Decision Tree
As we dive into the concept of decision trees in machine learning, we explore their historical evolution, the simplicity behind their complex decision-making capabilities, and the statistical foundations that make them so effective.
Have you ever wondered how machines make sense of data and help in making decisions? The realm of machine learning is vast, but at its core lies a simple yet powerful tool—decision trees. These models, akin to the branching paths of a tree, offer clarity in the complex world of data analysis. Decision trees stand out in their dual capability to tackle both classification and regression tasks, making them indispensable in predictive modeling. But what truly sets decision trees apart is their mimicry of human decision-making processes, offering a level of interpretability that few other machine learning models can match. As we dive into the concept of decision trees in machine learning, we explore their historical evolution, the simplicity behind their complex decision-making capabilities, and the statistical foundations that make them so effective. How do these models transform data into decisions, and why are they considered a cornerstone in the field of machine learning? Join us as we unravel the intricacies of decision trees and their pivotal role in shaping the future of analytical projects.
What are Decision Trees in Machine Learning
At the intersection of simplicity and sophistication lies the decision tree—a fundamental supervised learning technique with a profound impact on the machine learning landscape. Decision trees excel in both classification and regression tasks, a versatility highlighted in the recent Coursera article. This duality in function allows them to not only categorize data but also predict continuous outcomes, showcasing their predictive modeling prowess.
Versatile Applications: As outlined on platforms like xoriant.com and mastersindatascience.org, decision trees model decisions and their possible consequences in a tree-like structure, closely mirroring the human decision-making process.
Simplicity and Interpretability: One of the most appealing aspects of decision trees lies in their simplicity. They provide a clear, interpretable model that makes them particularly suitable for analytical projects where understanding the decision process is as important as the outcome itself.
Historical Context and Evolution: The journey of decision trees in machine learning, traced back through a comprehensive analysis on analyticsvidhya.com from May 11, 2020, reveals their evolution from simple decision-making frameworks to complex models capable of handling vast datasets and intricate scenarios.
Statistical Foundation: At their core, decision trees derive from information theory. This statistical foundation ensures that each split in the tree maximizes the information gain, leading to the most informed decisions possible.
Through this exploration of decision trees in machine learning, we uncover not just the mechanics of how they operate but also the reasons behind their widespread use and the unique position they hold in the machine learning toolkit. How do these models continue to evolve, and what future applications might they unlock?
Key Terminologies in Decision Trees
Understanding the core terminologies associated with decision trees in machine learning is crucial for anyone looking to master this powerful tool. Each term represents a fundamental component that contributes to the decision-making capabilities of a decision tree. Let's delve into these terminologies, their roles, and how they interconnect to form a decision tree's structure.
Nodes, Edges, Root, and Leaves
Nodes: These are the points in the tree where decisions are made. Each node represents a test on an attribute, with branches to child nodes representing the outcome of that test.
Edges: Edges are the connections between nodes, guiding the path from one decision to the next. In the context of decision trees, they represent the outcome of the tests conducted at nodes.
Root: The root is the topmost node of the tree, where the decision process begins. It represents the initial test that starts the decision-making process.
Leaves: Also known as terminal nodes, leaves represent the final outcomes of the decision paths. They hold the decision or prediction the tree makes after all tests are performed.
Splitting and Pruning
Splitting: This process divides the nodes into two or more sub-nodes, enhancing the tree's decision-making capabilities. Splitting occurs based on certain criteria that aim to best separate the data into distinct classes or predictions.
Pruning: To prevent a decision tree from overfitting, pruning removes parts of the tree that provide little to no additional power in classifying instances. It simplifies the model, making it more generalizable to unseen data.
Entropy and Information Gain
Entropy: A measure of the randomness or disorder within a dataset. In decision trees, entropy helps determine how a node can be split in the most informative way. Lower entropy means less disorder and more purity in the dataset.
Information Gain: This metric measures the reduction in entropy after a dataset is split on an attribute. Higher information gain values indicate a more significant reduction in disorder, making an attribute an excellent candidate for splitting.
Attribute Selection Measures (ASM)
Attribute Selection Measures (ASM) stand at the core of decision tree algorithms, serving as the criterion for selecting the attribute that best splits the data at each node. According to the DataCamp tutorial on decision tree classifiers, ASMs evaluate the potential of each attribute in segregating the data into target classes, aiming to maximize the information gain or minimize impurity.
Gini Impurity vs. Entropy
Gini Impurity: A measure used to determine how often a randomly chosen element would be incorrectly identified. It reflects the frequency at which any element of the dataset will be mislabeled when it is randomly labeled according to the distribution of labels in the dataset.
Entropy: As mentioned, entropy measures the disorder or randomness in the data. It aims to quantify the uncertainty involved in predicting the outcome.
Both Gini impurity and entropy serve as measures for selecting the best attribute for splitting the data in a decision tree. The choice between using Gini impurity or entropy depends on the specific requirements of the machine learning task at hand. While entropy provides a measure of disorder based on information theory, Gini impurity offers an alternative that is computationally faster to calculate in practice, as discussed in the Machine Learning with R book cited in the analyticsvidhya.com blog from January 16, 2017.
In summary, these key terminologies form the backbone of decision trees in machine learning, each playing a specific role in the structure and function of the tree. From the initial split at the root to the final decisions made at the leaves, understanding these terms is essential for anyone looking to leverage decision trees in their machine learning projects.
How Decision Trees Are Structured
The architecture of decision trees in machine learning unveils a fascinating journey from simplicity to complexity, embodying a methodical approach to decision-making that closely mirrors human thought processes. Understanding this structure not only enriches one’s knowledge but also enhances the practical application of decision trees in solving both mundane and complex problems. Let's explore the anatomy and significance of its components in depth.
The Anatomy of a Decision Tree
The structure of a decision tree is both intuitive and strategic, designed to systematically break down data into smaller subsets to reach a conclusive prediction or classification. This breakdown is facilitated through various components:
Root Node: The starting point of a decision tree. It represents the entire dataset, from which the decision-making process initiates. According to the insights from christophm.github.io, the root node embodies the first condition that splits the data into two or more subsets.
Decision Nodes: As we traverse down from the root, decision nodes represent the conditions or questions that further segregate the data based on specific attributes. Each decision node branches out to answer a particular query related to the data.
Leaf Nodes: The terminal points of the tree where final decisions or predictions are made. Upon reaching a leaf node, one can determine the outcome based on the path followed through the tree.
Splitting the Data
The decision-making prowess of a tree lies in its ability to split the data effectively at each node. This process, as highlighted in the xoriant.com blog, involves selecting an attribute and partitioning the data into smaller subsets. The choice of attribute for each split is not arbitrary but is determined based on statistical measures that aim to maximize the purity of the subsets created. The goal is to organize the data in such a way that each subsequent split brings us closer to a definitive answer.
The Role of Tree Depth
The depth of a decision tree, or how far down the tree extends, plays a pivotal role in its complexity and accuracy. However, with increased depth comes the risk of overfitting—when a model learns the training data too well, including its noise and outliers, thereby performing poorly on unseen data. Analyticsvidhya.com sheds light on this aspect, indicating that deeper trees, while potentially more accurate, may not generalize well to new data. Balancing depth with model performance is, therefore, essential.
Pruning: A Necessary Measure
To mitigate the risks associated with deep trees, pruning becomes a critical step. Pruning involves trimming down parts of the tree that contribute little to the decision-making process. This technique not only helps in preventing overfitting but also simplifies the model, making it more interpretable and faster in making predictions. The concept of pruning underscores the importance of model generalization over mere accuracy on training data.
In essence, the structure of a decision tree in machine learning is a testament to the elegance of simplicity combined with the rigor of statistical analysis. From the root to the leaves, each component plays a critical role in deciphering the underlying patterns in the data, guiding us to informed decisions. The process of splitting, influenced by the depth of the tree and refined through pruning, illustrates a balanced approach to achieving both accuracy and generalizability in predictive modeling. Through this structured methodology, decision trees not only offer a clear visual representation of decision-making but also serve as a robust tool for tackling a wide array of problems in machine learning.
Building Decision Trees
Building a decision tree in machine learning involves a structured and methodical process that mirrors the decision-making prowess of the human mind. This process ensures that the final model is not just a repository of data but a reflection of the intricate patterns and relationships within it. Let's delve into the step-by-step process of constructing a decision tree, highlighting the significance of each phase and the meticulous considerations involved.
Selecting the Best Attribute
Attribute Selection Measures (ASM): The cornerstone of decision tree construction is the selection of the best attribute at each decision node. This decision, as detailed in the DataCamp tutorial, hinges on ASM, which evaluates the potential of each attribute to segregate the data effectively, aiming for homogeneity or purity in the resulting subsets.
Algorithms for Attribute Selection: The choice of algorithm significantly influences the attribute selection process. Prominent algorithms include ID3, C4.5, and CART, each with its unique approach. For instance, ID3 (Iterative Dichotomiser 3) prioritizes attributes with the highest information gain, while C4.5, an evolution of ID3, also considers the ratio of information gain, allowing for more balanced trees. Conversely, CART (Classification and Regression Trees) uses the Gini impurity as a metric, suitable for datasets with categorical targets.
Splitting the Dataset
Dataset Division: Following the selection of an attribute, the dataset splits into subsets, each corresponding to a possible value of the attribute. This process is recursive, with each subset potentially serving as a new decision node if further splits are warranted. The aim is to create branches in the tree that lead to leaf nodes with homogeneous or pure outcomes.
Handling of Missing Values and Categorical Data: An inherent challenge in building decision trees involves dealing with missing values and categorical data. Techniques such as imputation for missing values and encoding for categorical data ensure that the model remains robust and reflective of the underlying data distribution.
Pruning the Tree
Preventing Overfitting: As underscored in the "Machine Learning with R" chapter, pruning is essential to prevent overfitting, a common pitfall where the model learns the noise in the training data to the detriment of its performance on unseen data. Pruning involves removing branches that have little impact on the overall accuracy, thereby simplifying the model.
Role in Enhancing Model Generalization: By eliminating redundant or non-informative branches, pruning not only bolsters the model's ability to generalize to new data but also enhances interpretability, making the decision process more transparent and understandable.
Ensemble Methods: Boosting Decision Tree Performance
Leveraging Strength in Numbers: Decision trees, while powerful, often benefit from being part of an ensemble method, such as Random Forests, Gradient Boosting, or XGBoost. These methods combine multiple decision trees to form a more accurate and robust prediction model.
Random Forests: Incorporate numerous decision trees built on randomly selected subsets of the data and attributes, essentially creating a "forest" of trees whose collective decision, typically through majority voting, yields the final prediction.
Gradient Boosting and XGBoost: Focus on sequentially improving the prediction accuracy by correcting the errors of previous trees. XGBoost, in particular, has gained acclaim for its efficiency and performance across various machine learning competitions, as highlighted in the medium.com analytics vidhya blog post.
In constructing decision trees, each step, from selecting the best attribute using ASM to pruning the tree, is pivotal. These steps ensure that the model not only accurately captures the complexities of the data but also remains adaptable and interpretable. By addressing challenges such as handling missing values and leveraging the power of ensemble methods, decision trees continue to stand as a testament to the blend of simplicity and efficacy in machine learning.
Types of Decision Tree - Classification and Regression Trees
The realm of decision trees in machine learning is diverse and nuanced, tailored to address a broad spectrum of data-driven questions. At the heart of this versatility lie two primary types of decision trees: classification trees and regression trees. Each serves a distinct purpose, sculpting the landscape of machine learning applications with precision and adaptability.
Classification Trees vs. Regression Trees
Classification Trees: These trees excel in sorting data into predefined categories. They thrive on categorical outcomes, where responses are discrete, such as 'yes' or 'no', 'spam' or 'not spam'. A recent Coursera article from Nov 29, 2023, underscores their utility in scenarios where the prediction of a category is paramount. For example, in medical diagnoses, a classification tree might predict whether a patient has a disease based on symptoms and test results.
Regression Trees: In contrast, regression trees deal with continuous outcomes. They predict a quantity rather than a category. This distinction is critical in fields like real estate, where a regression tree could predict the price of a house based on features such as square footage, location, and number of bedrooms. The Coursera article delineates this difference, emphasizing the role of regression trees in predictive modeling where the outcome is a numerical value.
Real-World Applications
For Classification Trees:
Email spam filters categorizing emails as 'spam' or 'non-spam'.
Loan approval systems deciding whether to approve or reject a loan application.
For Regression Trees:
Predicting housing prices based on various attributes like location, size, and age of the property.
Forecasting sales figures for the next quarter based on past performance metrics and market trends.
Impact on Algorithms and Splitting Criteria
Classification Trees focus on maximizing information gain or minimizing impurity (e.g., using Gini impurity or entropy). This approach ensures that each split in the tree makes the resulting subsets as pure as possible in terms of the target variable.
Regression Trees aim to minimize variance with each split. By reducing the variance, the model ensures that the predictions are as close to the actual values as possible, enhancing the model's accuracy.
The Hybrid Approach in Complex Models
The versatility of decision trees extends beyond their individual use. In complex machine learning projects and competitions, a hybrid approach, leveraging both classification and regression trees, proves invaluable. This strategy enhances the model's accuracy and adaptability, allowing it to tackle intricate problems with finesse. For instance, in a competition to predict customer churn, a model might use classification trees to identify potential churners and regression trees to predict the likelihood or timing of churn.
The integration of classification and regression trees into complex models showcases the ingenuity and flexibility of decision trees in machine learning. By selecting the appropriate type of tree and tailoring the algorithms and splitting criteria to the specific needs of the problem at hand, data scientists unlock powerful solutions to a wide array of predictive challenges.
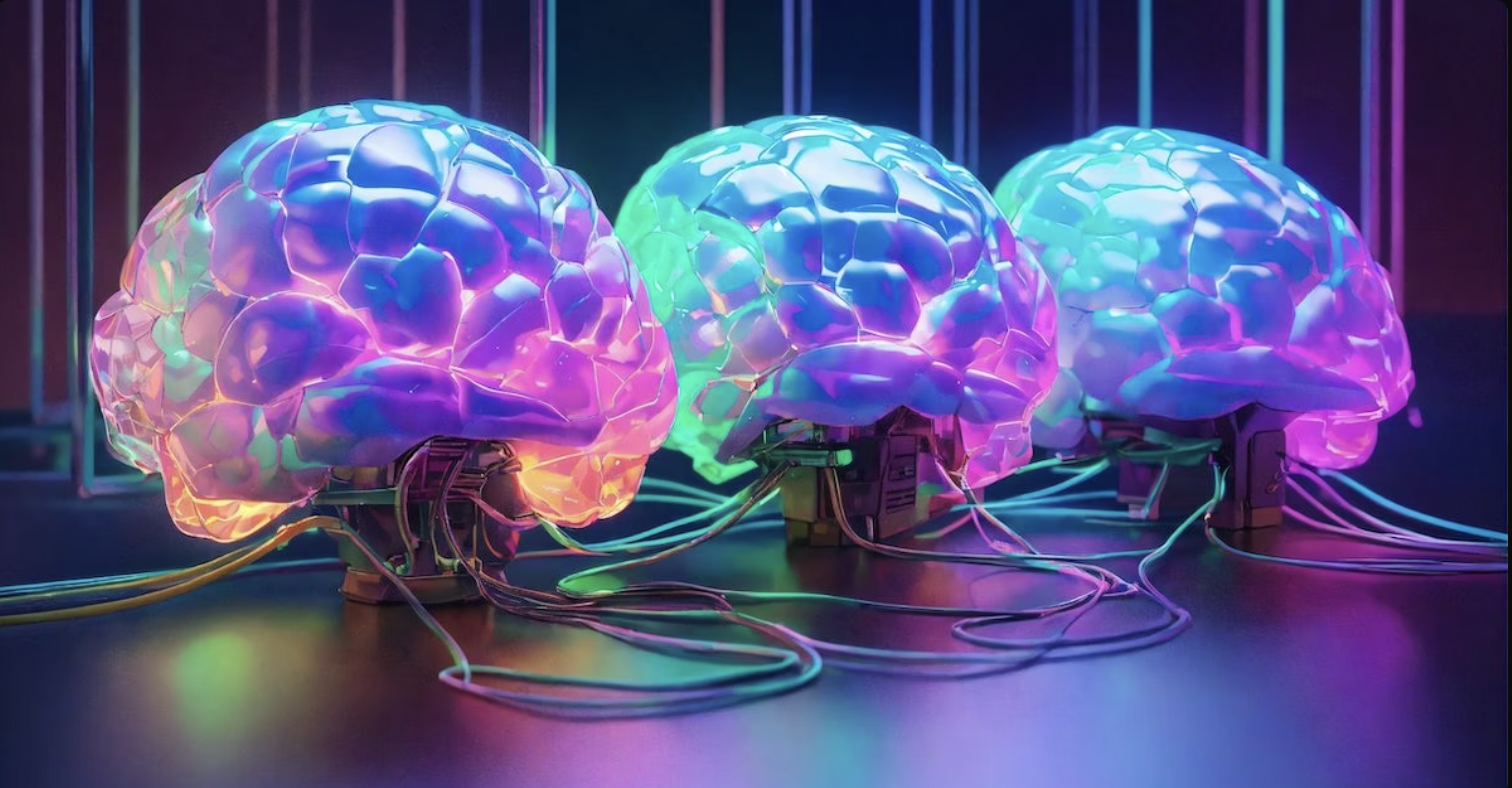