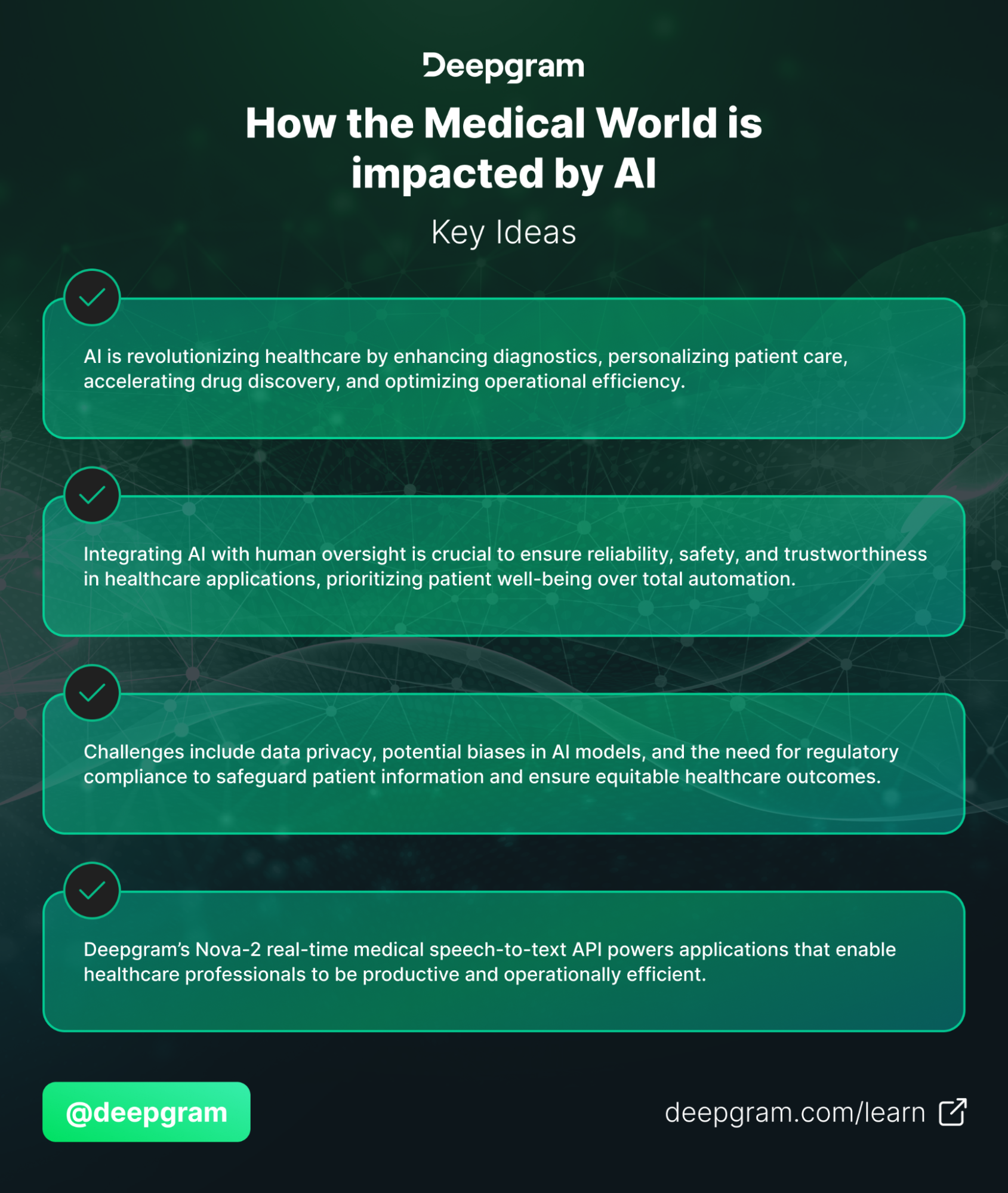
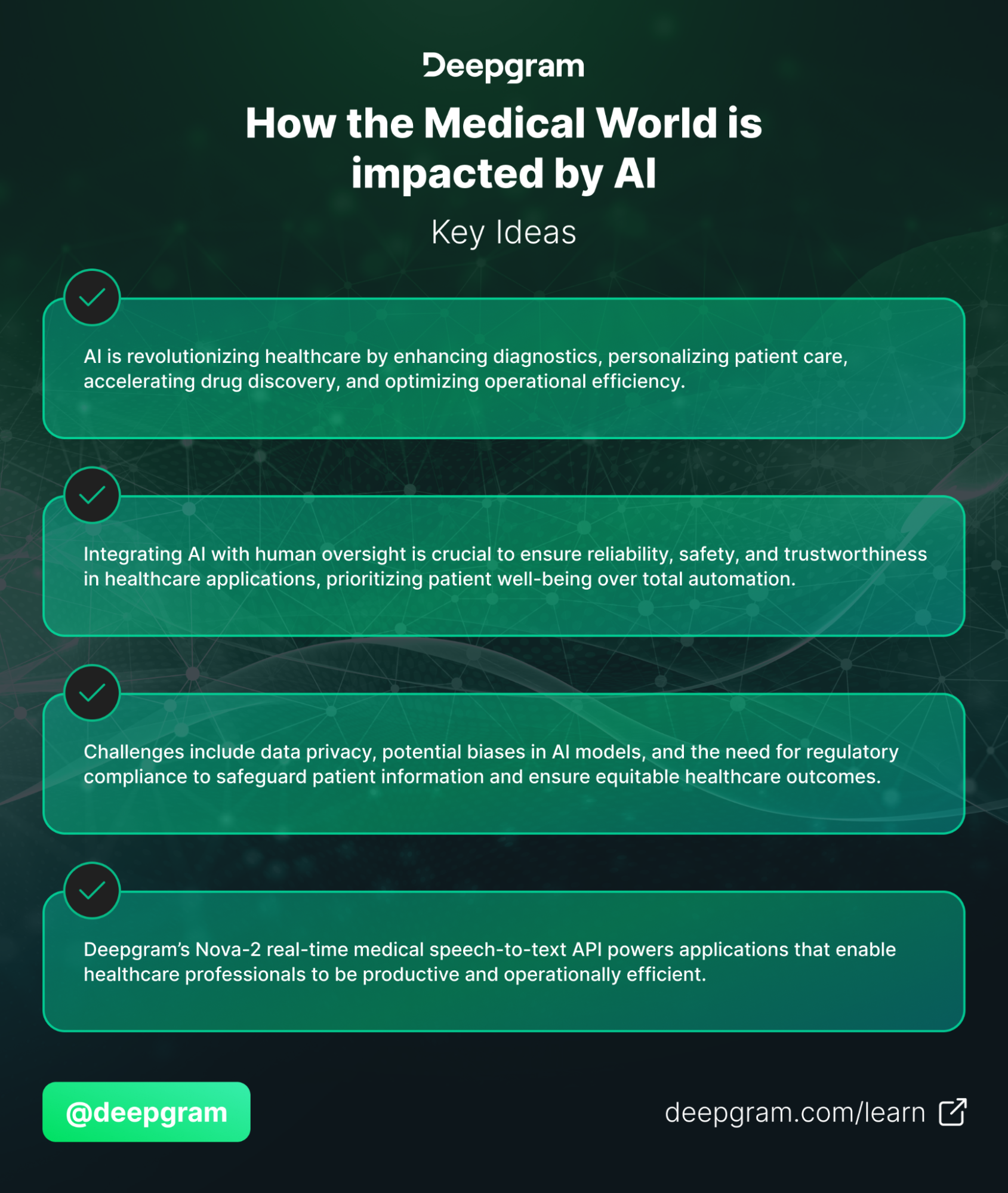
Medicine is a complex system that involves institutions, research, and multidisciplinary teams, including doctors, nurses, pharmacists, therapists, and social workers. These teams work together to maintain and improve public health.
Despite advancements in medical technology, healthcare professionals often face challenges such as stress, miscommunication, staffing issues, and time constraints. These challenges can lead to errors, increasing patient and government costs.
Artificial Intelligence (AI) is playing a growing role in healthcare by reducing costs and improving the quality of care. AI achieves this by following expert guidelines and transforming various aspects of healthcare, including diagnostics, treatment recommendations, drug discovery, and operational efficiency.
This article will explore how AI has improved medicine and healthcare and discuss the challenges and ethical considerations associated with AI in this field.
Use of AI in Healthcare
In 2016, AI pioneer Geoffrey Hinton boldly predicted: "We should stop training radiologists now...within five years, deep learning is going to do better than radiologists."
While Hinton's statement highlighted the potential of AI in medical imaging, it sparked a broader conversation about the role of automation in healthcare.
Eight years later, radiologists continue to play a crucial role, working alongside AI to improve diagnostic accuracy and patient care.
AI has sparked optimism about automating various aspects of our lives. However, healthcare is a sensitive field where the cost of an AI error can be high.
Experts like Ben Shneiderman and Fei-Fei Li advocate for AI that augments and empowers humans rather than replacing them. This balanced approach combines human control with automation to improve the medical field.
With this perspective in mind, we have categorized the use cases of AI in healthcare into the following areas:
Patient-focused: AI tools and platforms designed to directly benefit patients, such as personalized treatment plans, remote monitoring, and virtual assistants.
Clinician-focused: AI solutions that support healthcare professionals, including diagnostic aids, decision support systems, and administrative tools.
Administrative and Operational-focused: AI applications that aim to improve the efficiency and effectiveness of healthcare operations, such as resource allocation, appointment scheduling, and claims processing.
Let’s explore each category in subsequent sections.
Patient-focused Applications
Patient-centric AI allows healthcare organizations to provide customized medical care. These technologies actively involve patients in their medical care by prioritizing patient needs, preferences, and well-being. Below are some of the tasks they assist with.
Personalized Care
Healthcare organizations can use AI-powered medical assistants to provide more contextual answers to patient questions, manage appointment scheduling, and send reminders. Patients can use voice or text to access these services.
On the treatment side, companies like Oracle power the healthcare analytics at the UK’s National Health Service organization to identify cost-effective treatments and save millions of pounds annually, among other things.
Another example is TORTUS AI. The platform uses Deepgram’s Nova-2 speech-to-text Medical Model to generate consultation transcripts for healthcare practitioners.
That way, they can control Electronic Health Record (EHR) systems and perform downstream actions like ordering tests for patients tailored to their consultation results, scheduling appointments, and more.
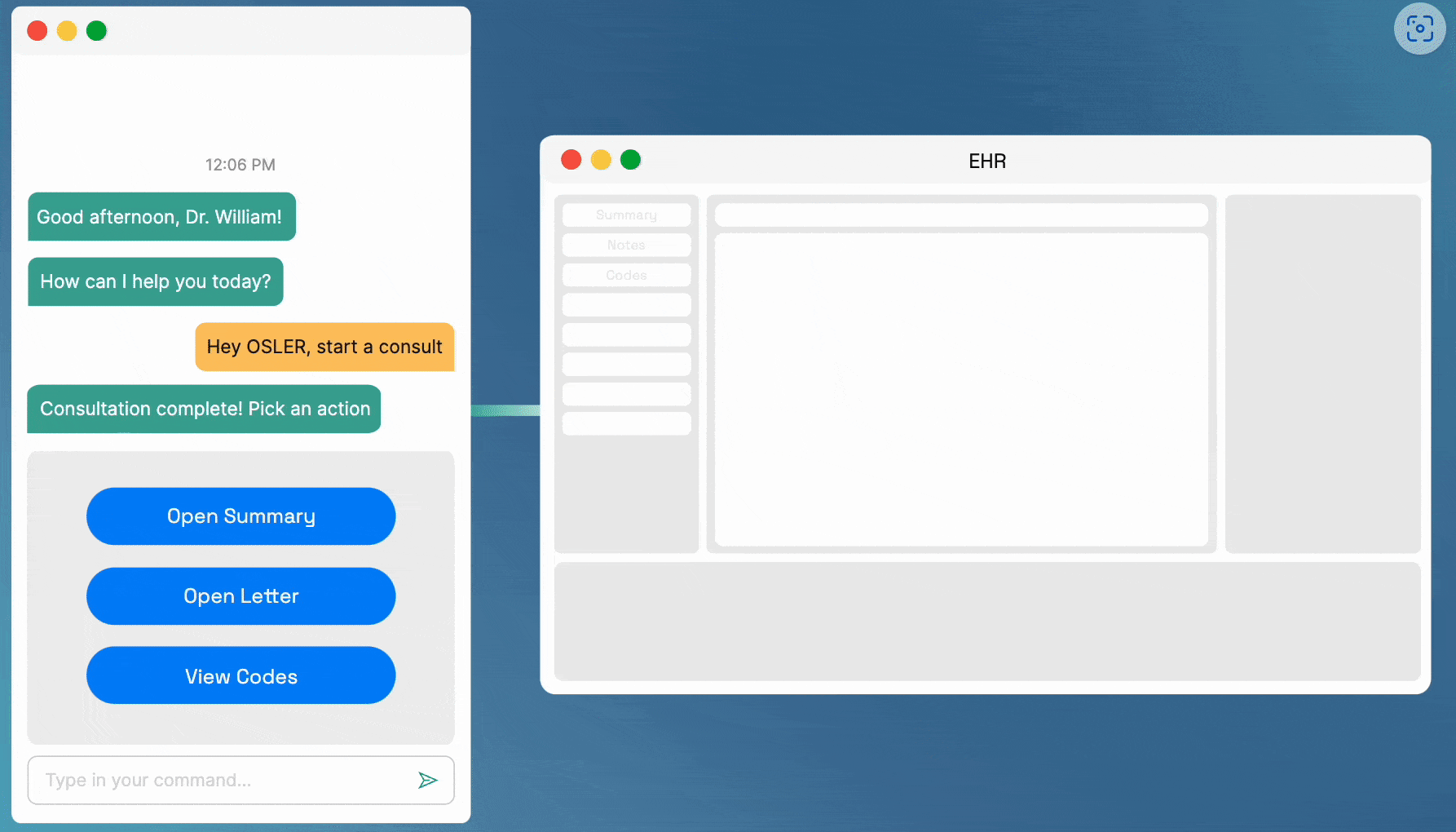
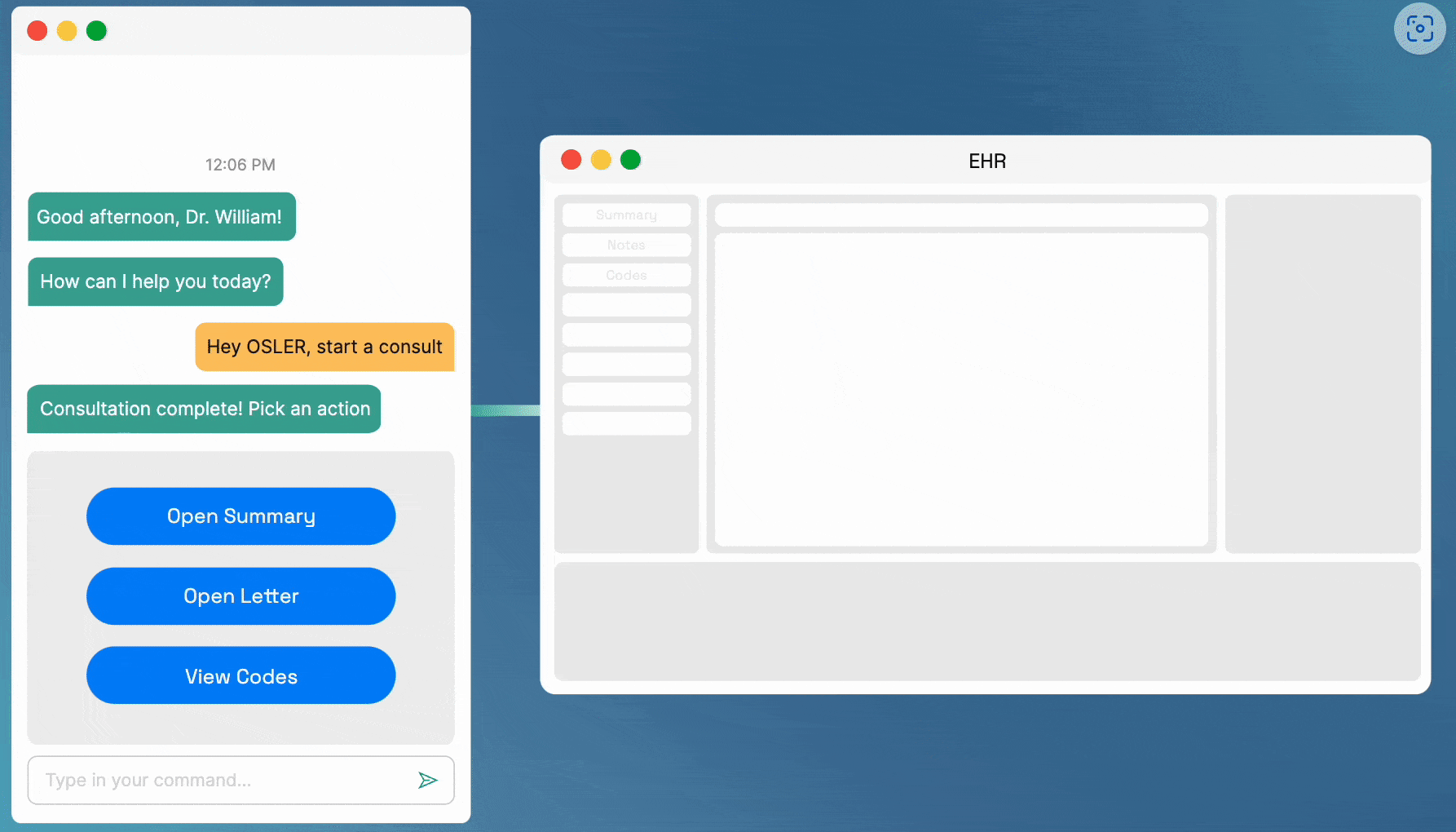
Remote Monitoring
With IoT (wearables and sensors), healthcare devices, cloud computing, and AI, medical professionals can monitor the vitals of patients at risk of different diseases and track their health over time.
They can understand a patient's disease stage by aggregating data and applying modeling algorithms like clustering. This information enables healthcare professionals to provide personalized care and facilitates remote patient health data monitoring, supporting continuous patient care and complementing telehealth services.
Case study
Vironix Health combines medical personnel and AI to monitor health data for people with chronic conditions like diabetes and heart failure. Their services assist hospitals in tracking patients after discharge.
Their algorithms and health scores help identify patients at risk of declining health at home. They work with health professionals to set up monitoring tools at the patient's home or environment. These tools aggregate data and provide insights into the patient's health status.
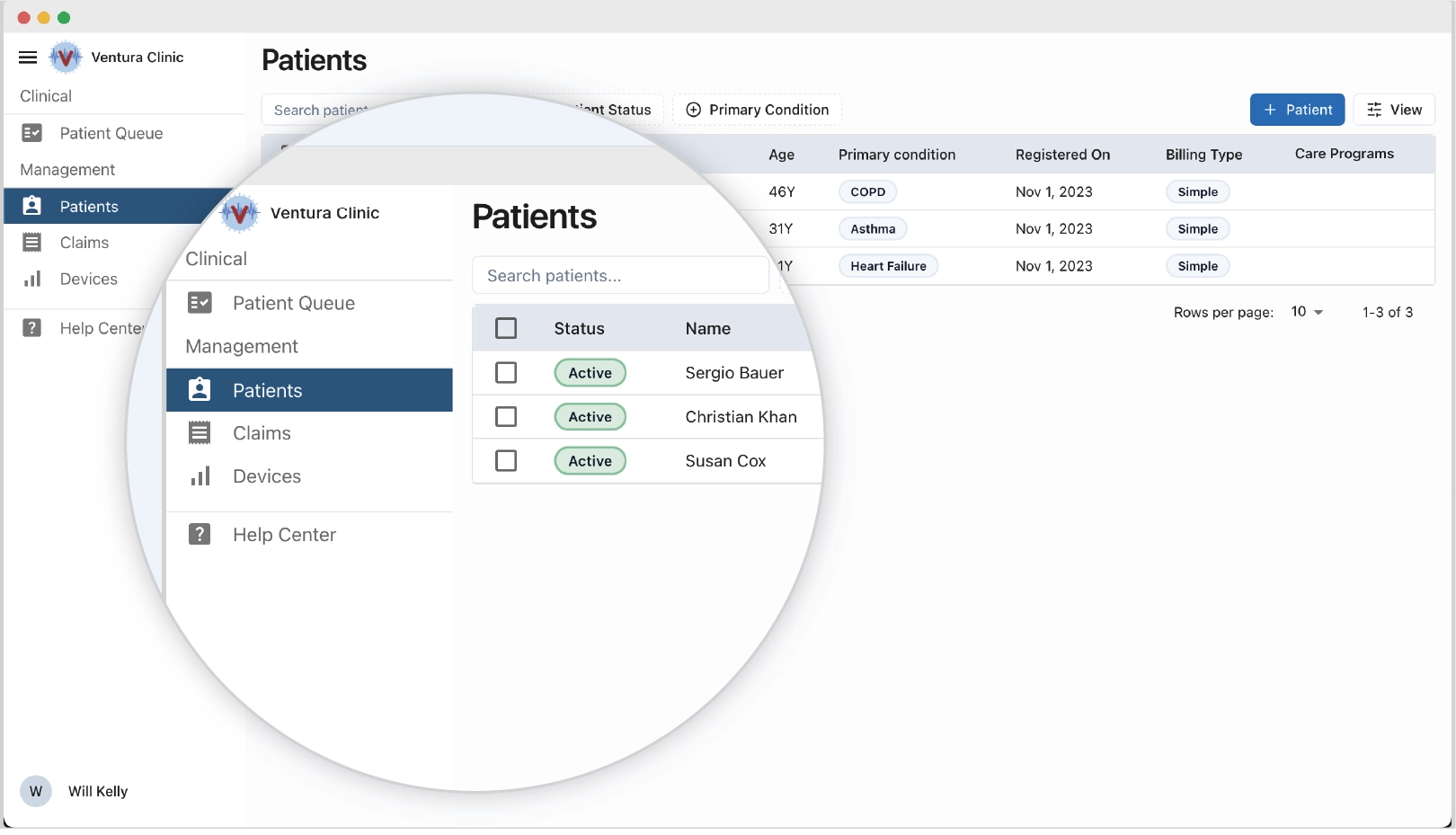
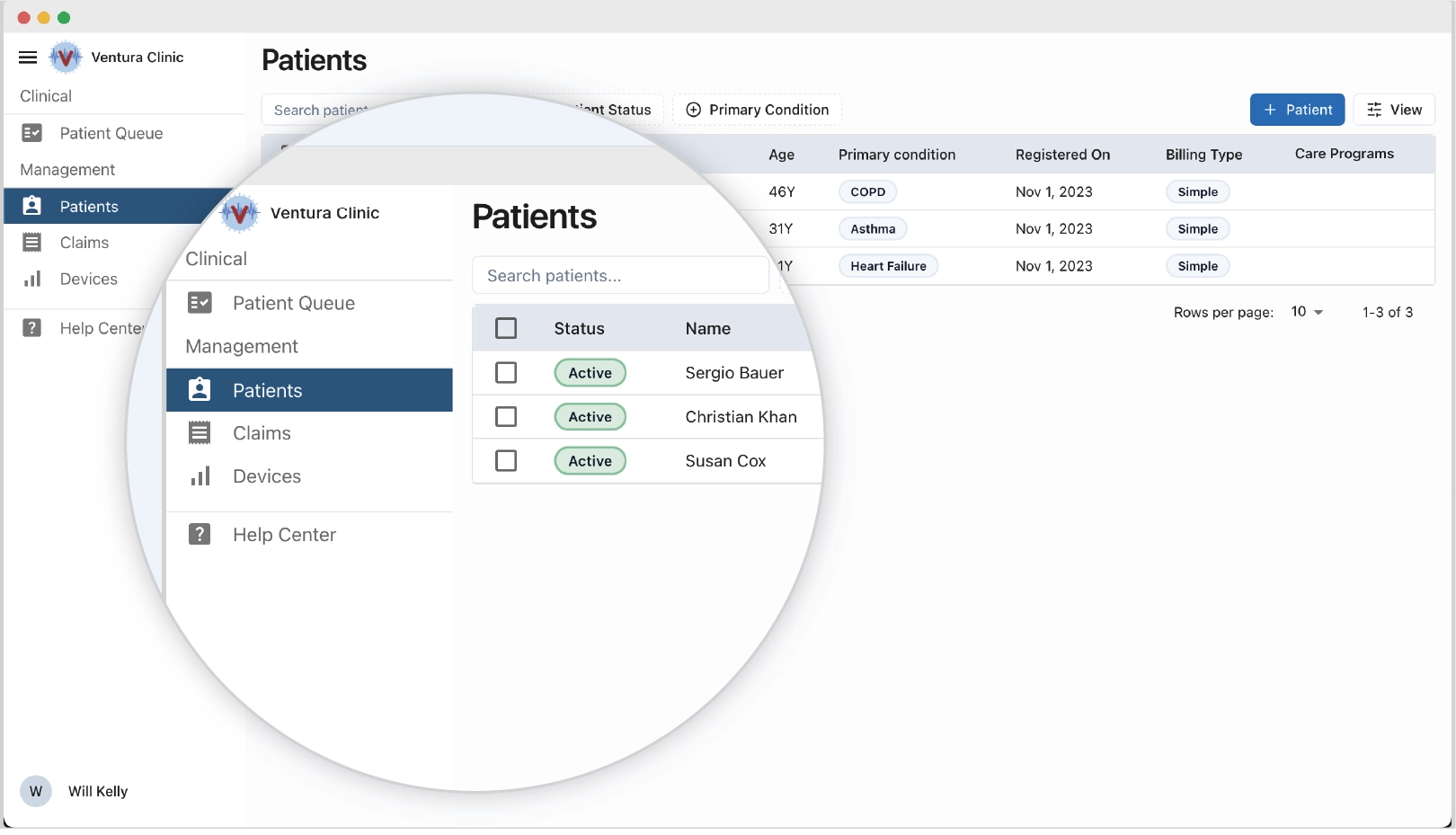
Clinician-focused Applications
Research by Ortega et al. involving 1,373 U.S. physicians from 2017 to 2021 showed that burnout increased from 44.4% to 50.4%. This trend potentially threatens the U.S. healthcare system's ability to care for patients.
This shows the need for solutions to reduce burnout and manage the workload of healthcare professionals.
AI-powered Diagnostics and Clinical Decision Support
Every healthcare institution has a workflow for managing various situations and collaborating with healthcare professionals and their patients. This creates an opportunity to use AI to support clinical decisions and reduce the cognitive load on clinicians.
Some hospitals have a clinical decision support system (CDSS), a tool that provides clinicians with alerts, reminders, and evidence-based clinical guidelines that improve patients' health and make healthcare more efficient.
Other hospitals may have separate tools to help clinicians with their medical and operational duties while giving care.
Generally, they are used for preventative care, helping monitor an inpatient’s health status or detect early signs of diseases before they progress. Medical imaging research is well established in this area, with foundation models (e.g., LLaVA-Med or Med-Clip, etc.) used to analyze X-rays, MRIs, CT scans, and eye scans.
To a large extent, these models accurately detect abnormalities, helping radiologists diagnose conditions such as cancer, fractures, and neurological disorders. This can reduce the time it takes to read scans and quickly help radiologists understand what they see.
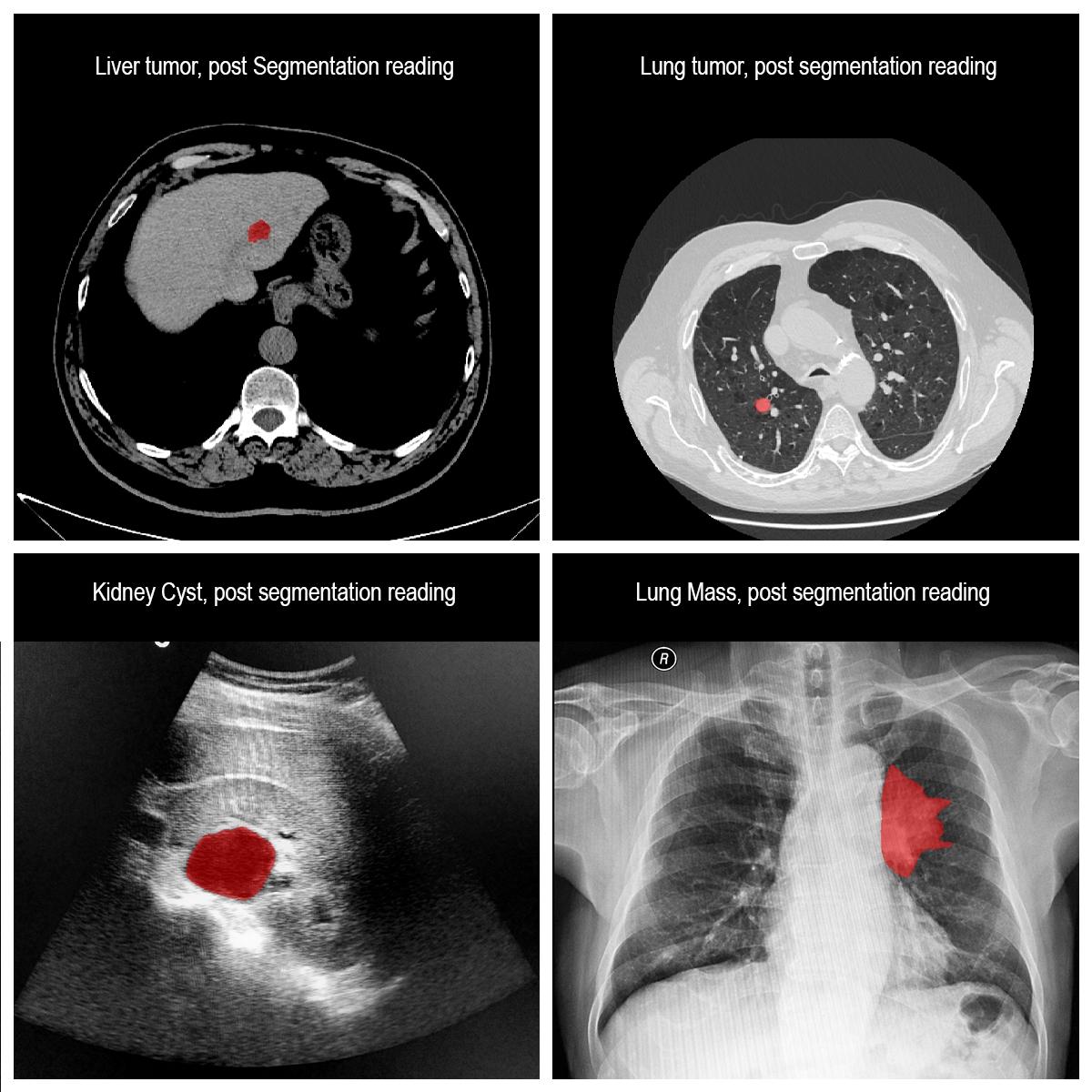
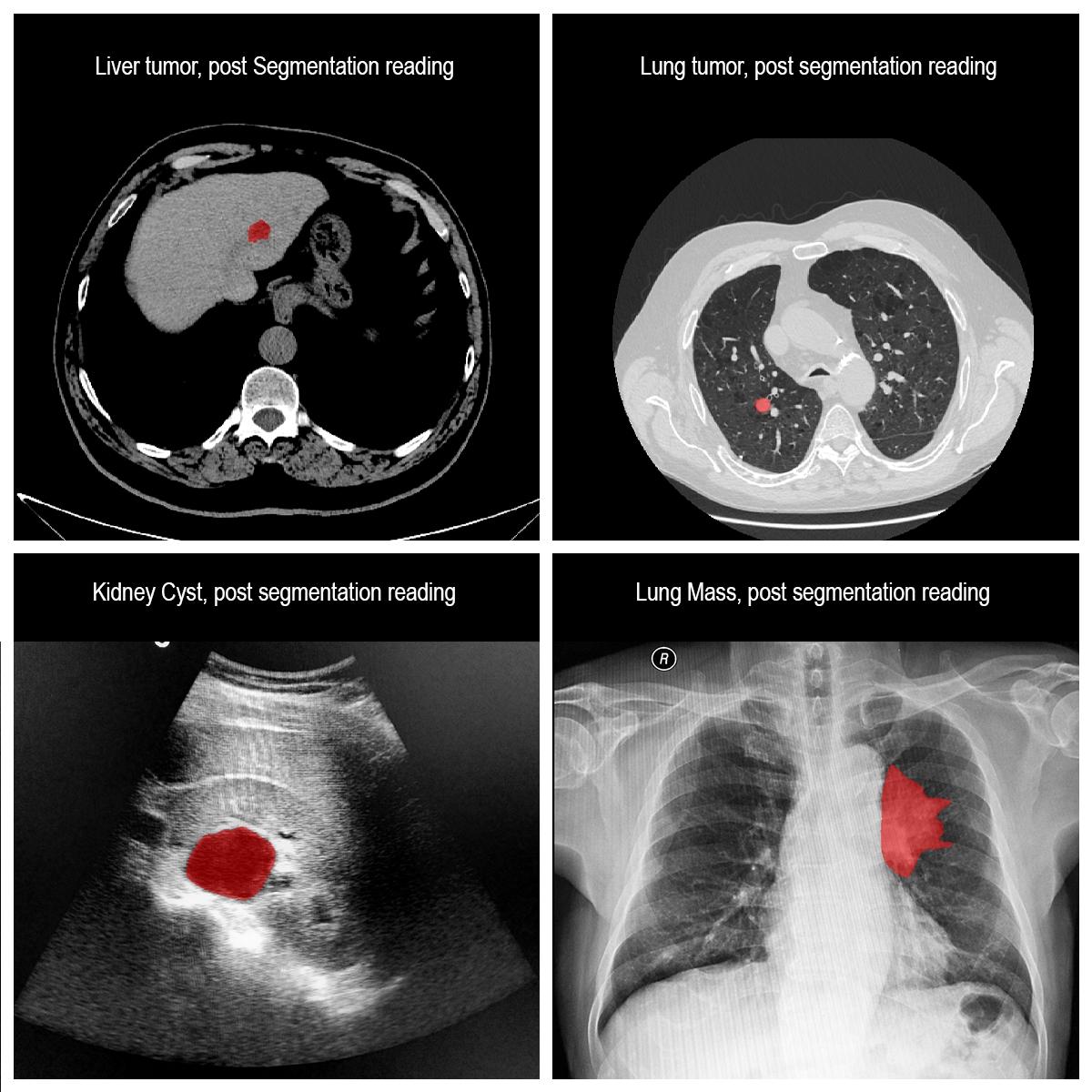
AI is also used in clinical decision-support systems to ensure patients have the best outcomes, including accurate diagnoses and treatment plans, timely alerts and reminders, and evidence-based interventions.
Case study
H2O.ai and Kaiser Permanente collaborated to improve intensive care unit (ICU) patient outcomes by developing an alert monitoring system. This system predicts when patient deterioration might occur, requiring an unplanned ICU transfer.
Kaiser uses four decades of electronic medical records, including lab results, vital signs, demographics (age, gender, etc.), comorbidities (presence of additional disorders), and bed transfer history, to predict the likelihood of a sudden crash or mortality in the hospital. The system can detect deterioration up to 12 hours in advance.
The system analyzes risks to generate a score that provides warnings, continuous monitoring and warning, dissemination, and communication of scope to medical professionals, which leads to an intervention.
The system can alert physicians or nurses immediately if the probability of deterioration exceeds a predetermined threshold, allowing them to intervene promptly.
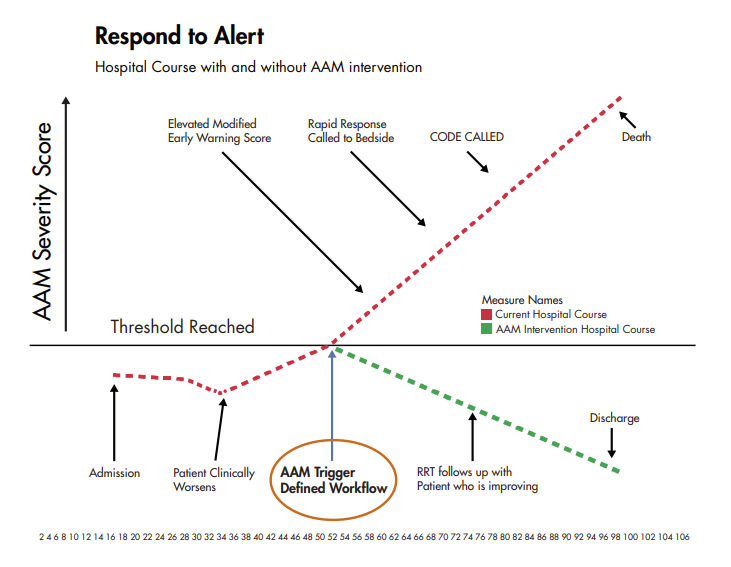
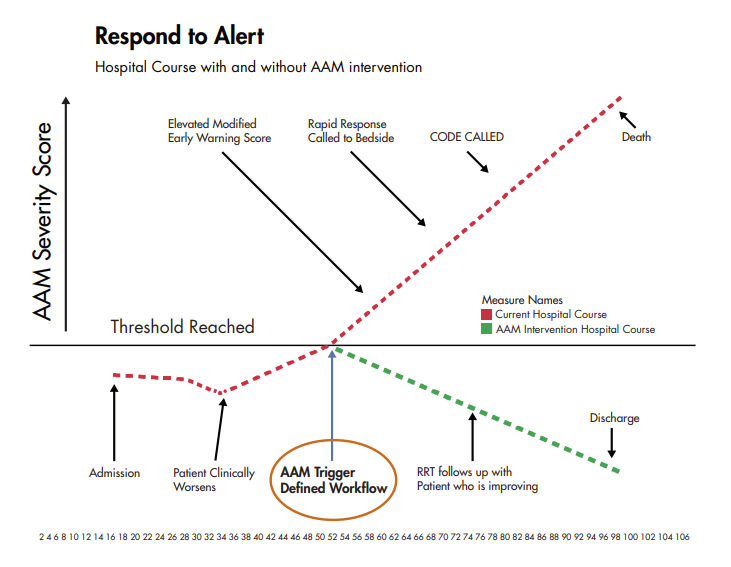
Researchers from Google published a paper, "Using generative AI to investigate medical imagery models and datasets," in The Lancet eBioMedicine. They explored using generative models to improve our understanding of medical imaging ML models. Check out the report.
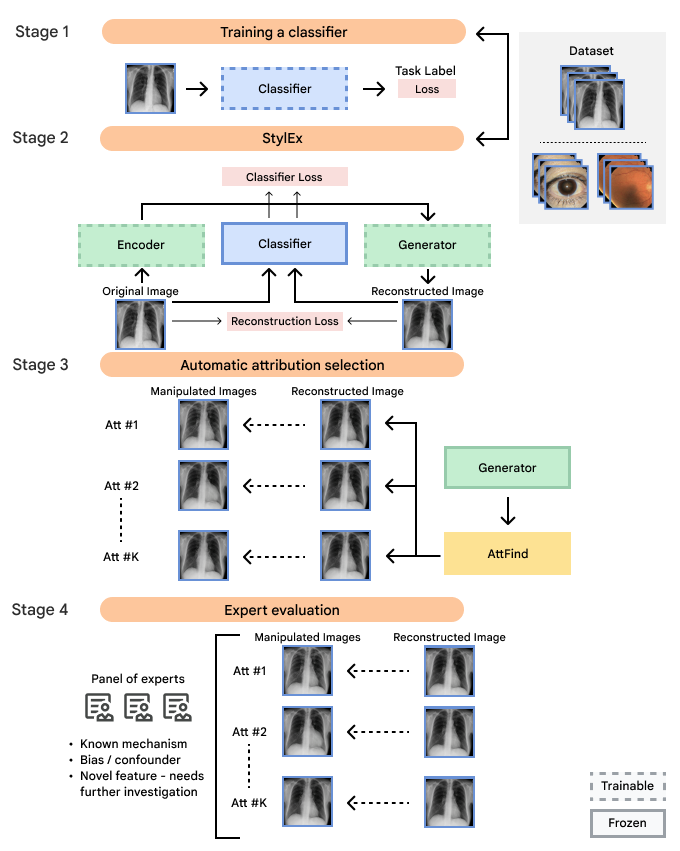
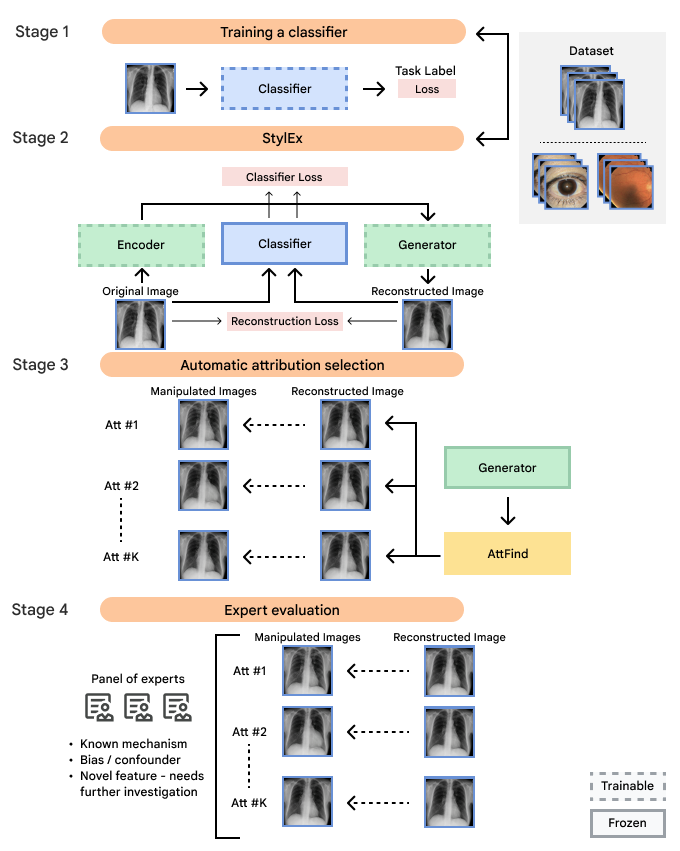
Reducing Human Error
According to the World Health Organization (WHO), patient harm is the 14th leading cause of global disease burden, comparable to tuberculosis and malaria. Medication, diagnostic, or surgical errors—which can occasionally result from subpar system design, complicated procedures, or worker stress—often lead to this harm.
For example, Therac-25 had six or more incidents between 1985 and 1987 due to programming errors that led to overdoses of radiation. This caused severe injuries—and, in some cases, death—to patients.
You should not automate the procedures without human oversight because the system is not liable for the mistake but for the people and businesses that created and operationalized the system.
The ideal solution combines high human supervision (e.g., monitoring, system, and model audits) and high automation in high-priority reflex-level situations (e.g., automatic drip medication infusions) to reduce the risk of complications and promote reliable and safe systems.
This approach reduces errors, simplifies physicians' workflows, and allows them to focus on higher-level patient interactions and decision-making.
AI in Drug Discovery
In medicine, AI can accelerate drug discovery by predicting drug interactions and efficacy, reducing time and cost in clinical trials, and efficiently identifying new drug targets and candidates.
Although this is in its early stages, it shows signs of potential.
Case Study
Exscientia is a pioneer in AI-designed drugs. They created DSP-1181, a potent drug, in less than 12 months, compared to the industry average of 4-6 years.
Sumitomo Pharma began Phase I clinical studies for DSP-1181 in January 2020 for obsessive-compulsive disorder, but the drug did not progress past Phase I. They were discontinued in July 2022 due to not meeting evaluation criteria.
Generally, it is challenging to get AI-designed drugs through FDA approval criteria in the US.
However, according to Boston Consulting Group, by 2022, over 150 AI-designed small-molecule drugs will be in the discovery phase, with more than 15 advancing through clinical trials.
Teams at Google DeepMind and Isomorphic Labs launched AlphaFold 3 in May, which predicts the structure and interactions of all of life’s molecules better than its predecessor, AlphaFold 2.
https://x.com/Google/status/1813296292887433463
Administrative and Operational-focused
AI improves hospital operations by optimizing scheduling, inventory management, and resource allocation, improving efficiency, and reducing costs.
Here, we explore three key areas where AI is making a significant impact:
Documentation
Healthcare professionals spend a lot of time on administrative tasks, particularly documentation and attending to patients. This reduces the time they can dedicate to patient care and usually contributes to burnout.
Case study
Lyrebird Health uses Deepgram's medical variant of the Nova-2 STT model to handle all medical documentation. This technology listens, learns, and generates notes instantly, reducing physician stress and minimizing errors due to the model’s high accuracy.
Deepgram’s Nova-2 Medical model can transcribe healthcare terminology, including symptoms, diagnoses, treatments, medications, and clinical jargon.
Compared to other models, this one has superior keyword accuracy, faster transcription times, and improved communication in medical settings. This can reduce the cognitive load when taking medical notes or communicating with patients cost-efficiently.
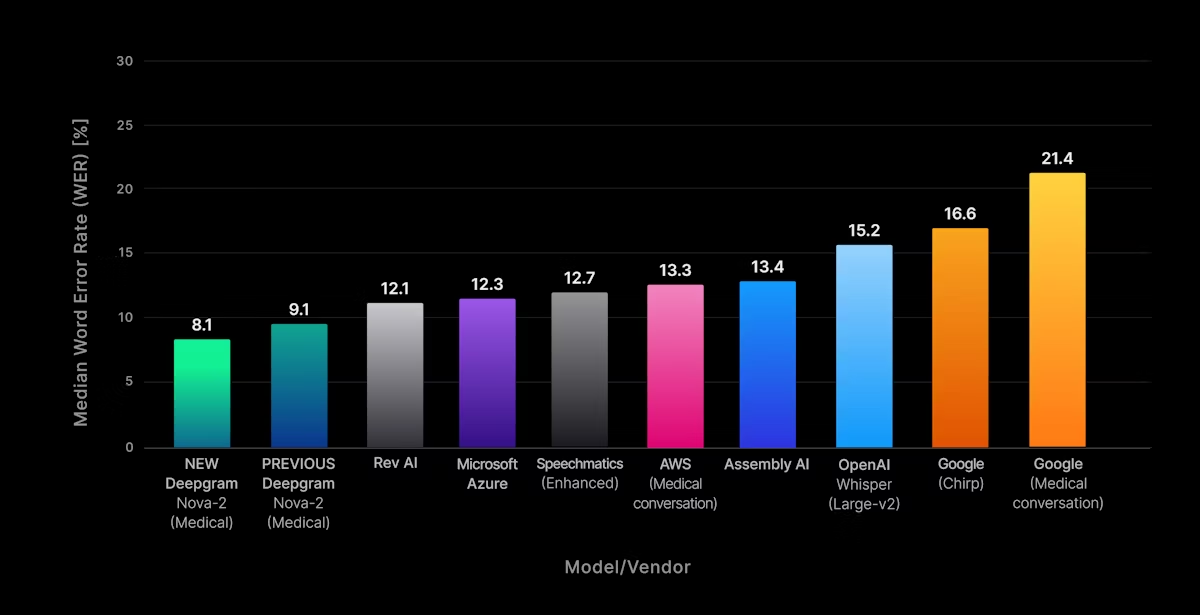
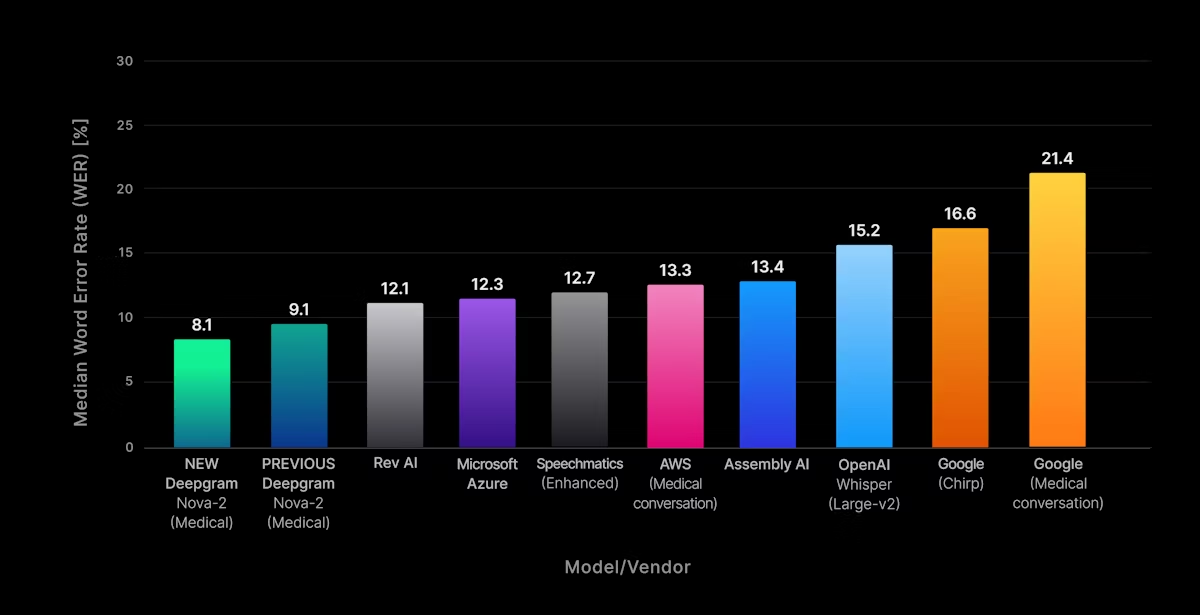
Daily.co provides telehealth customers with an API to generate tailored clinical note drafts critical to making the clinician’s workflow more productive. The API is powered by direct access to Deepgram’s real-time transcription models, among other tools.
Virtual Assistants
Managing patient inquiries, scheduling appointments, and handling routine administrative tasks can be time-consuming for the hospital staff and prone to errors.
Case study
Phonely is an AI receptionist who answers phone calls and accurately records patient information like a human. It integrates with tools like Google Calendar and Calendly to schedule appointments, transfer calls to a human, and automate follow-ups. Phonely operates on Deepgram’s Nova-2 STT API for optimal performance.
YourDoctor is an AI-powered conversational assistant that offers health advice. It allows users to describe their symptoms and generate personalized medical recommendations and guidance.
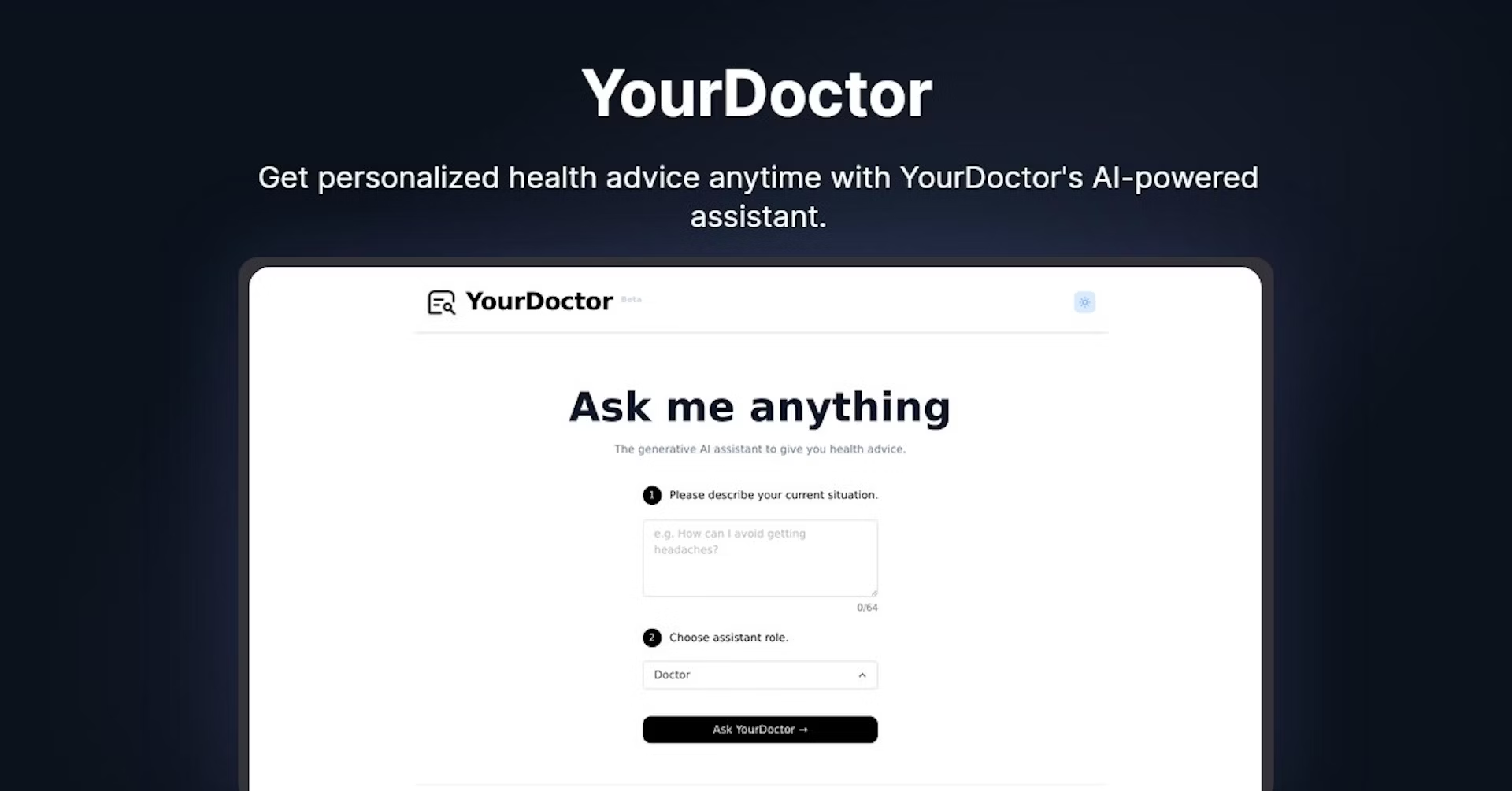
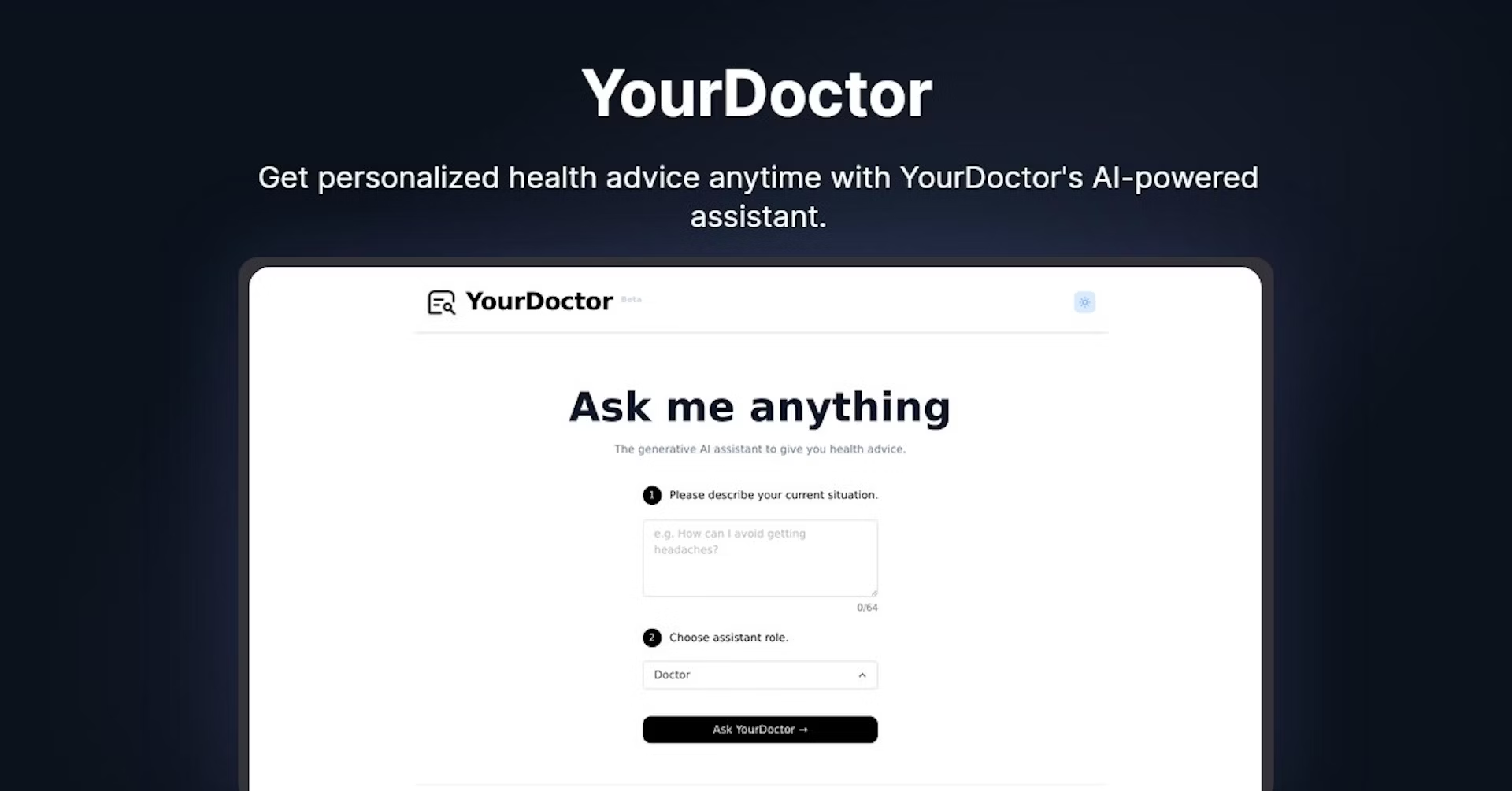
Medical Consultations
Healthcare systems often struggle with efficiently managing patient consultations, leading to long wait times and inefficient use of resources.
Case study
Middletown Medical improves patient care with a custom mobile app that helps people find doctors and request appointments. Using Deepgram's STT model, the app automatically transcribes telemedicine consultations, simplifying the documentation of notes and prescriptions.
Medical Chat is an AI-powered platform that instantly answers medical, clinical, and veterinary questions. It aims to be an accurate, reliable, and up-to-date resource for health information.
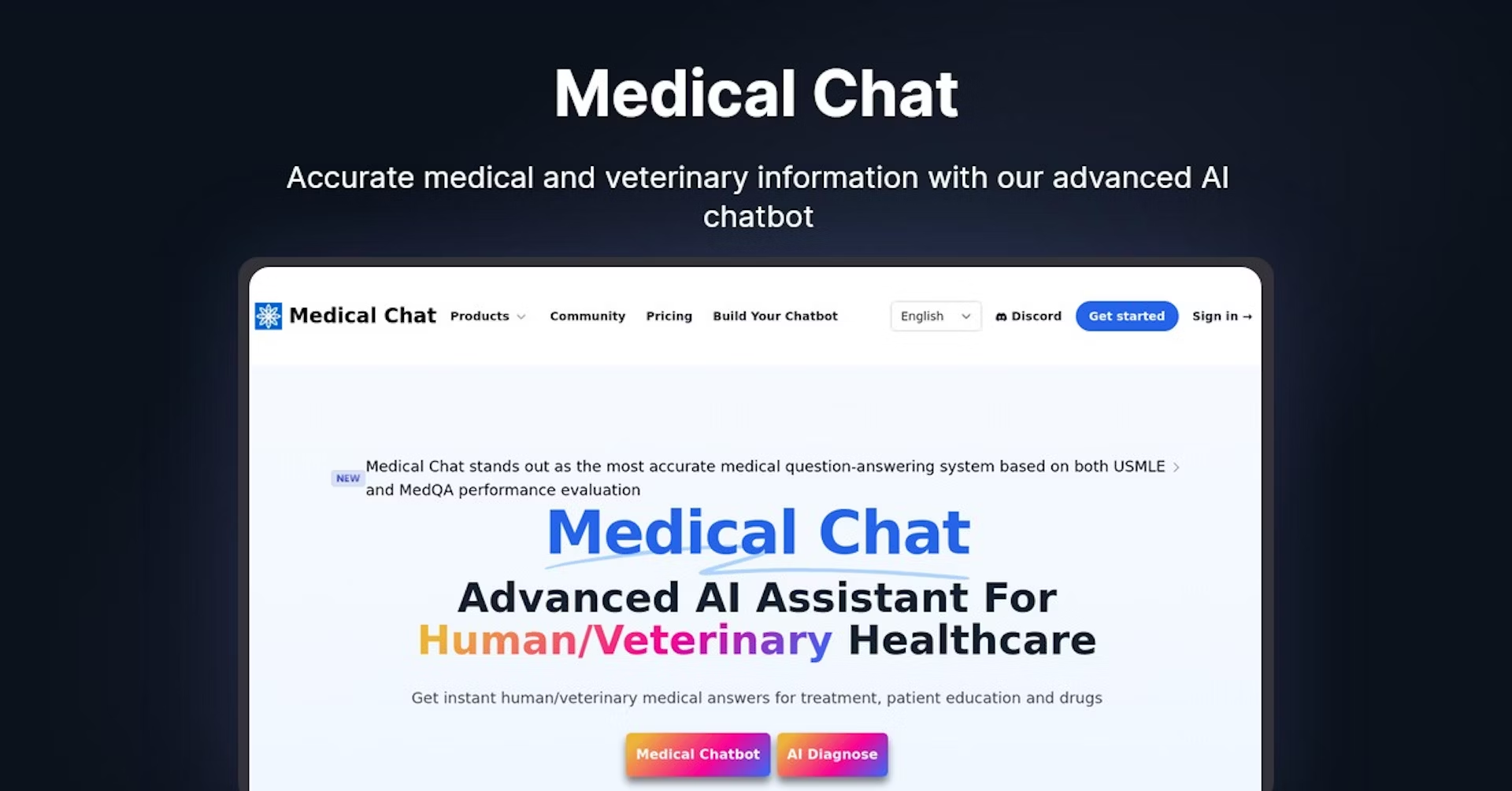
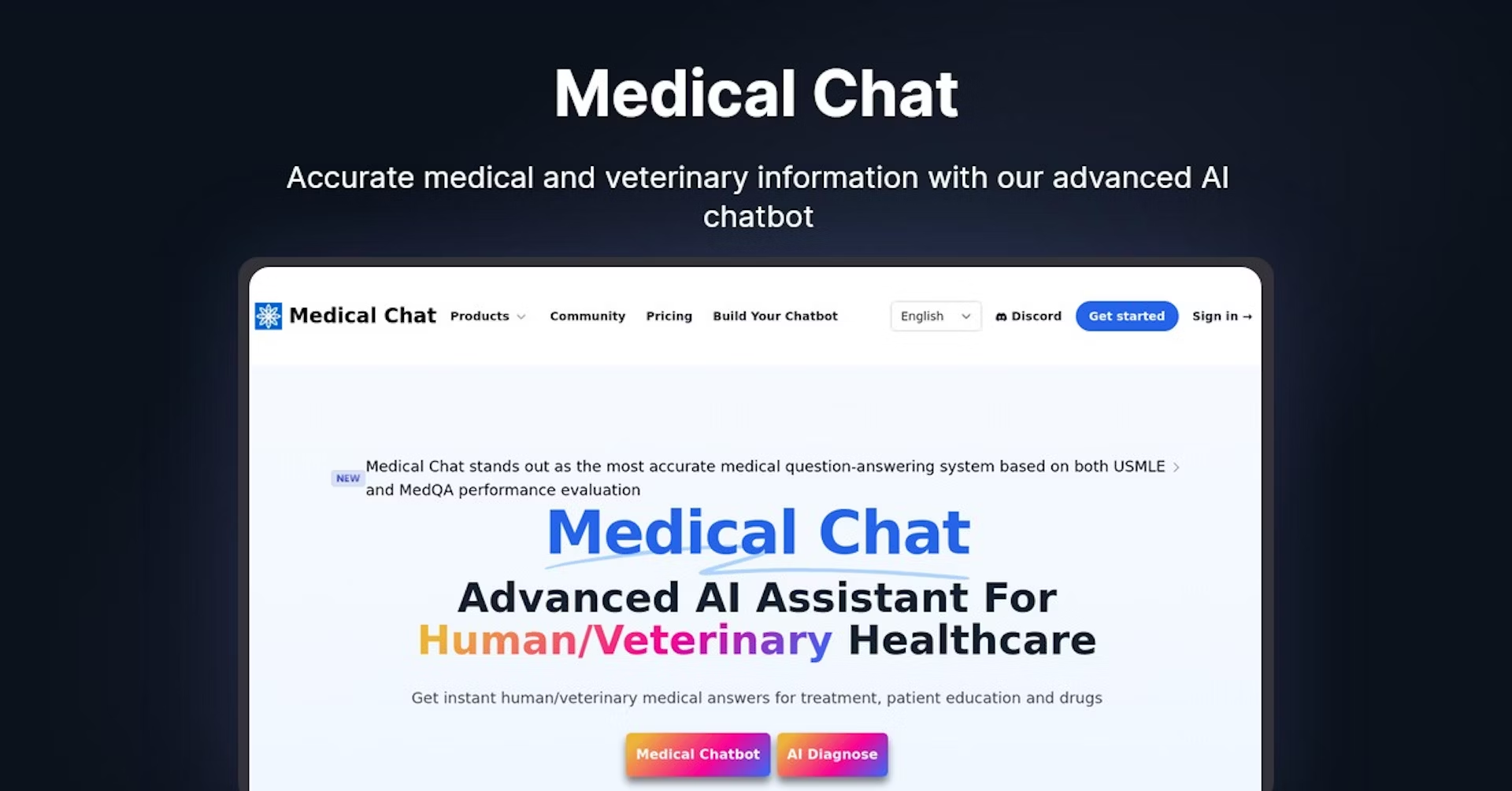
Challenges and Ethical Considerations in Applying AI in Medicine and Healthcare
AI models are designed to achieve their objectives based on available data and programming, using statistical measures to make decisions under uncertainty. In natural language applications, this uncertainty can sometimes result in "hallucinations" or responses that don't fit the context, which usually doesn't harm human lives.
In healthcare, that level of uncertainty can be the difference between life and death for patients who rely on AI technology for dosage or clinicians for diagnoses. Here are two core challenges of current AI applications in healthcare:
Data availability and potential bias in AI models
Privacy and security
Privacy and Security
Health data contain a lot of sensitive, personally identifiable information (PII), and privacy aims to ensure that an individual can’t be identified with the information in a dataset, whether in the dataset or not. Privacy is a fundamental human right, and a violation of this right can have serious consequences.
AI systems in healthcare must comply with strict regulations governing the use and sharing of personal information, such as HIPAA in the United States or GDPR in Europe.
This is a non-trivial issue because some bad actors breach the security of the systems via cyberattacks, including:
Such breaches can lead to unauthorized access to sensitive patient data, compromise patient privacy, and potentially disrupt healthcare services.
Data availability and potential bias in AI models
It is challenging to obtain high-quality, comprehensive datasets for some medical use cases due to privacy concerns, fragmented healthcare data systems, and the need for extensive labeling and annotation.
This can result in datasets with varying densities of knowledge (data points), ultimately leading to potential bias in the model.
Such bias might amplify disparities in patient representation and favor a limited number of treatment or diagnostic options, affecting outcomes for a diverse population, among other potential worst-case scenarios. Adversarial testing is required to test for bias in the model.
AI technology in healthcare has to be designed to be reliable, safe, and trustworthy. This ensures a level of human oversight over all operations.
In an ideal scenario, you can have much automation and the same amount of oversight to ensure that skilled workers who understand the system function as intended and keep patients safe.
Google DeepMind’s CoDoC exemplifies human-centered AI through human-AI collaboration. It improves reliability by identifying when predictive AI systems are uncertain.
CoDoC requires three inputs for each training case: the AI's confidence score, the clinician's interpretation, and the ground truth from clinical follow-up. This effort towards responsible and safe AI will likely inspire further research.
Conclusion: How AI Impacts the Medical World
AI is transforming healthcare across various domains, from personalized patient care and clinician support to enhancing administrative efficiency.
It plays a crucial role in diagnostics, treatment recommendations, drug discovery, and operational tasks, ultimately aiming to reduce costs and improve the quality of patient outcomes.
The vision for an AI-enabled future in healthcare promises better outcomes and increased efficiency, with AI systems augmenting human expertise rather than replacing it.
To achieve this, we must combine AI’s power with human oversight to prioritize patient well-being and ensure reliability, safety, and trustworthiness in all applications.
Frequently Asked Questions
What are the main areas where AI is transforming healthcare? AI impacts diagnostics (like medical imaging), treatment recommendations, drug discovery, and operational efficiency within healthcare institutions.
How does AI enhance patient care? AI enables personalized care by tailoring treatments and interventions to individual patient needs and preferences, facilitated through AI-powered medical assistants and remote IoT device monitoring.
What are the challenges associated with AI in healthcare? Challenges include ensuring data privacy and security, addressing potential biases in AI models, and managing the integration of AI without compromising patient safety or exacerbating existing healthcare disparities.
Why is a balanced approach between AI and human oversight crucial in healthcare? Balancing AI automation with human oversight ensures reliability, safety, and trustworthiness in healthcare operations while also preserving the critical role of human judgment and empathy in patient care.
How does AI contribute to operational efficiency in healthcare settings? AI optimizes hospital operations by automating scheduling, inventory management, and resource allocation tasks. It also enhances provider efficiency through smart documentation, intelligent alerts, resource management, chart summarization, intelligent triage, patient safety assessment, admission support, medical management, discharge support, quality improvement, and intelligent monitoring and follow-up systems. Additionally, AI technologies like chatbots and virtual assistants provide 24/7 patient support, improving engagement and satisfaction.
Unlock language AI at scale with an API call.
Get conversational intelligence with transcription and understanding on the world's best speech AI platform.