Introducing Aura-2: The World’s Most Professional, Cost-Effective, and Enterprise-Grade Text-to-Speech Model
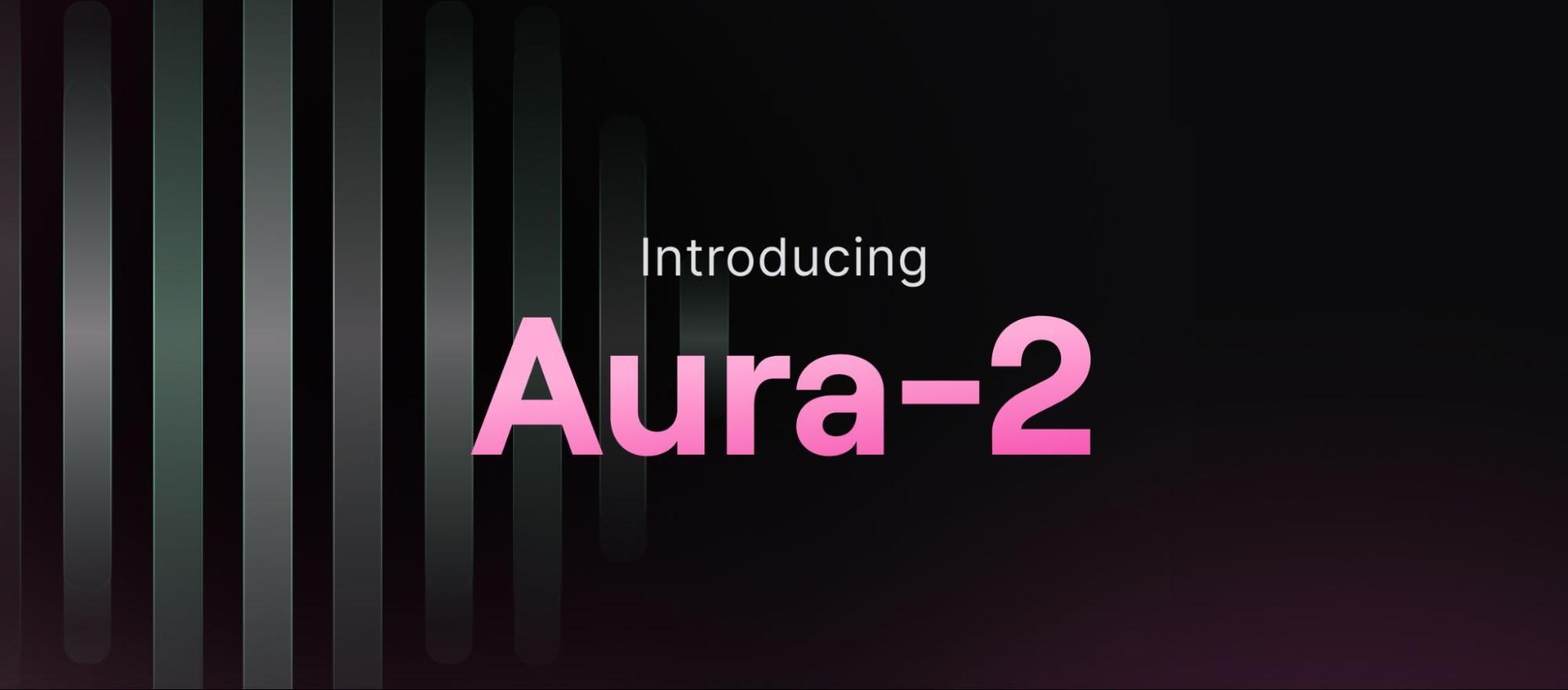
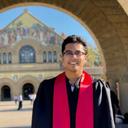
TL;DR
The First Enterprise-Grade TTS Model: Aura-2 is the only text-to-speech (TTS) model purpose-built for enterprise use cases, rather than entertainment scenarios.
Natural, Accurate, and Fast: Delivers human-like speech with domain-specific pronunciation, including drug names, legal references, alphanumeric identifiers, and structured inputs like dates, times, and currency values. It also achieves sub-200ms TTFB latency and offers pricing that supports large-scale use.
Powered by Deepgram Enterprise Runtime (DER): Supports flexible deployment options across cloud, VPC, and on-premises environments, along with model hot-swapping and real-time optimization. These are capabilities that most TTS vendors are unable to provide.
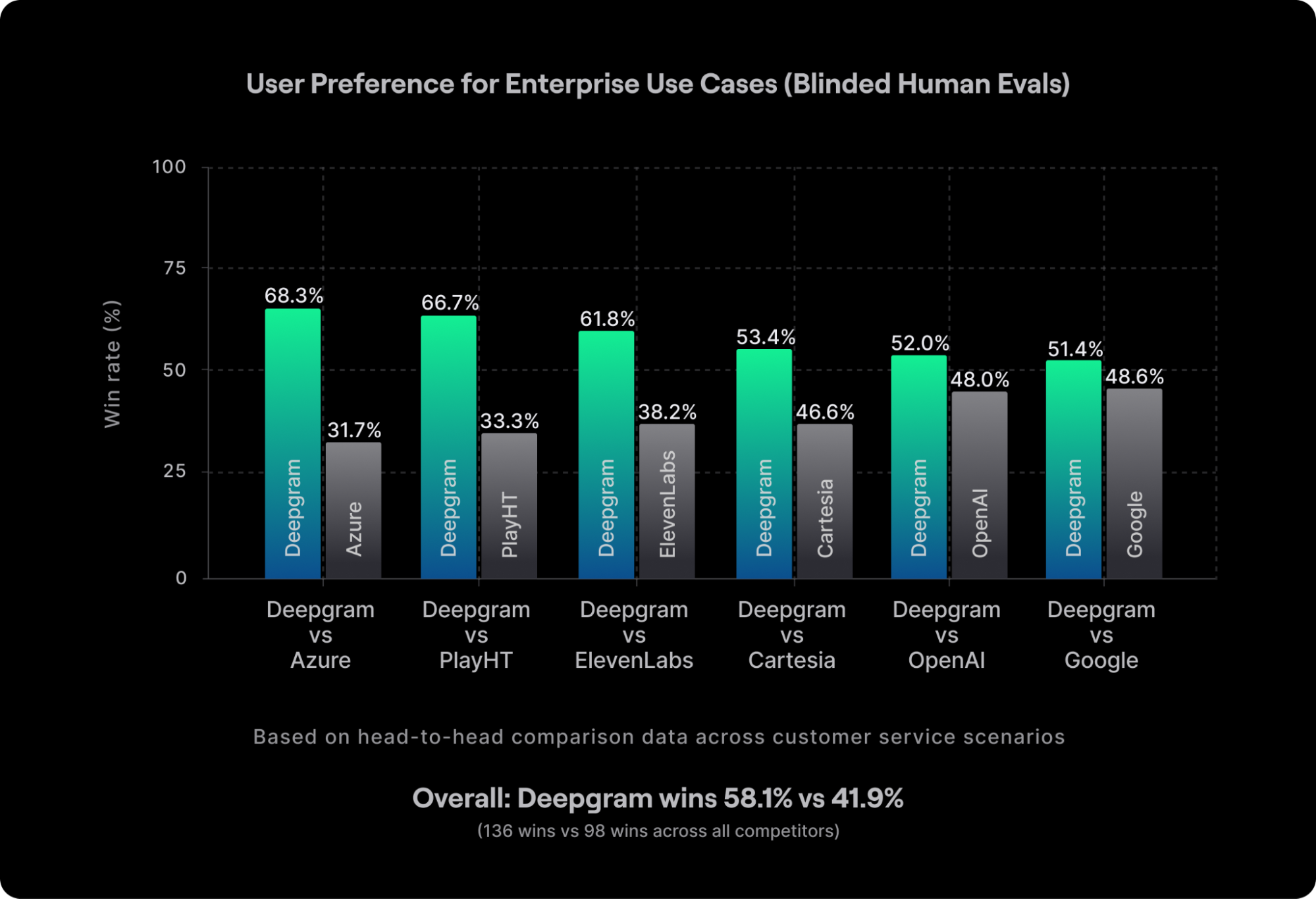
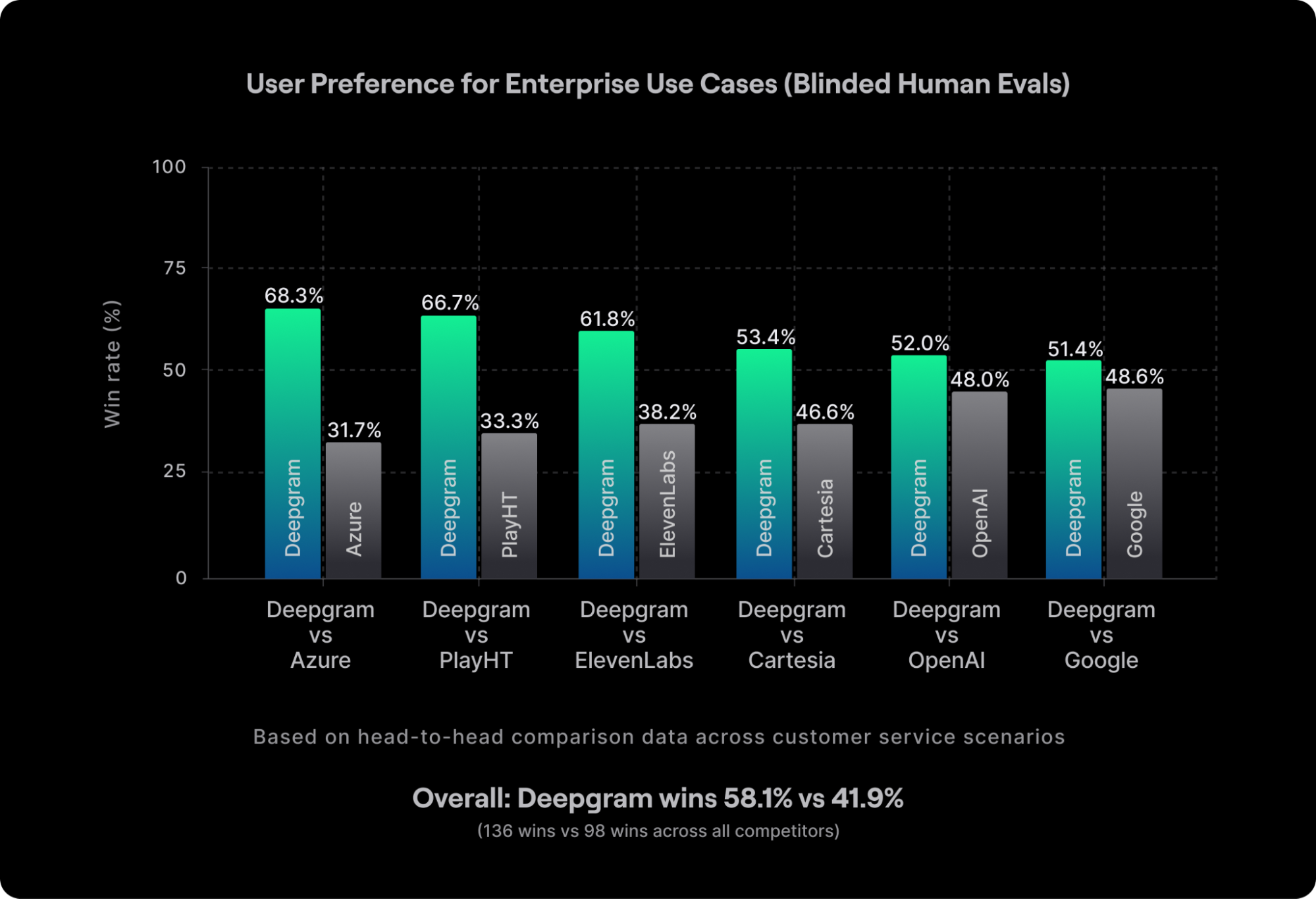
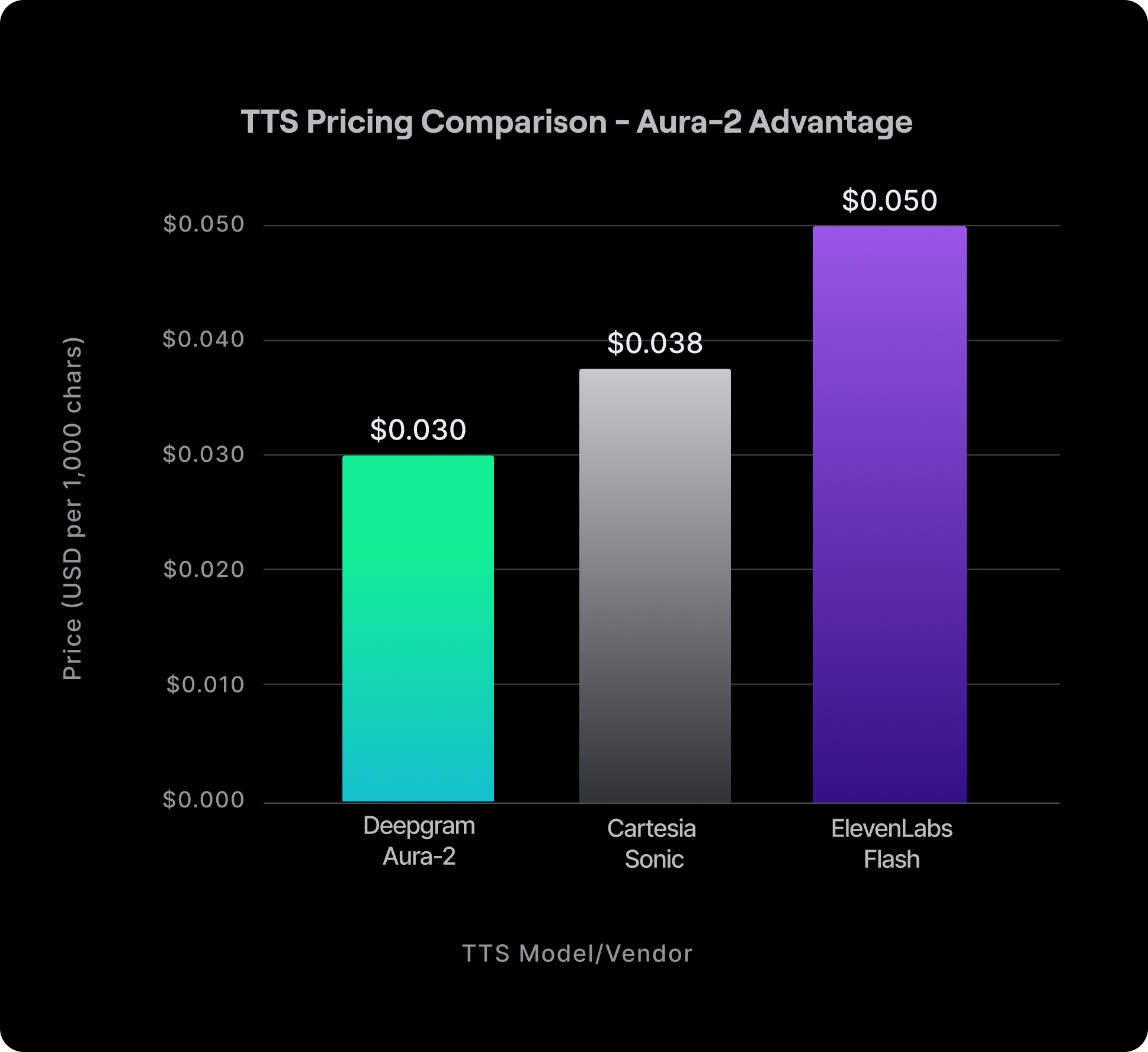
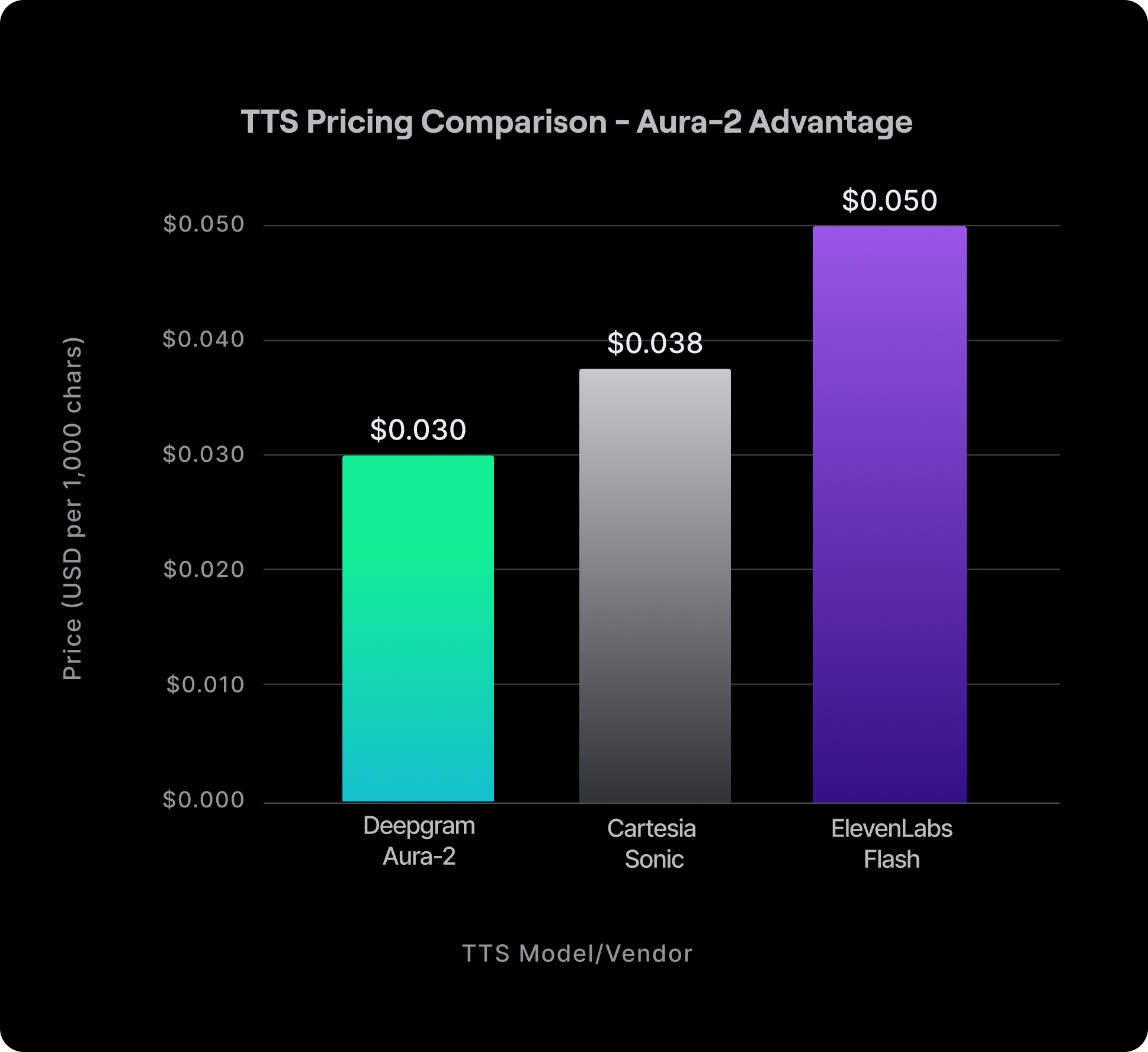
Aura-2 Offers Enterprise-Optimized Text-to-Speech for Real-Time Voice AI
When most people think of text-to-speech, they picture audiobook narration, animated characters, or celebrity voices. That reflects how most TTS models are built—optimized for entertainment use cases, not for the structured, high-throughput demands of enterprise environments. As one enterprise architect we spoke to put it, “Who wouldn’t want to sound a little more like Matthew McConaughey? But the things I care more about are the speech robustness elements.”
Aura-2 was developed to address those enterprise-specific challenges. While entertainment-focused TTS models prioritize style and expressiveness, they often fail in real-time workflows where responsiveness, clarity, and precision matter. They struggle with pronouncing passwords, legal disclaimers, and structured inputs such as dates or alphanumeric identifiers, and frequently introduce latency when scaled across thousands of concurrent sessions.
Aura-2 is different. It is the first text-to-speech model purpose-built for enterprise applications, delivering consistent, reliable performance with the responsiveness, accuracy, and control needed for production-scale voice systems. In environments where clarity and stability are critical, sounding "somewhat human" is not enough.
Key capabilities include:
✅ Context-Aware, Business-Ready Speech – Voices are tuned to match the tone, pacing, and emphasis required in professional and transactional interactions
✅ Domain-Specific Pronunciation – Optimized to handle specialized terminology, numerals, and complex phrasing across sectors such as healthcare, finance, and legal.
✅ Real-Time Responsiveness – Achieves sub-200ms time-to-first-byte (TTFB) for conversationally fluid interactions.
✅ Cost-Efficient at Scale – Delivers premium voice quality and performance at a significantly lower cost per character than competing TTS models.
Aura-2 is available now through the Deepgram API. This blog offers a technical deep dive into what sets Aura-2 apart, from latency and voice design to pronunciation accuracy and deployment architecture.
Aura-2 is Powered by the Deepgram Enterprise Runtime
Most text-to-speech systems available today were designed to prioritize voice quality for offline content, such as audiobooks, narration, or marketing media. These models can sound expressive in controlled settings, but when applied to real-time enterprise use cases like virtual agents or contact center automation, they often fall short. Latency spikes, limited concurrency, and inflexible deployment options become noticeable at scale because these systems weren’t architected for enterprise use cases.
Aura-2 takes a fundamentally different approach. It runs on the Deepgram Enterprise Runtime (DER), the same infrastructure that powers Deepgram’s speech-to-text and speech-to-speech models. DER is purpose-built for high-performance, low-latency voice AI. Rather than treating infrastructure as an afterthought, Deepgram integrates model and runtime development to optimize the full stack for real-time responsiveness, scalability, and operational control.
DER supports features essential to enterprise readiness, including automated model adaptation, hot-swappable deployment with zero downtime, lossless compression for efficient inference, and flexible hosting across public cloud, VPC, or on-prem environments. It is designed to meet the performance and compliance requirements of high-volume, latency-sensitive applications without sacrificing quality or introducing hidden infrastructure tradeoffs.
Natural Text-to-Speech Voices Built for Enterprise, Not Entertainment
In enterprise voice applications, how a system sounds is just as important as what it says. A voice that feels theatrical, robotic, or inconsistent can erode trust and undermine the user experience, especially in high-volume, customer-facing environments. Aura-2 addresses this by delivering natural, clear, and professionally appropriate speech. With over 40 distinct voices, it gives teams the flexibility to align tone and delivery with brand, context, and use case, whether that’s an empathetic support agent, a confident scheduler, or a calm voice assisting a patient.
Most text-to-speech models are trained for entertainment. They prioritize dramatic delivery and emotional range, which works for podcasts or audiobooks but falls short in structured, real-time interactions. We don’t speak to our doctor or bank the same way we narrate a novel, and enterprise-grade TTS should reflect that. Aura-2 is different. Built on Deepgram’s foundation of real-world conversational data, it learns how people actually communicate across industries like healthcare, customer support, and financial services.
Aura-2’s Enterprise Voice Catalog
Aura-2 includes a catalog of more than 40 distinct voices, each developed with a clearly defined tonal profile and specific enterprise use case in mind. Unlike entertainment-focused platforms that prioritize style customization and expressive variety for storytelling and content creation, Deepgram’s approach focuses on aligning each voice with real-world business contexts.
Voice Design Considerations
Each voice is evaluated and refined based on several key factors:
Vocal neutrality: Minimizing overly expressive prosody that might alienate global users.
Tone consistency: Maintaining alignment with brand and context across a wide range of user intents, including apologies, confirmations, and escalations.
Emphasis control: Voices are tuned to naturally highlight important keywords, numbers, and phrases—helping users follow instructions, understand summaries, or act on information without confusion.
How We Tested Text-to-Speech Voice Quality
To evaluate Aura-2 in real-world enterprise scenarios, Deepgram conducted a blinded user preference study designed to assess voice quality across both enterprise and media-style use cases. Human evaluators listened to randomized sets of three audio samples for each prompt and selected the voice they preferred for the scenario based on the appropriate pacing, inflection, clarity, and inherent voice qualities best suited to that particular use-case. Each set could include samples from any combination of vendors, with no guarantees of Aura-2 being present in every round. This format enabled more nuanced comparisons and minimized bias in vendor exposure.
Use cases spanned customer service, IVR, casual chat, interview-style dialog, audiobook narration, and commercial reads. Vendor identities were hidden, and sample order was fully randomized to ensure fairness. Vendors included Azure, Google, ElevenLabs, PlayHT, Cartesia, and OpenAI. In total, the study included 2,794 three-way comparisons, totaling 8,382 audio samples, providing a robust sample size for evaluating across a wide range of TTS outputs.
Aura-2 emerged as the clear preference. In customer service scenarios, one of the most critical enterprise applications, it was chosen nearly 60% of the time on average. While some vendors performed well in more conversational tasks, Aura-2 stood out for its consistency and clarity in structured, high-stakes interactions where pacing and precision matter most.
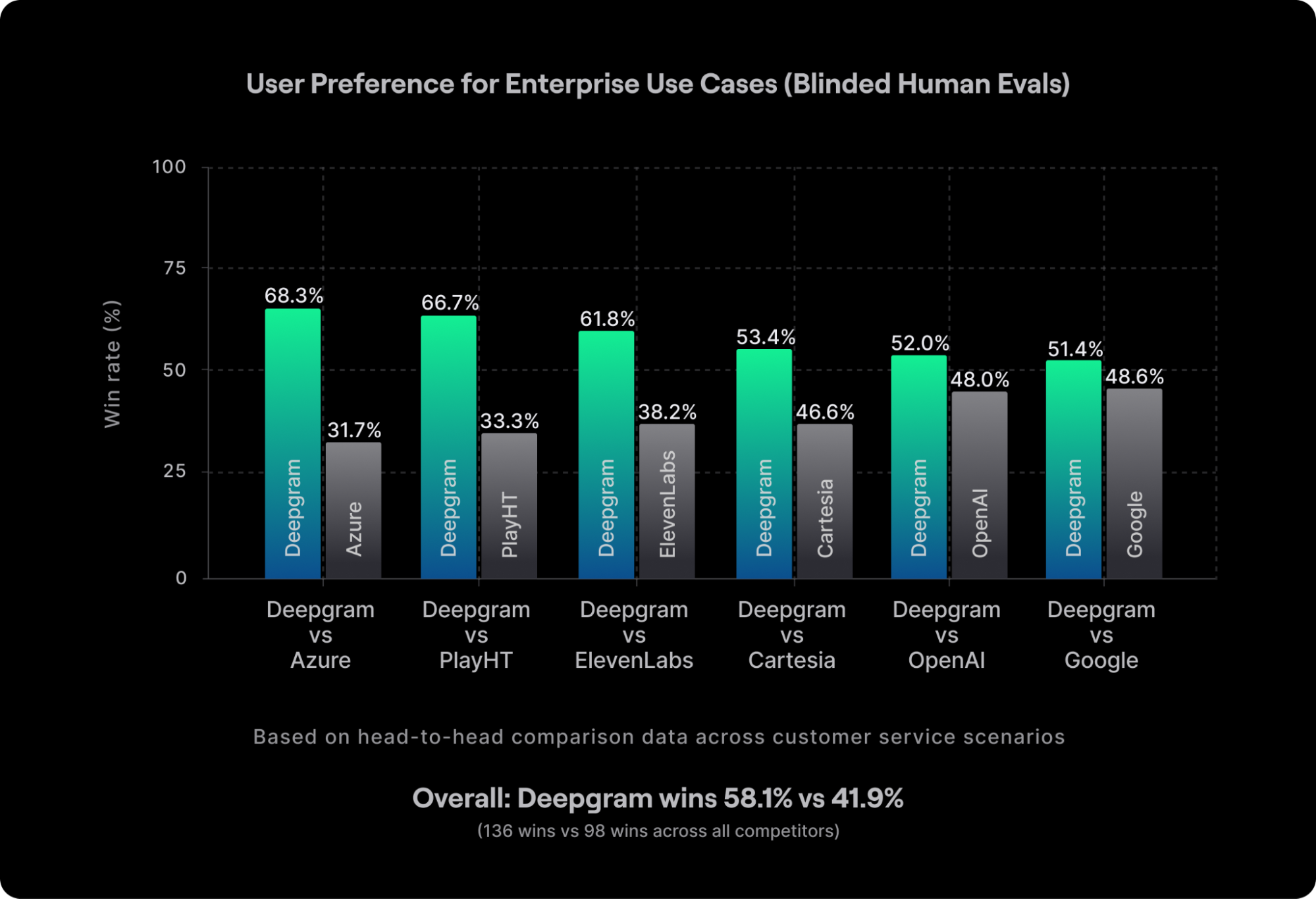
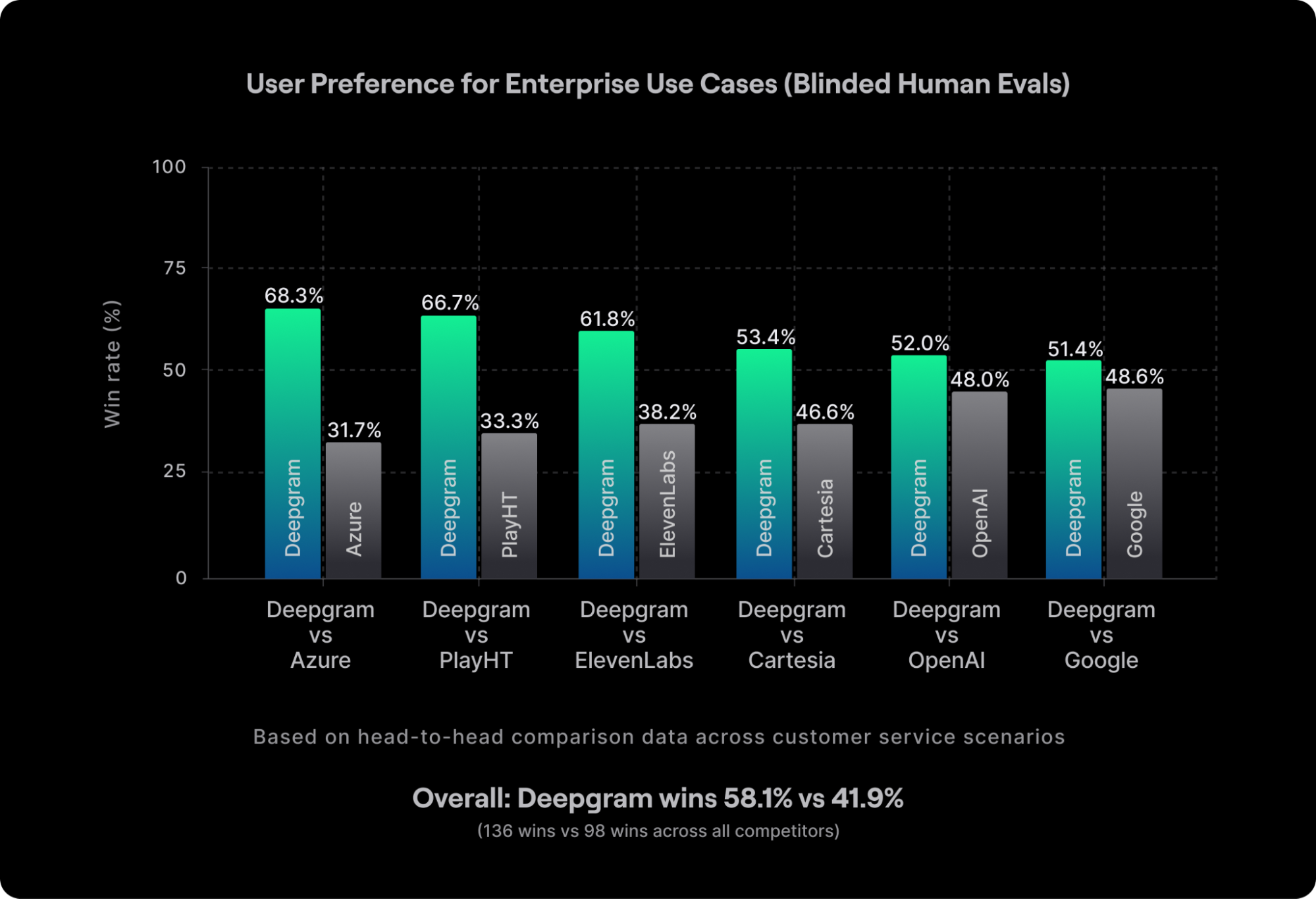
In addition, four of the five most preferred voices for enterprise tasks such as customer support and IVR were from Deepgram’s Aura-2 catalog. This result underscores not only the strength of individual voices, but also the overall consistency of the Aura-2 catalog.
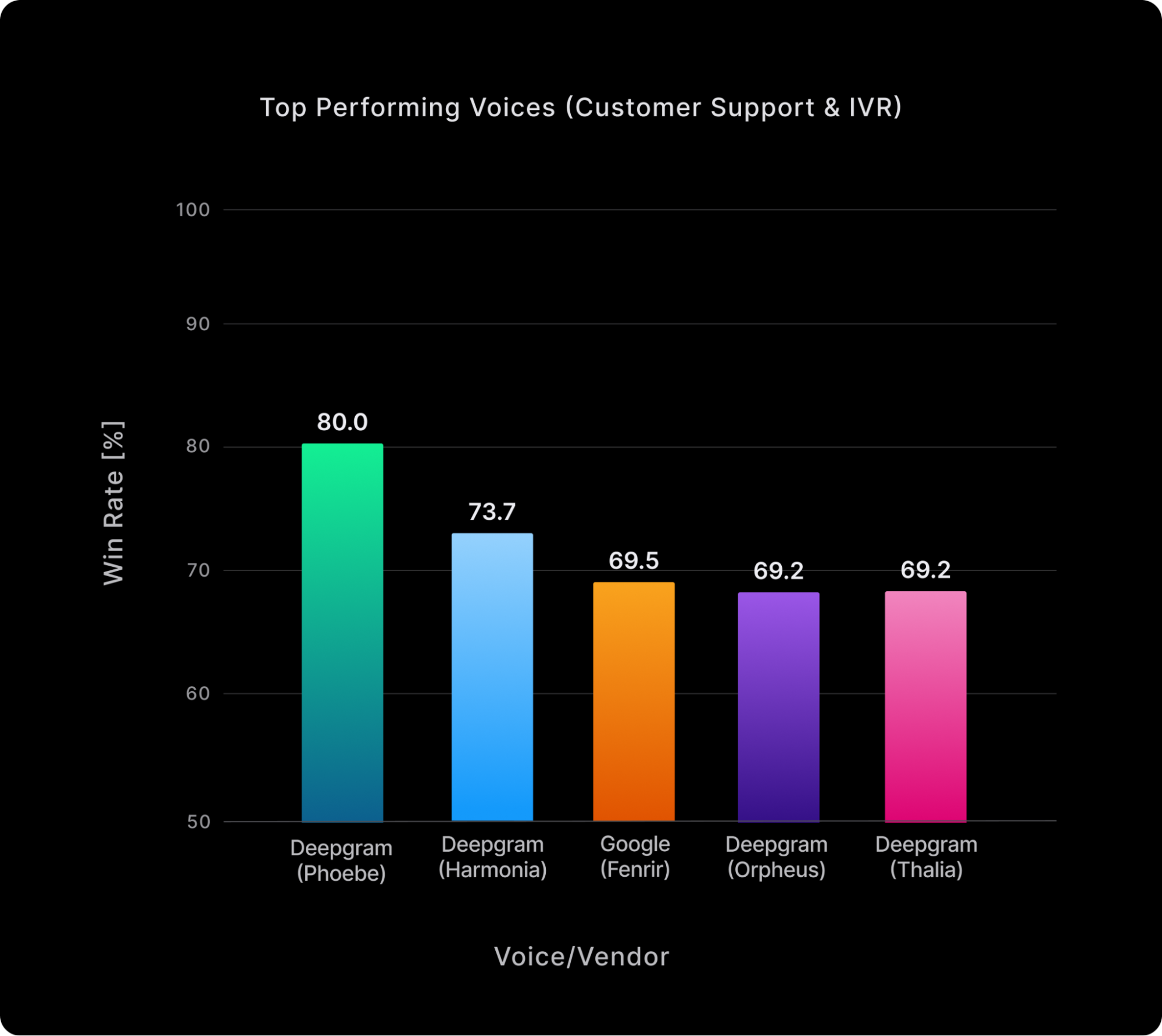
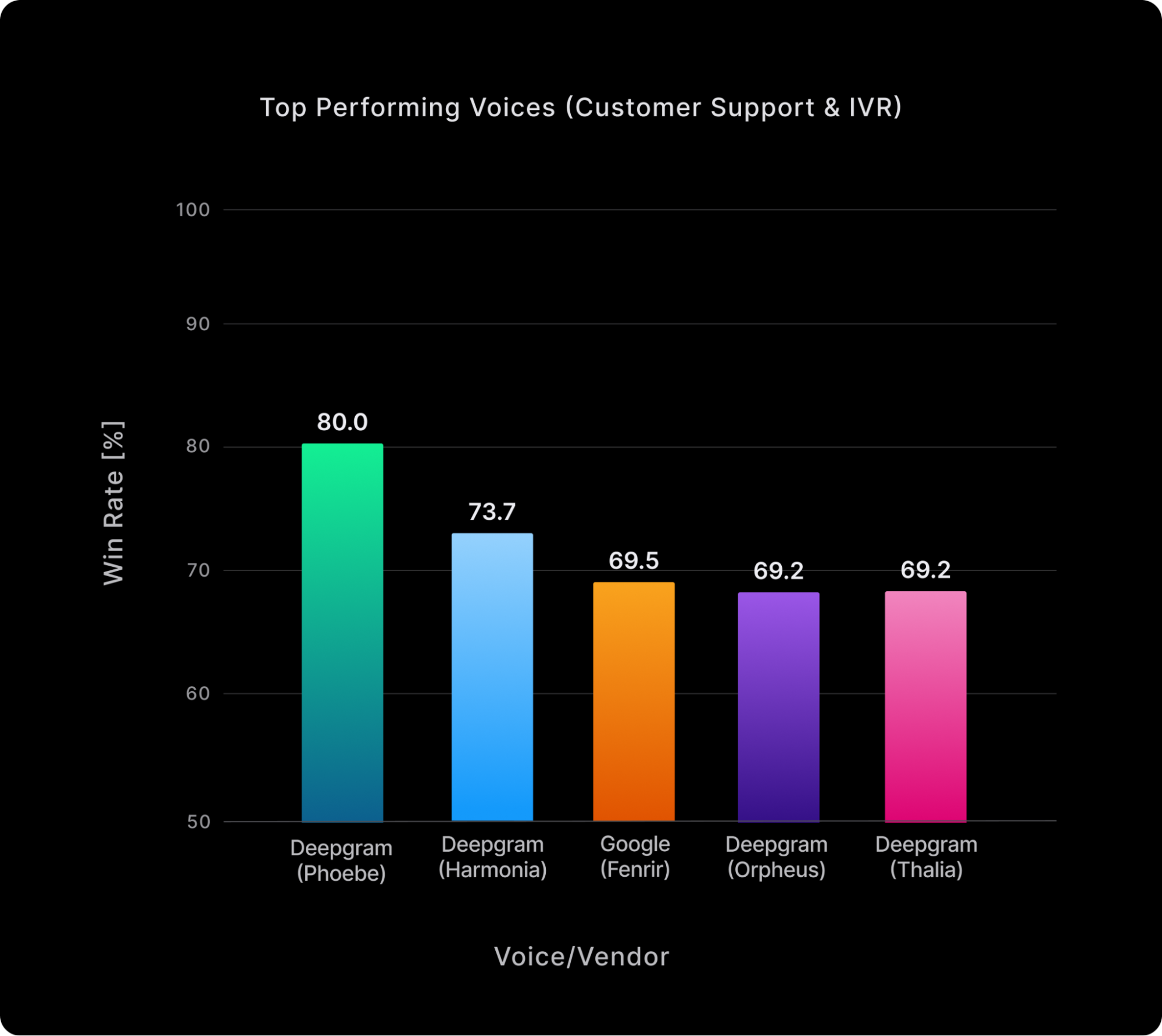
Aura-2 delivers enterprise-grade voice quality through deliberate choices in voice design, training data, and inference behavior. Deepgram focused on voices tuned for specific business contexts, prioritizing tonal consistency and reliability. Trained on enterprise-relevant speech like call center logs and transactional prompts, Aura-2 learns how real conversations flow, including when to pause, emphasize, or maintain formal cadence. Technically, it’s optimized for consistency: controlling loudness, reducing jitter, and pacing structured content naturally, all without needing SSML. The result is speech that sounds professional, predictable, and ready for production.
Precision That Speaks Volumes: Aura-2’s Advantage in Pronunciation Accuracy
In enterprise applications like customer support, IVRs, healthcare automation, and financial services, pronunciation accuracy is critical. Mispronounced drug names, distorted numbers, or garbled email addresses and passwords can lead to costly errors, compliance risks, or a loss of user trust. Aura-2 sets a new standard for handling domain-specific and formatted language. It is built to speak numbers, dates, email addresses, and passwords clearly and consistently, matching how users expect to hear them in real conversations.
By contrast, entertainment-focused TTS models are typically trained on narrative content like audiobooks or character scripts, which lack the structured inputs and specialized terminology common in enterprise settings. As a result, they often mispronounce critical terms or formats. A voice might sound natural, but if it reads “www.deepgram.com” as a garbled phrase or struggles with a word like “atorvastatin,” it breaks the illusion of human-like communication. Aura-2 avoids these pitfalls by being trained on the structured, high-precision language enterprises rely on every day.
Text-to-Speech that Sounds Right the First Time
To evaluate how well Aura-2 performs in these scenarios, Deepgram benchmarked its pronunciation accuracy against the same six TTS providers featured in our voice quality comparison: Azure, Google, ElevenLabs, PlayHT, Cartesia, and OpenAI.
Deepgram’s research team designed a rigorous evaluation focused specifically on enterprise edge cases, inputs commonly found in customer-facing, automated workflows. The test included more than 280 utterances covering:
Currency and numerals (e.g., “$5.7M,” “€14.30,” “1.75 tbsp of sugar”)
Dates and timestamps in varied formats (e.g., “03/11/29,” “2028-3-8,” “10:07 a.m.”)
Email addresses, passwords, and URLs (e.g., “support@help.br,” “P@ssw0rd123”)
Complex addresses and location references (e.g., “119 Pine St, Greenville, MI 31407”)
Each utterance was synthesized using multiple vendors and assessed in pairwise evaluations conducted by Deepgram’s research team. Evaluators used a 4-point qualitative scale—”Unacceptable”, “Needs Improvement”, “Adequate”, and “Good”—to rate each sample based on pronunciation fidelity, appropriate pacing, and the structural clarity of complex phrases.
A pivot-table analysis of all utterances revealed the following “Good” rating percentages:
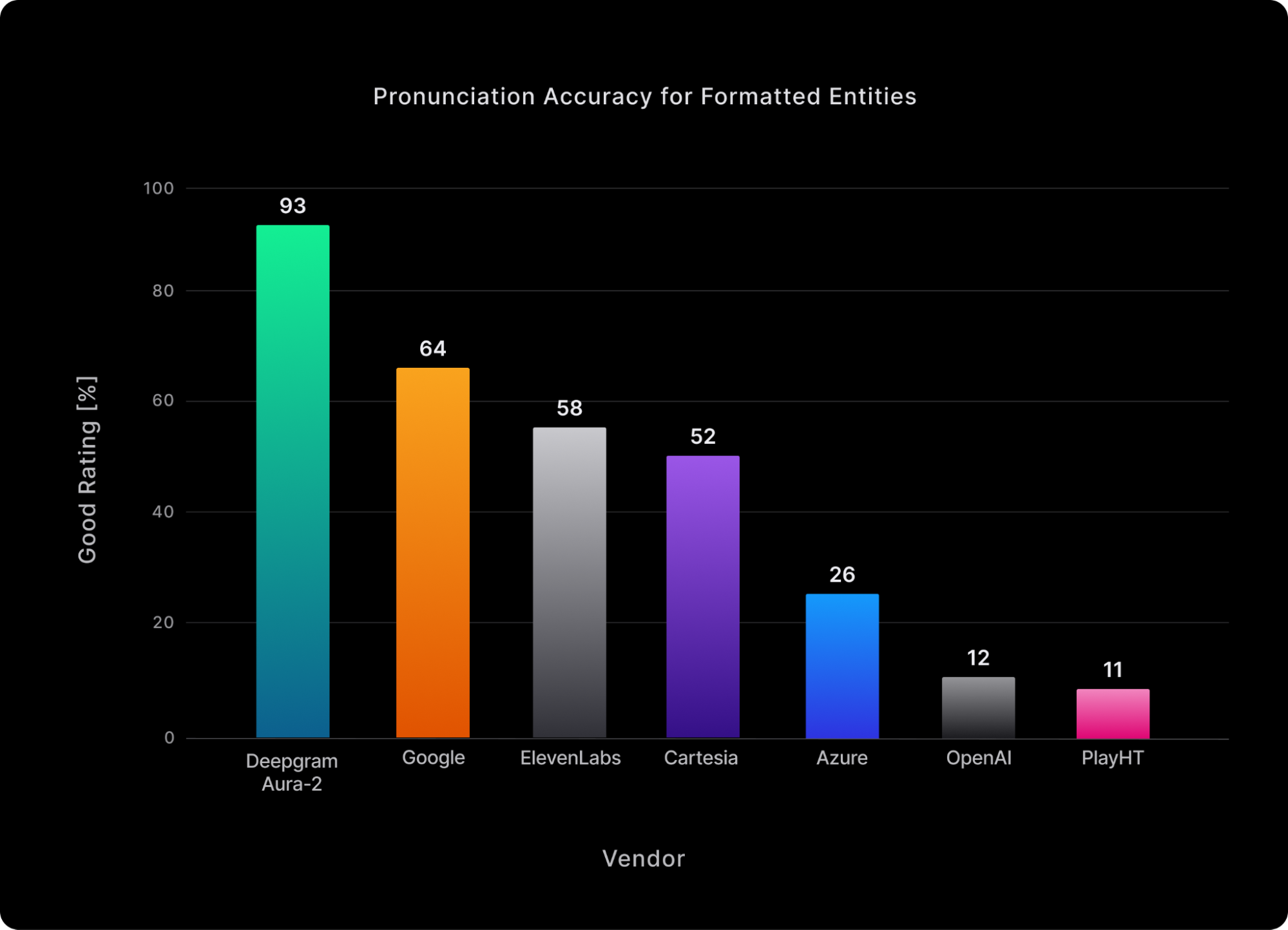
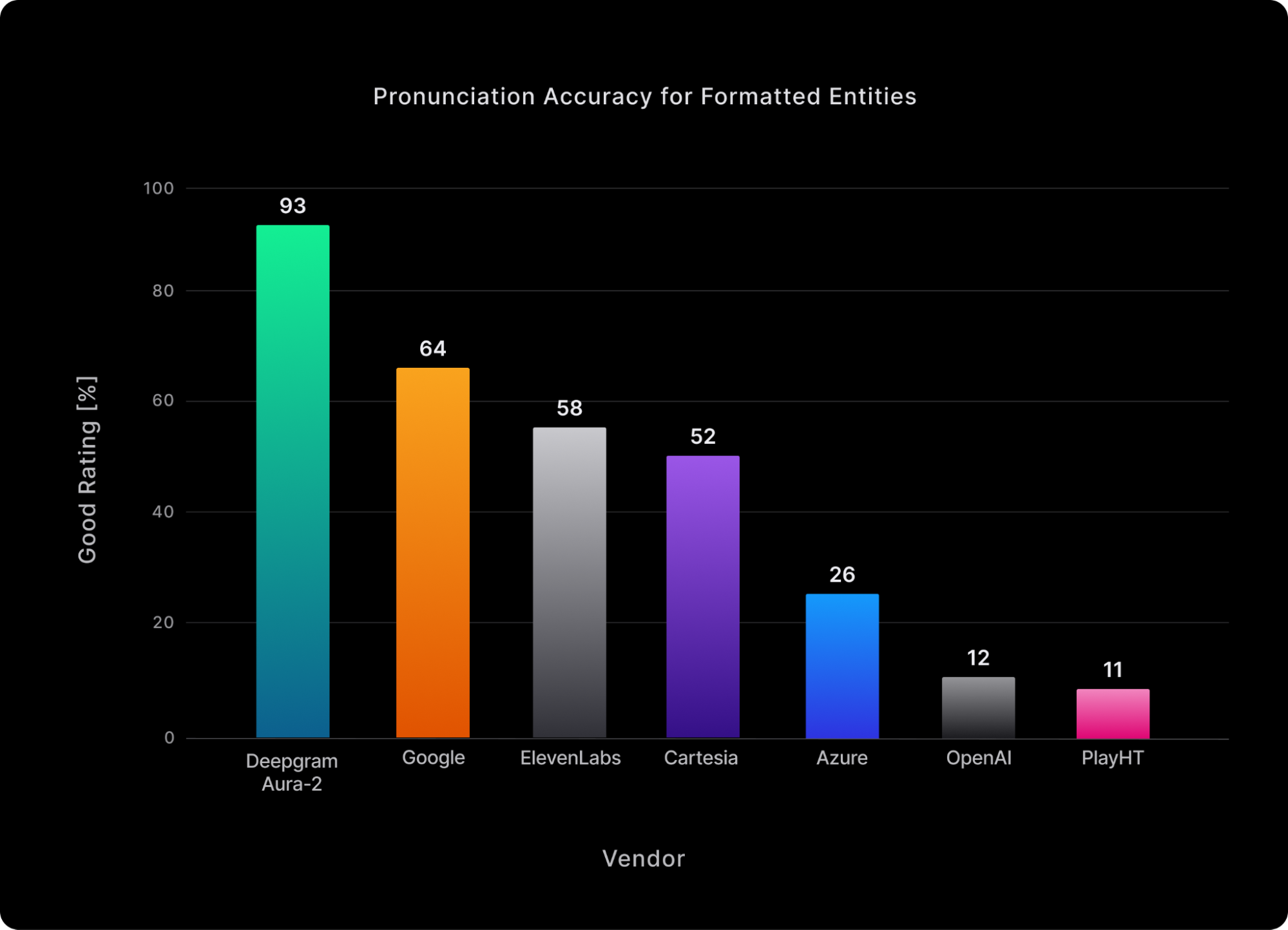
These results demonstrate that Aura-2 doesn’t just sound natural—it sounds correct, particularly in enterprise contexts where precision matters.
To showcase the practical impact of accurate pronunciation, the following examples are drawn directly from the benchmarking study. Each input challenges text-to-speech systems with content that is often mispronounced, poorly paced, or formatted incorrectly by models trained for entertainment.
🎧 Audio Comparison Examples
Input: "Finish by 03:00pm"
Input: "Contact support@help.br, visit airtel.in, or email help@barclays.co.uk."
Input: "The athlete signed a contract for $5.7M."
Input: "The drink costs $2.09."
Input: "A movie ticket costs $7.49."
Input: "608 Birch ter., Fairview, FL 32067."
Input: "269 Elm Pkwy., Lansing, TX 16261."
Input: "119 Pine St, Greenville, MI 31407."
Input: "Enter your password P@ssw0rd123."
Input: "According to the schedule, the maintenance will take 10 hrs and 15 mins to complete."
Although pronunciation errors may seem minor, in practice they introduce costly friction. They can lead to miscommunication in financial transactions, erode trust in automated systems, and reduce the success of self-service flows. Over time, even small errors scale into poor experiences and increased operational costs.
Why Aura-2 Excels at Pronunciation: Adaptability Through Shared Infrastructure
Aura-2’s strength in pronouncing structured, domain-specific language goes beyond training data. It reflects how the system learns and adapts in real enterprise environments.
Because Deepgram’s speech-to-text (STT) and text-to-speech (TTS) systems share the same runtime, Aura-2 benefits from consistent entity handling across the voice pipeline. Terms accurately transcribed by Nova-3, such as dosage instructions, serial numbers, or legal clauses, are reproduced with correct pacing and formatting in Aura-2. For example, if Nova-3 transcribes “AcmeX100” or “500GB” with proper casing and structure, Aura-2 will speak it clearly and naturally, avoiding mismatches that often occur in disconnected STT and TTS stacks.
Aura-2 also improves over time. Deployed in live enterprise environments, the Enterprise Runtime passively learns from real-world usage. As it encounters new terms, such as emerging drug names, product SKUs, or regional address formats, it adapts without manual updates or SSML. By using transcription output as a feedback loop, Aura-2 enhances pronunciation dynamically and remains fluent in the specific language of each business it supports.
Real-Time Performance and Scalability: Meeting the Enterprise Need for Speed
In latency-sensitive environments like real-time voice agents, IVRs, and contact centers, responsiveness is essential. Voice AI must respond within 200 to 300 milliseconds to maintain natural conversational flow. Anything slower can disrupt turn-taking, reduce perceived intelligence, or lower user satisfaction. Aura-2 is built to consistently meet this threshold, delivering sub-200ms performance even at high concurrency and scaling to thousands of simultaneous sessions without compromising voice quality.
This reflects a fundamentally different design philosophy from that of entertainment-focused TTS systems. In media use cases like audiobooks, character voices, or podcasts, audio is typically generated offline, where expressiveness and quality take precedence over speed. These systems often emphasize vocal richness and emotional range at the cost of latency, since timing is less critical. Aura-2, by contrast, is purpose-built for live production environments where real-time responsiveness directly affects interaction quality, task completion, and user trust.
Fastest to Speak, Fastest to Scale
To assess Aura-2’s responsiveness under real-world conditions, Deepgram conducted latency benchmarks against the same six leading providers discussed in the earlier sections, focusing on two core metrics that matter most in real-time voice applications:
Time to First Byte (TTFB): Measures how quickly audio playback begins after a TTS request is submitted.
Real-Time Factor (RTF): Measures how fast the system generates audio relative to its duration.
Aura-2 delivered the best performance in both metrics:
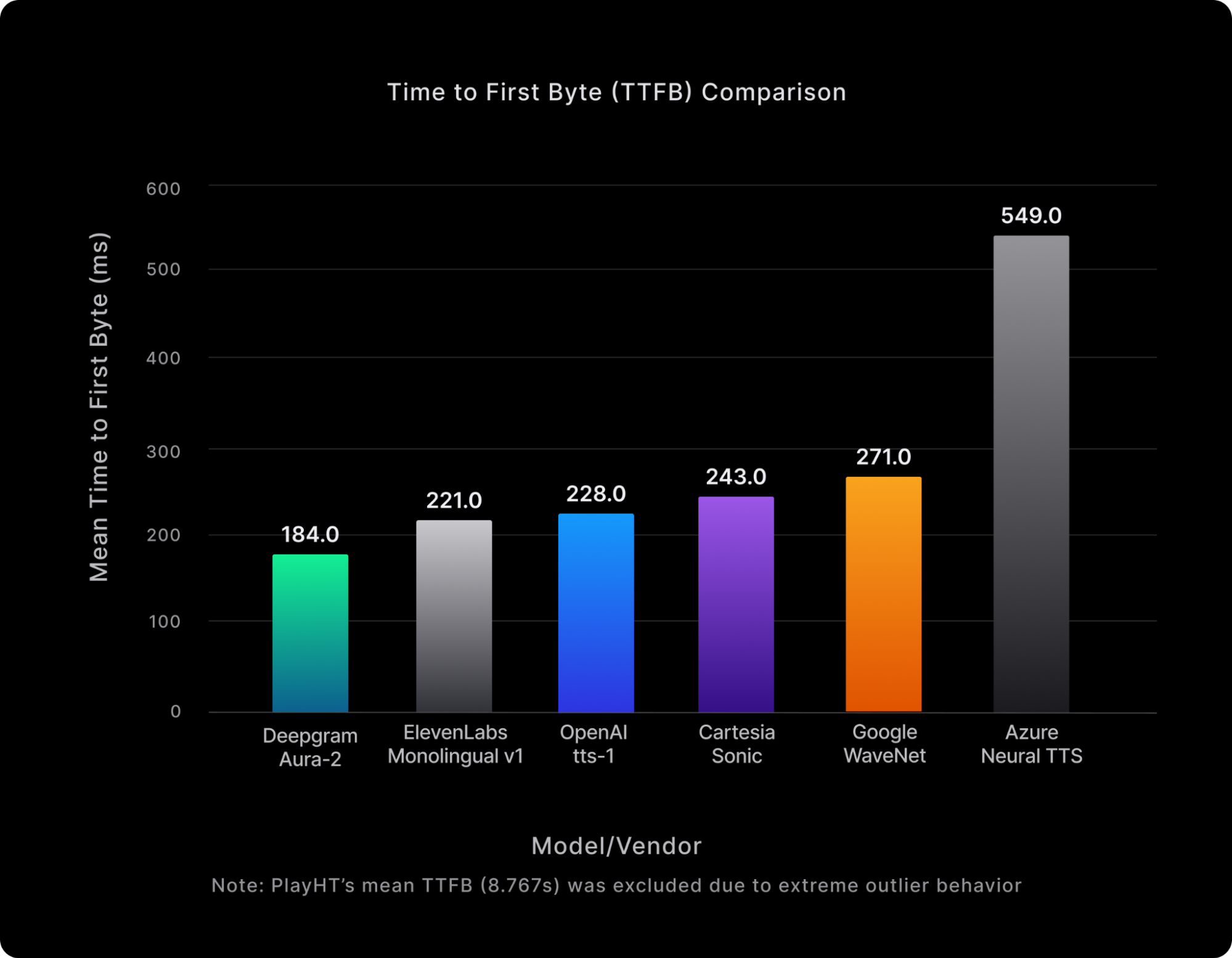
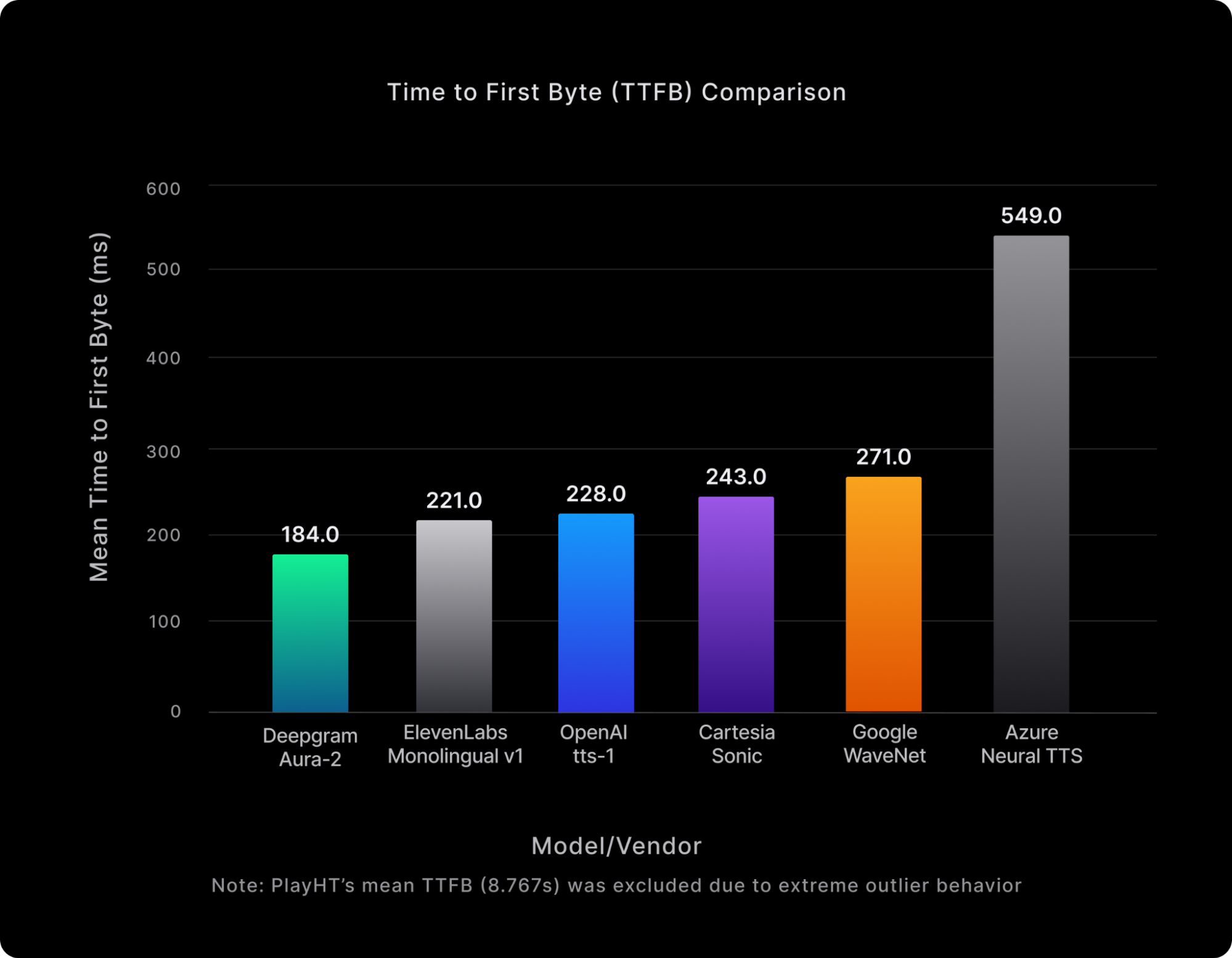
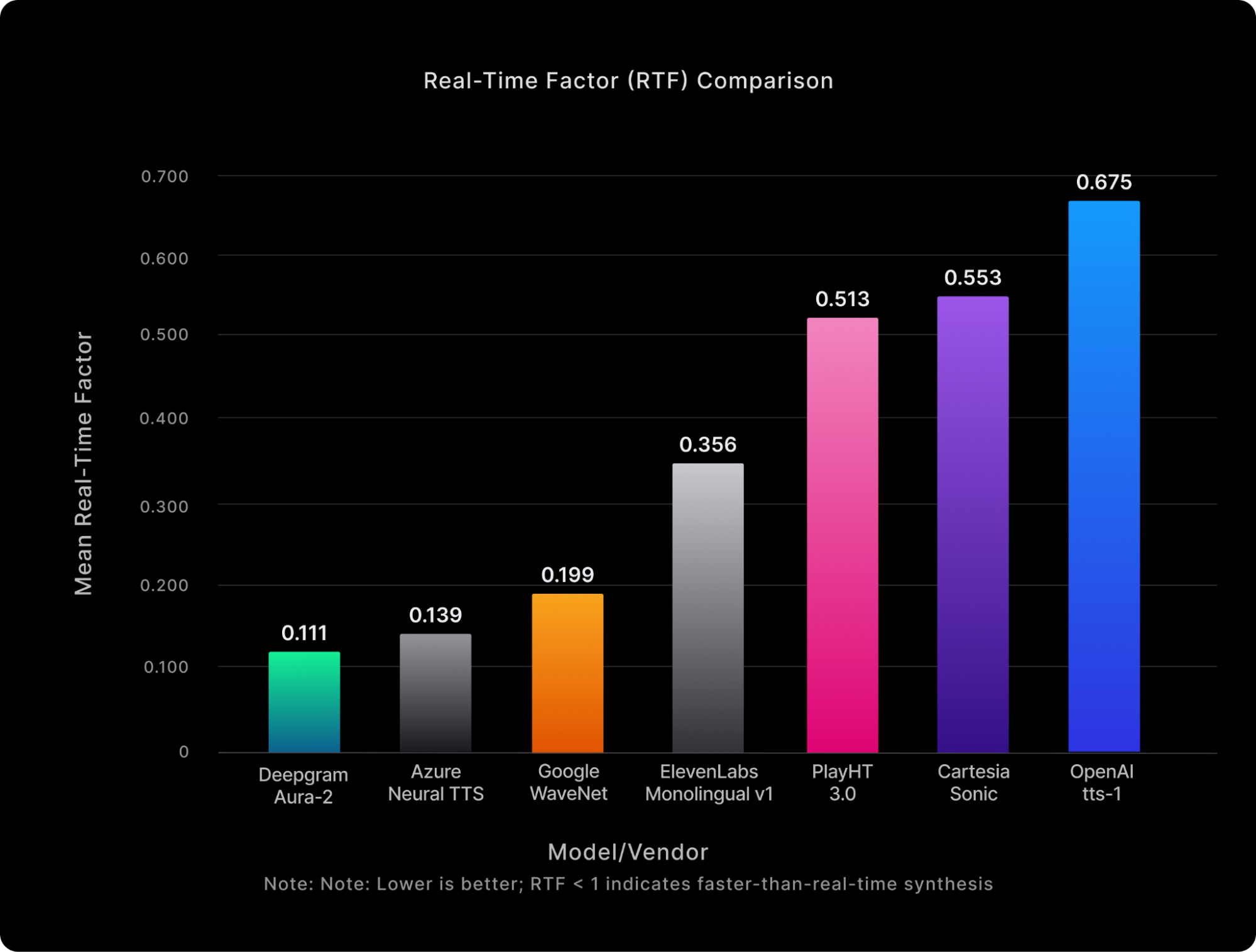
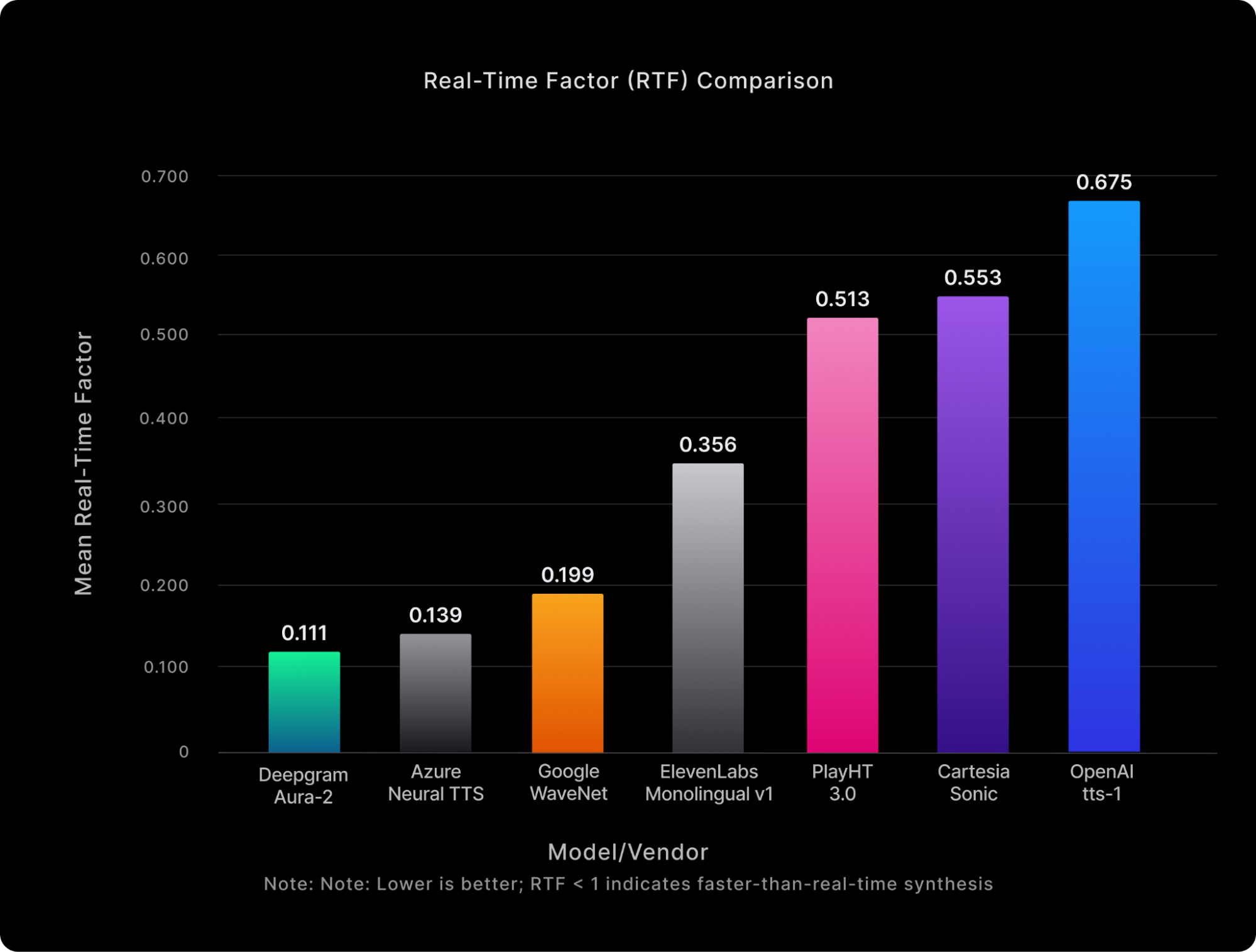
Aura-2 not only leads in average performance, but it also shows minimal variance and low maximum latency. This consistency is especially important for latency-sensitive use cases such as virtual agents, IVRs, and real-time assistants. While other vendors exhibited delays under load, Deepgram consistently stayed under the 200-millisecond threshold that is often cited as necessary for natural conversational flow.
With a Real-Time Factor of 0.111x, Aura-2 can synthesize one second of audio in just over 100 milliseconds. This speed allows developers to pre-buffer full phrases and eliminate perceptible pauses, enabling responsive and human-like voice interactions.
Note: Benchmarks reflect each provider’s default or most widely available high-quality model. Some vendors offer lower-latency variants (e.g., ElevenLabs Flash), but these often come with plan restrictions, reduced voice options, or trade-offs in naturalness.
Technical Drivers Behind Aura-2’s Real-Time Performance
Aura-2’s ability to deliver consistently low-latency, high-throughput performance stems from architectural decisions that prioritize real-time responsiveness and operational efficiency.
At the core is Deepgram’s streaming-first Enterprise Runtime, which uses GPU-accelerated inference and optimized models with quantization and pruning to reduce memory and compute load. This enables faster execution and supports high concurrency without introducing bottlenecks. Latency is minimized not just by fast inference, but by architectural design: audio begins streaming as soon as synthesis starts, without waiting for full generation. The shared infrastructure between STT and TTS eliminates delays from serialization, inter-service calls, or redundant API hops.
Deepgram’s runtime is also deployable into your own infrastructure, whether in a VPC, private cloud, or on bare metal. This allows inference to run close to the application layer, significantly reducing roundtrip latency and giving enterprises more control over performance, compliance, and data locality.
The runtime is stateless by default, enabling distributed workloads to be orchestrated with minimal coordination. Requests are routed efficiently across compute nodes or containers, and additional capacity can be spun up rapidly to absorb demand spikes. Because concurrency is governed by compute throughput rather than memory constraints, Deepgram maintains low-latency service even under heavy load.
Cost-Efficiency at Scale: Text-to-Speech Without the Premium Price Tag
When you're handling millions of TTS requests, cost isn’t an afterthought. It shapes what you can build and how far you can scale. Aura-2 delivers high fidelity and low latency without compromising on cost-efficiency, making it a strong fit for enterprise-grade voice applications.
Aura-2 is priced at $0.030 per 1,000 characters, making it more cost-effective than comparable text-to-speech offerings in the market. As shown in the pricing comparison chart, Aura-2 delivers a more affordable per-character rate than Cartesia Sonic ($0.038) and ElevenLabs Flash ($0.050), despite meeting or exceeding both in latency and voice quality performance for enterprise use cases.
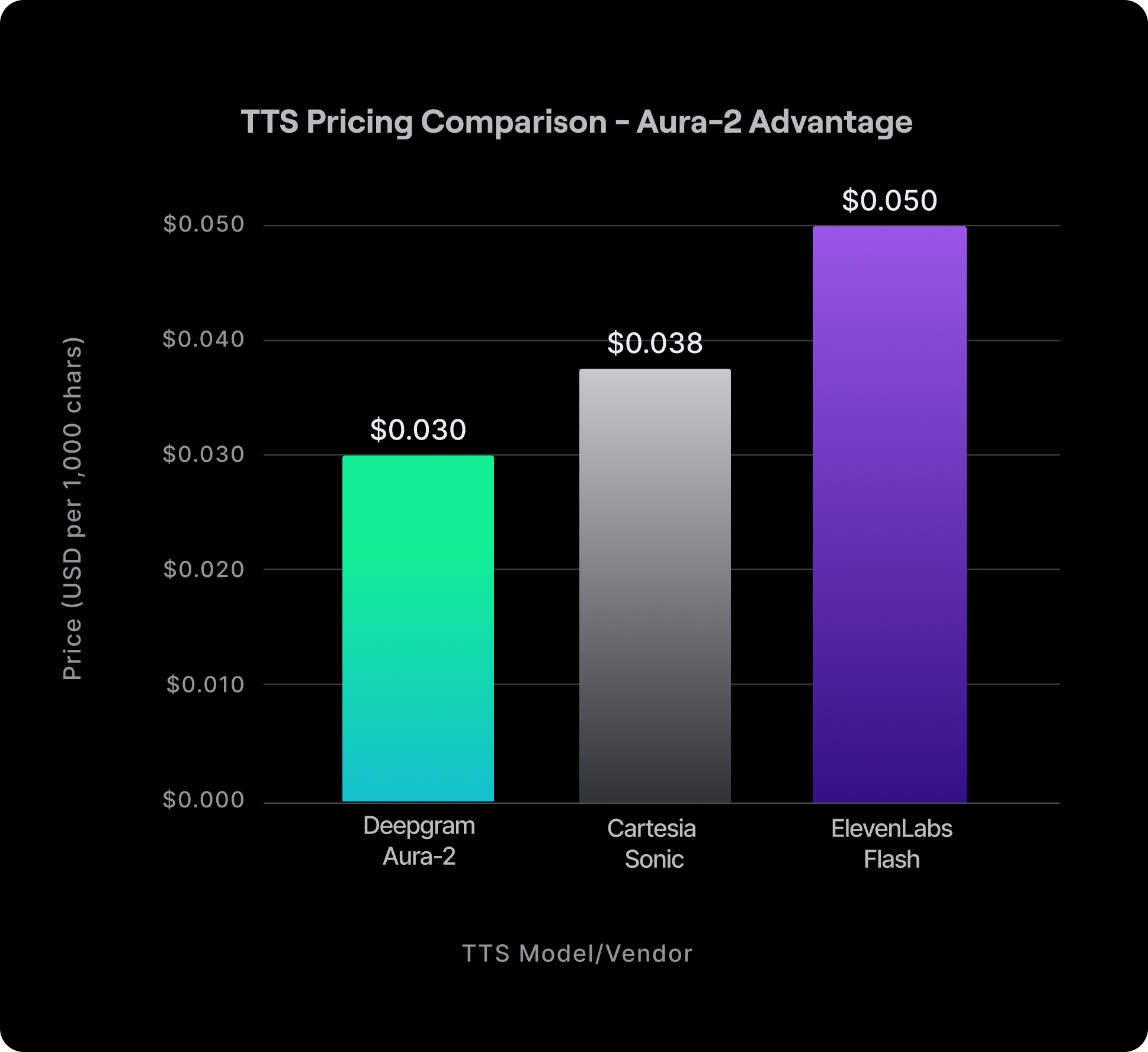
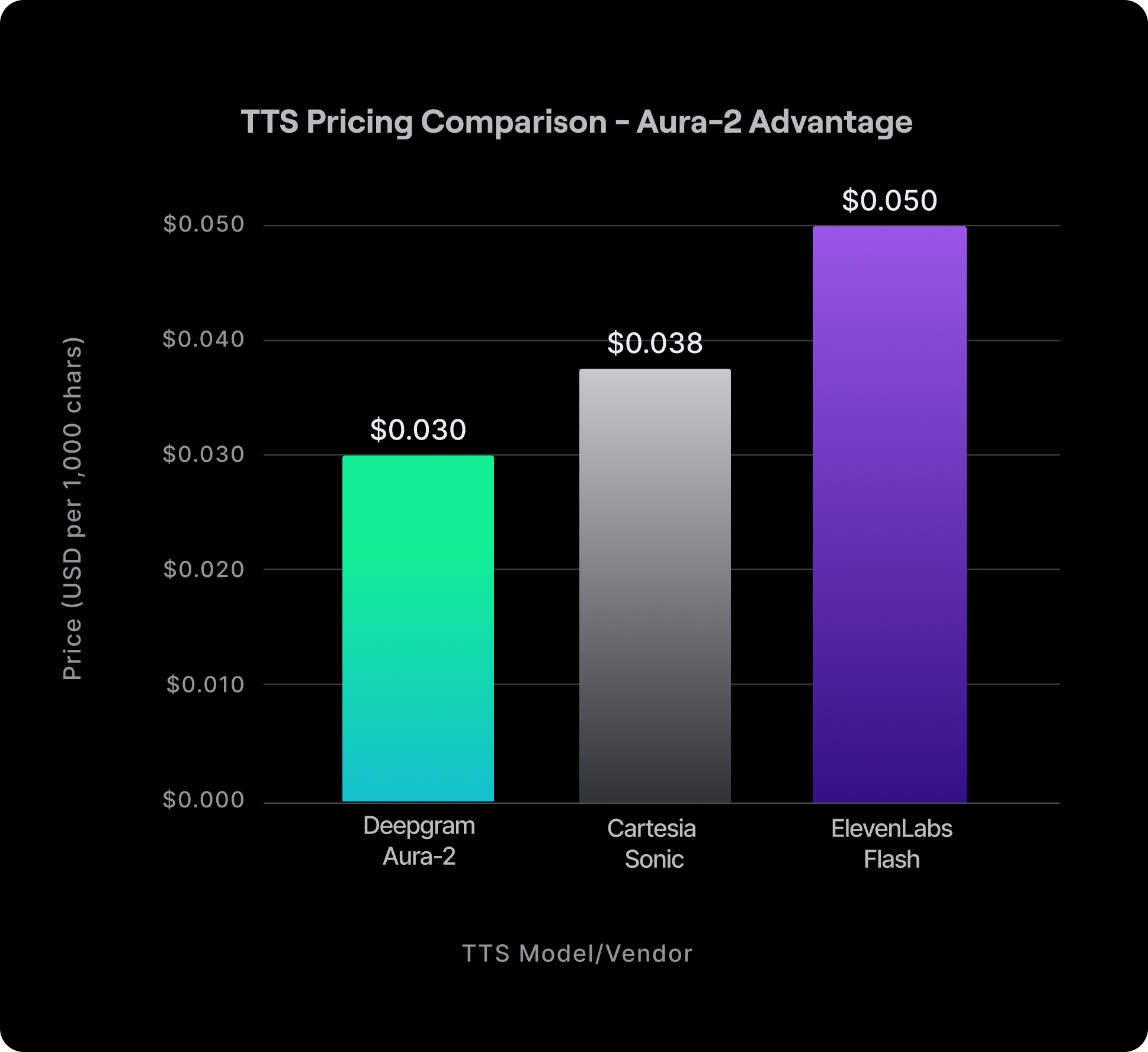
Many vendors use tiered pricing that changes based on latency, voice quality, or access to features like voice cloning and real-time streaming. For example, ElevenLabs' more expressive or low-latency models are limited to higher subscription tiers, with some features only available on business plans. This complicates cost planning and can drive up total cost of ownership (TCO) for enterprise-scale deployments.
These pricing models reflect a different design philosophy. Vendors focused on entertainment use cases optimize for expressiveness over scalability. Their economics are built around small-batch, offline generation, not always-on, high-concurrency workloads.
Deepgram also offers volume discounts for committed usage, enabling large enterprises to secure significantly lower effective rates as usage grows. For teams deploying at scale, cloud egress fees can become a significant and often overlooked cost. Deepgram’s support for VPC and on-prem deployment helps eliminate this expense entirely. This gives you full control over infrastructure and better predictability in total cost of ownership.
What Enables Deepgram’s Text-to-Speech Cost Advantage
Aura-2’s cost-efficiency isn’t a tradeoff; it’s the result of engineering for optimization at every layer. As discussed earlier, Deepgram’s Enterprise Runtime is built for high-throughput processing and concurrency, minimizing compute per request through techniques like quantization, pruning, and precision tuning. This allows more TTS jobs to run simultaneously on the same hardware, lowering the baseline cost of infrastructure.
These efficiencies are especially valuable in streaming scenarios, where real-time performance often comes with increased compute overhead. Aura-2 avoids that tradeoff by delivering low-latency synthesis without high resource usage. Deepgram’s extreme compression also reduces transmission costs and bandwidth requirements, which is particularly helpful in high-volume or long-form use cases.
Deepgram's unified runtime also simplifies operational management. Enterprises do not need to provision and scale separate infrastructure for STT and TTS, or maintain duplicate orchestration logic and compute environments. Fewer moving parts lead to lower operational risk, easier deployment, and a lower total cost of ownership.
Try Aura-2 for Yourself
Aura-2 represents more than just a step forward in voice synthesis. It marks a shift in how enterprise-grade text-to-speech is built, evaluated, and deployed. From consistent pronunciation of structured data to real-time responsiveness under load, Aura-2 is engineered for production environments where clarity, reliability, and scalability are non-negotiable.
If you're building real-time agents, automated workflows, or voice-first applications, now is the time to test what enterprise-ready TTS should sound like. Aura-2 is available via API with full documentation and a self-serve playground to help you get started quickly.
➡ Explore the API Documentation
➡ Try Aura-2 in the Deepgram Playground
➡ Sign up and get $200 in free credits — enough for over 13 million characters of synthesis.
Unlock language AI at scale with an API call.
Get conversational intelligence with transcription and understanding on the world's best speech AI platform.