is it just yes, no score?
Demetrios:
No, it's way more than that. It's depending on the type of questions. Some of them are simple, yes, no questions. But then we have those that would return a numeric range, say, for example, lead quality. So we not only listen to the agent on the call, we also listen to the caller, and we're able to say, this lead has a lead quality or a lead score of 90 out of 100, which means they're closer to conversion than a lead that has a 30% score. So that's a type of a numeric one. The third type is really cool, and I think it's a differentiator in the market. When you ask a question and go, did the agent really care about the customer on this call? And that's super.
Demetrios:
That's a tricky question. Not only can it return a numeric value and say, Yep, they cared 70%, it actually comes back with why, Reasoning of why it gave that score and what it recommends in the future for the agent to hit a hundred percent, what they missed on the call, what they could learn from that one call.
Demetrios Brinkmann:
Okay, so this is fascinating. Is it doing any sentiment analysis on the tone or is it just the words that are being used?
Demetrios:
Oh, definitely. Both of them. Both of them. So we actually use Deepgram for the sentiment analysis, and we rely on that both on the sentence level, speaker level, and the overall conversation level. That is one of the data points that we feed to the models to then make these determinations.
Demetrios Brinkmann:
Okay, so you've got this analyst happening on so many different levels. You recognize that fairly quickly, I imagine, when you were in this point of despair, thinking that the business had one foot in the grave and one foot on a crutch, and then you decided, let's see if we can incorporate this AI into the product, and let's see what happens. Can you talk me through the product development phase and how you went about trying to upgrade your offering?
Demetrios:
Well, yeah, it was. It was an interesting. An interesting problem, an interesting challenge, to say the least.
Demetrios Brinkmann:
So take me back to the time where you were in the depths of despair, almost seeing that ChatGPT came out and you thought the business was taking a nosedive, and now you just explained eloquently where you've gotten to. There's been a road to get there. And I know what it looks like now, what it, what did it look like before? And how did you bridge that gap to infuse a new AI product into the business?
Demetrios:
Yeah, that's a very good question. So the world was and is still moving very fast. So what we had to do is really make a decision and go, here's where we see things going, set a goal and then as any business, we have to worry about our short term goals and achieve them. The long term goals are there and they're great. You have to be innovating, you have to always stay updated. You don't lose your edge in the market. That's great from a long term perspective, but from a short term perspective, these customers, you know, the 70, 75,000 rooftops that we serve are sitting there and going, what are you doing about it? How is, how are you going to change the way I run my business? Because again, these decisions that they make based on our data translate to money at the end of the day. So it's all about revenue, it's all about conversion rates.
Demetrios:
So we made the decision that it's time for us to start experimenting with LLMs and see how it goes. So my AI team picked it up and we started measuring the performance of different models, both closed source and open source, comparing the results to. I think the biggest advantage is we've had so much manually scored data just sitting there and we've collected it over the years, so we're able to validate the performance of an LLM pretty quickly. Yes, these are really expensive to produce, but hey, we've spread the cost over many, many years and we have hundreds of thousands, if not million of data just sitting there.
Demetrios Brinkmann:
Wow.
Demetrios:
So we started comparing the out of the box performance of LLMs to our manually scored data and sure enough, it wasn't great because again, you have, we serve basically every single industry, so you can't compare calls to a dentist to calls that you make to buy new tires. They're very different in nature. The objective is different. With one, you're price shopping, but with the second, you're probably just looking for an emergency appointment because you just want to go in.
Demetrios Brinkmann:
The nature of those calls are completely different.
Demetrios:
Yeah. And even, even the language, different accents, different. Even different words that are used on different types of conversations. Right. Imagine a model that needs to know what a Camry is. That's a Toyota. Right. The model needs to know that it's a Toyota, et cetera, even the transcription provider in this case.
Demetrios:
So yeah, we quickly understood that, realized that out of the box, it's just not going to work. So we flipped the equation around and we went, we have all this data laying around. Let's try to take a very small model, 8 billion open source LLM, train it against the data and measure the outcome. And sure enough, it was always in the 90% + percentile of accuracy just because we had that data laying around. The next step was building a feedback loop where you go, how can we keep it improving? And how can we accommodate for data drift and all of these things that could happen? And it was really just building a way to capture where the model made a mistake, feed it back, and we continuously see these models improving in performance.
Demetrios Brinkmann:
Another area that I am really interested in is how you rolled out that feature. What did that look like? Because you had something that was working and I'm sure people did not want it to go, or if it goes, it better be much better. And so what did the rollout look like? Were you just grabbing a segment of users, testing it and then coming back and trying to reiterate, or was it something that you recognize? This is powerful. Let's just put it out there as a beta and see who uses it.
Demetrios:
Yeah, it was, it was a, it was a gamble. So we have, we have 40 indicators. Like I told you those, that's what we sold is 40 questions, expect 40 answers. That's what you're going to see. We released this ultimate AI as a beta and it basically allows you to ask any question you want in pretext. So you, you ask the question, it'll go through all of your conversations and measure based based on the output for that, for that question. It went out as beta. One customer grabbed it, they liked it, the next customer did.
Demetrios:
And I was very pleasantly surprised to know that that's the only thing my sales team is using today. They actually forgot about the existing 40 indicators and they're like, no, this is all we're going to use moving forward. And we see a lot of customers moving to it.
Demetrios Brinkmann:
So the fascinating thing that I want to end on is traditionally, usage was a very good thing. When you had customers using your product, that means that you're having success. I've heard other founders say these days, usage means I have to be very watchful of my costs under the hood, since you're now looking at usage from the transcription, but you're also looking at usage from an API call. If you are just hitting OpenAI in your case, you're not. And I know you specifically went for a smaller model because I imagine it's faster and it's more accurate, as you said, but also very cost effective. Have you had those conversations of how you're going to ideally be the most cost effective as possible?
Demetrios:
Of course, that's. That's always on, on my KPI sheet, right when. When I report to the board. So the ways to even start looking at that is to go, well, who's involved? What are the pieces? And at the foundation of what we do, everything starts with a phone call. So it's voice that needs to be transcribed to text. So it starts by looking at that vendor and go, what can we do here? Who should we be working with? And what kind of savings can we look for based on volume? So it started right there. And obviously we are with Deepgram and we have been for the past year now. We were with a different vendor before that.
Demetrios:
And we did our homework. We compared just Qualities, we compared it to the known names in the industry, we compared it even to Whisper, and we came to the conclusion that Qualities is in par with expectations. And there was a significant saving in cost by switching to Deepgram. And we made that decision at the end of last year. So that was the first piece. The second piece, just like you mentioned, is using the smaller LLMs, finding the right partners, having the burst capacity to handle our load because you don't want to be behind in processing during peak hours. And when it comes to telephony, peak hours are known as 10am Eastern to 2pm Eastern. That's where the majority of the phone calls take place.
Demetrios:
And we need to be able to scale to, you know, 20 or 30 GPUs running at during peak hours down to a couple at night where there is lower volume while not paying for 30 GPUs, as you know, continuously because they're reserved. So we figured that out. Smaller, smaller models, very dedicated, lower adapters, just like you mentioned, sitting on top of the model and having a system that allows us to switch them on the fly and respond in milliseconds.
Demetrios Brinkmann:
Yeah, the spot instances are crucial in that case because I can see how 30 GPUs could get out of hand. That would be a very costly mistake if you're like, ooh, we forgot to turn those off.
Demetrios:
Yeah, A one hundreds are not cheap. In fact, you cannot even find them nowadays.
Demetrios Brinkmann:
No, it is wild. Well, Mo, this has been great. I really appreciate you coming on here and explaining everything to us about what you all are doing at Convirza. And also this evolution that you've had. It's not every day that I get to talk to someone in your position that has gone through such a disruption but has found the silver lining in it and been able to use that as a benefit as opposed to just moping in how the world's changing. Because, as we know, the world's always changing.
Demetrios:
I appreciate it. I appreciate it. This was great. Thanks for having me. And I look forward to seeing you again.