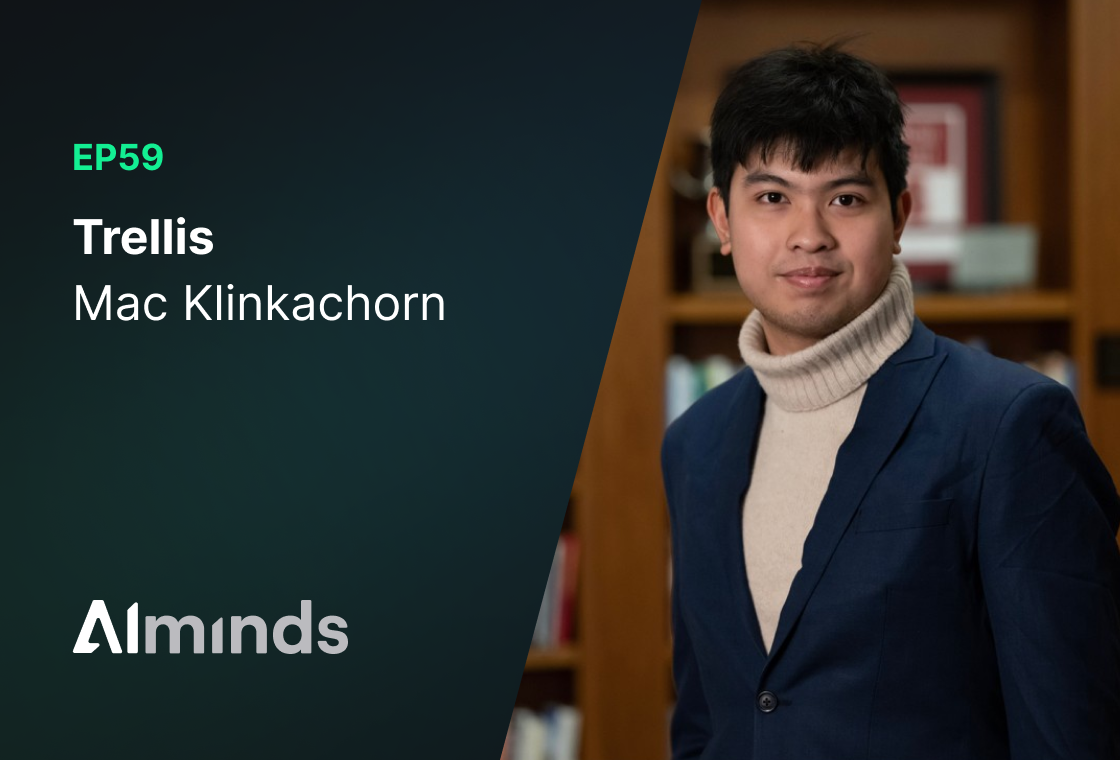
AI Minds #059 | Mac Klinkachorn, Co-Founder & President at Trellis
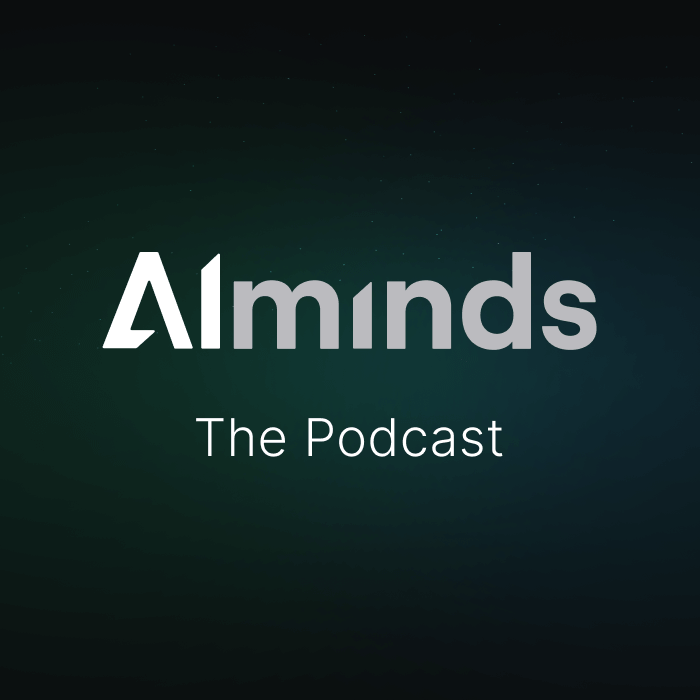
Mac Klinkachorn, Co-Founder & President at Trellis. Trellis automates manual PDF tasks at scale with AI agents. Trellis integrates end-to-end with your systems of record and allows users to define infinitely complex workflows to capture their business processes.
Listen to the episode on Spotify, Apple Podcast, Podcast addicts, Castbox. You can also watch this episode on YouTube.
In this episode of the AI Minds Podcast, Mac Klinkachorn, Co-Founder & President at Trellis, shares his journey from physics to AI, tackling unstructured data automation at scale.
Mac discusses his early entrepreneurial ventures in Thailand, his transition from physics to AI at Stanford, and how he discovered the challenges of handling unstructured data in enterprises.
He explains how Trellis automates PDF workflows, transforming messy, non-standardized documents into structured, actionable data, particularly in industries like healthcare and real estate.
The conversation delves into the technical and operational hurdles of AI-powered document processing, the evolving role of LLMs, and how Trellis integrates validation and workflow automation.
Mac also shares insights on AI’s future in enterprise automation, why data extraction is only part of the challenge, and how Trellis is pushing the boundaries of AI-driven efficiency.
Show Notes:
00:00 Innovative Leak Detection Business
05:11 Founding Trellis: Streamlining Data Processing
06:56 Automate PDF Workflows Pain Point
09:46 Trellis: Traceable PDF Data Processing
15:43 "Persisting Challenges in PDF Automation"
16:45 "Automated PDF Processing with AI"
More Quotes from Mac:
Demetrios:
Welcome back everyone to the AI Minds podcast. This is a podcast where we explore the companies of tomorrow being built AI first. I'm your host Demetrios. And this episode, like every episode, is brought to you by Deepgram. The number one speech to text and text to speech API on the Internet today. Trusted by the world's top enterprises, conversational AI leaders and startups, some of which you may have heard of like Spotify, Twilio, NASA and Citibank. I have the pleasure this episode to be joined by the co founder of Trellis Mac. How you doing today, dude?
Mac Klinkachorn :
Doing great, thanks for having me.
Demetrios:
Well, I know you're in Vegas right now for the HIMSS conference, so I'm glad that you took some time out of your day to join us. I want to start with you at 15 years old, starting a company. Tell me about that. And how did you even have the audacity to start something at that age?
Mac Klinkachorn :
So I grew up in Thailand and back when I was 15, my dad just got laid off from his job. And one of the big problem we had in our household is that there's like this small water leaks that just keep on going. We call in a lot of plumber. The very traditional approach to water leak detection is to just smash everything down and then find a leak. But with some physics, I realized that you can really find the leak pretty efficiently with just looking at water pressure. So that became the idea for the first business detecting water leaks at industrial scale. And that was like quite a fun thing. Nothing related to software or software as a service, but it's a very like direct, finally get paid kind of set up, which is quite interesting.
Demetrios:
So physics feels like it is going to be a theme that runs through your life. At 15 you already knew you had a little bit of a love relationship with physics.
Mac Klinkachorn :
I think it's a really interesting way of distilling very complex things into something really simple. And I think computer science is the opposite way where you try to construct complex things out of simple primitives. So I think that's kind of like going two ways. But I think physics is really interesting where you see something very complex and you try this to understand and distill it down to very simple setup.
Demetrios:
So because of your love of physics, from my understanding you were able to win a scholarship that took you around the world and landed you at Stanford.
Mac Klinkachorn :
So in high school there's this competition called International Physics Olympiad. All kids who spend too much time studying physics get to compete in different interesting locations. I went to Siberia and Indonesia to do that and as a result of that got a scholarship to study physics or anything else at Stanford.
Demetrios:
Incredible. Now at Stanford, what happened? You had a little bit of a life crisis.
Mac Klinkachorn :
So I went into Stanford thinking that I'll be a physicist and I studied a bit of physics. Looking into doing more physics research and realized that a lot in physics right now, the timeline for starting it and seeing the results in the range of 15 to 20 years. And back at the time at Stanford, there's this AI wave that's going on. Not anything as big as today, but people are starting to realize the potential of these language models or image model. I think imagenet came out a few years before as well.
Demetrios:
And where did that take you? You completely did a 180 and started working on AI and ML use cases instead of physics.
Mac Klinkachorn :
So I started pretty simple playing around with the BERT models, using it to build system to classify different texts and resume that we got for a hackathon Work with Chris Peach who spent a lot of time in computational education and thinking about ways we can tack along LLM models to help speed up the process of student learning. Spend some time at professor company Sebastian Thurn Cresta looking at ways to use LLM to coach sales agent to become better. So these initial building blocks of LLM that are not even like these LLM are not even as good as today. But back then you can see the potential of this technology changing how human or knowledge workers are being done.
Demetrios:
And then what happened? You get out of school, you decide you're not going to go work for a company or you have seen the light and you want to create your own company.
Mac Klinkachorn :
After working at a few startups I was this is pretty cool. Seems like there's going to be still a lot of open problem to tackle. So in my final year at Stanford, I was at the Stanford AI lab with my buddy Jackie and we just hack around a bunch of stuff building different projects. And in any AI or ML projects that we work on, one of the most painful part is cleaning up the data and getting these unstructured data, which is anything from PDF, text, audio, video into a format where it's usable in production. And we were, anytime we want to do something cool, we want to, let's say, make predictions out of medical notes or analyze millions of voice calls. We need to go through a 6 to 12 month process of setting up the pipeline to clean these data up, parse it map it. There's a lot of edge cases and outliers and it just feel like not fun part of cleaning that data up. So we were, there's probably a better way to do this and a more standardized, streamlined way to leverage these data at scale without having to go through that setup, that's kind of like the starting seed of Trellis.
Mac Klinkachorn :
And we end up iterating through a few ideas and land at Trellis, where we help companies automate unstructured data at scale, starting with PDF, which is the most painful data type. Originally we were playing around with video audio, but in enterprises, one of the data source that people complain about every day is PDF and that's where we think we can have the most impact.
Demetrios:
I told you before we hit record, when I was looking at Trellis's big on the website, the first thing you see when you go to run trellis.com, it says, Automate your PDF workflows at scale. And I said, say no more, you're speaking my love language. Let's talk. It is the most painful and it is also the most ubiquitous. Especially if you're working in finance or these sectors, like regulated sectors, everybody comes to you with their different PDFs and you can't really, when you try to ingest it. I think one of the big hang ups is that the different fields get thrown all over the place. And so it's very complex to ingest it and have the same looking file that you had before you ingested it.
Mac Klinkachorn :
So we iterated around with the headline a bit, but I think Automate PDF at scale, Deploying AI agent to process PDF scale at scale seems to resonate a lot of people. And as you mentioned, the hard thing about PDF is that there's no standardized format. And I think that's for a good reason, because PDF in a sense is a way for. It's like a human API. It's a way that humans use to communicate with each other. It encodes both business logic, domain understanding and maybe like business process as well.
Mac Klinkachorn :
So as a result, there's no standardized format. And in our opinion, there will never be a standardized format to, exchange some of these data because it just, people need a way to express their complex ideas and send it to other humans. But the unfortunate thing is that these ways of expressing very complex ideas are not very well suited for computers and automated systems to work with.
Demetrios:
Yeah, 100%. And it is, it's really interesting for me to Learn about how you're ensuring the accuracy. Because one complaint that I've heard with PDFs is maybe you have this PDF and it has a lot of information or text and then it has an image or it has a table and when you're ingesting that data and then later you want to do something with that information and in the text that says reference image 3.2 or reference table 1.1, you are not sure if your data is correctly pointing to the right image, especially if you get to large scale.
Mac Klinkachorn :
So at Trellis we build a pretty complex multi step pipeline. When the PDF come in we combine OCR and visual language models to effort like the first step, take the content out and then we help the companies define any mapping of how they want to structure the data, whether it's complex, JSON extracting table out of table, getting the data in the correct date or address format and then from there once we get the data out, we have a reference systems where for every field that we process and extract, we go and find where it's coming from. And when the users go into the product, they can click and see the exact location, exact bounding box of where they filled, whether it's a contract clause, information about a patient, a simple thing like birth date, exactly where it's coming from in a PDF. And that has been pretty powerful in really grounding the output of these AI models and providing visibility to our customers around how these process are being done. And it's all like a magic black box where you get like pretty clean data out but you can really trace back to the origins.
Demetrios:
So if I'm understanding this correctly, basically it is almost like a citation for PDF. So if you have something in digital format and you want to say is this really referencing? The box can highlight both of those and say show me the actual document.
Mac Klinkachorn :
So you can click and do like the citation to show you the original documents. The other way we tackle accuracy issues is allowing people to define workflow logic on top of that extracted data. And what I mean by that is that a lot of times when you extract either invoice or medical documents out, there's like some rule that underlie the data. The date must be less than the current date or a bit more complex than that. This number or this ID needs to align with whatever is in my ERP or ehr.
Demetrios:
Yeah.
Mac Klinkachorn :
And we allow people to reference these variable trigger workflow that connect with external API and use it to validate the extracted results. So it's like Both the LLM doing a check, but also external systems that are providing the second guardrails.
Demetrios:
What are some of these huge gains that you've seen clients get because they now have access, digital access to PDFs in a way that they didn't have before?
Mac Klinkachorn :
So I think digital access to PDF is maybe part of it. The key part of it is automating workflows and being able to gain real label savings. Which means that a process that used to be human doing manual data entry, now it's mostly automated and then humans can spend time doing more interesting and fruitful work. We spent quite a bit of time with companies in healthcare sectors and one of the major bottlenecks in that process is patient referral data and intaking different clinical reports. Different and clinical data. And in the previous world, in the pre LLM, pre AI world and even currently today people are getting a fax in, someone is doing the manual mapping of getting the right data points, answering different questions in a new form, doing that mapping manually. So it's like a huge time sunk. And with these process being in place, they have full visibility since it's all structured and in a database.
Mac Klinkachorn :
But also they can automate like 95 to 99% of what you have to do today and really realize that labor savings and ROI improvement at scale.
Demetrios:
That's brilliant. So in other words, it's not necessarily digitizing the PDF that is the best part about it. It's what you can do once you digitize that and what workflows you can build on top of that.
Mac Klinkachorn :
So that I think is a key part and it's interesting where in most enterprises, Especially in Legacy 1, Healthcare, Real Estate PDF is a unit of work. Every real estate transaction and we work with a few real estate companies, every real estate transaction start with someone sending packets of documents in. Similar to healthcare, every patient referral or prior authorization request starts with a packet of documents. And then someone need to go and scramble and map things and check things. But that's kind of like the starting point. And it's an enterprise unit work a hundred percent.
Demetrios:
I know a team that has. They're like a holding company, a finance holding company. And because they work all over the world, they're constantly being. They're hit up by banks all over the world that they bank with because the banks are asking all these questions for the know your customer. And those know your customer documents, they don't come in nice little Google documents or Word documents, they come in PDFs.
Mac Klinkachorn :
Scans the driver license, someone taking a picture, someone sending in a bank statement and things like that.
Demetrios:
So painful to think. And I know that they were using. I think they had 42 people. And I was talking to somebody because they embedded a machine learning engineer into the team to try and figure out where they could use AI to streamline processes. And this person that I was talking to said the bane of my existence are PDFs and it is so it's not a small feat that you're doing. I know that they are working really hard to try and create something like this for themselves. And they said it's been very difficult.
Mac Klinkachorn :
That's good to hear. And a number of our customers come to us that way as well. Where they were like I thought this would be a quick few months ML project. And a year later we still stuck dealing with these PDF definitely. And they had counted, you see that people are still doing these manual PDF work are in the 40, 50 in the hundreds. Which is kind of crazy to me. When we started Trellis, but now seems to be something that are still pretty commonplace. One interesting note that might be interesting for the listeners is that a lot of PDF automation has been around for quite a while.
Mac Klinkachorn :
But the traditional ML OCR approach really you have to build that system every time that you want to do PDF processing or do a mapping based on your business logic.
Demetrios:
What do you mean mapping every time that you get the PDF and then you have to manually look at the PDF and one by one say this table with this question. That type of thing.
Mac Klinkachorn :
So the traditional ML approach you would need to label a pretty large set of data for every type of PDF before that system become automated. But the interesting thing with the language model based approach, especially with fine tuning and prompting, is that it can almost work out of the box and then it improves over time because these language models already have semantic understanding of the data. So you don't have to be as precise and you don't have to manually label these data. And I think this is one of the big unlocks because then you can define your business logic and your workflows on the fly and the systems can get working out of the box and improve over time as opposed to having to manually fine tune and train these models which is quite and expensive and extensive tasks.
Demetrios:
So do you not fear those models getting so good that someone would not need a tool like Trellis?
Mac Klinkachorn :
That's something that's always top of mind at Trellis.
Demetrios:
Right.
Mac Klinkachorn :
Where what happens if models get better? I think one of the key things that we are seeing is that data extraction, mapping, like the part that we do, is only like 10 or 20% of the work to be done. The key part is once you extract the data, how do you build workflows around it? How do you trigger different actions that would really automate the job to be done? Things like validation, check, getting references, allowing people to do audit and evaluations on top of these data, doing analysis on top of these process data. Those things are something that I think even the best foundational models can really automate. And that's where I think there's a lot of value building product around that.
Hosted by
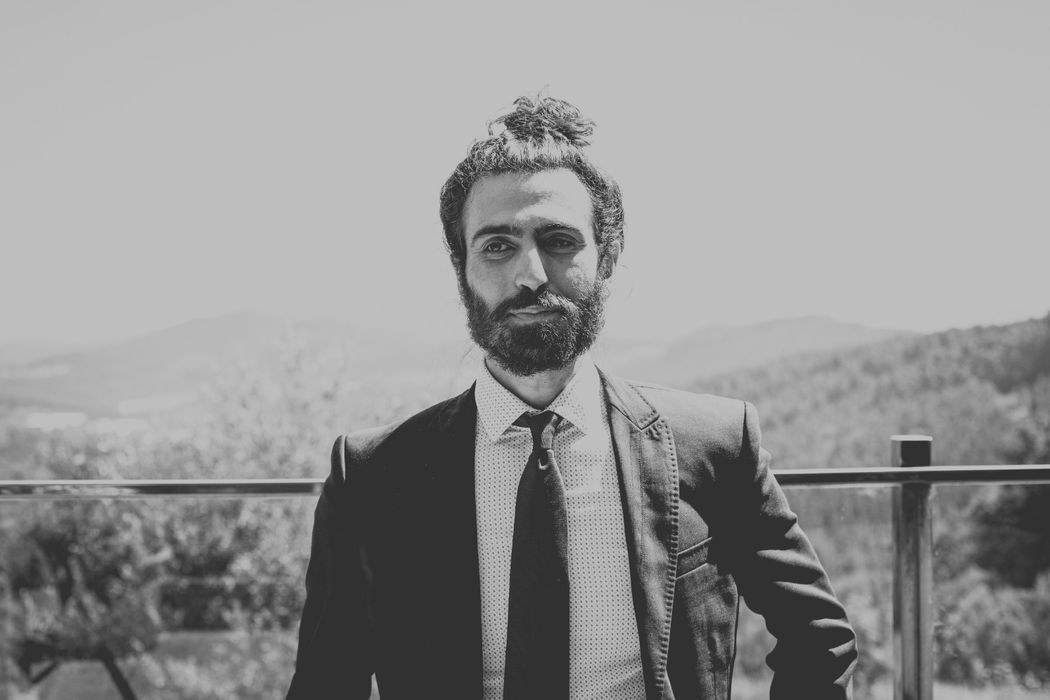
Demetrios Brinkmann
Host, AI MindsDemetrios founded the largest community dealing with producitonizing AI and ML models.
In April 2020, he fell into leading the MLOps community (more than 75k ML practitioners come together to learn and share experiences), which aims to bring clarity around the operational side of Machine Learning and AI. Since diving into the ML/AI world, he has become fascinated by Voice AI agents and is exploring the technical challenges that come with creating them.