AIMinds #034 | Richard Meng, CEO & Co-founder at Roe AI
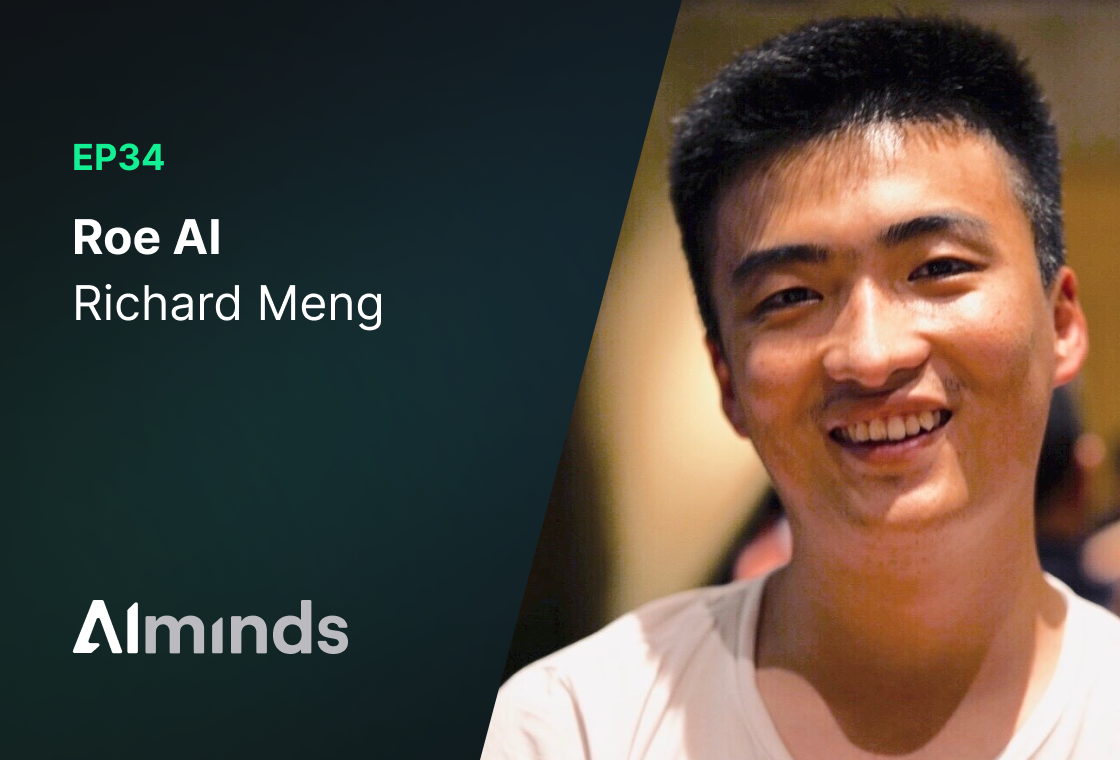
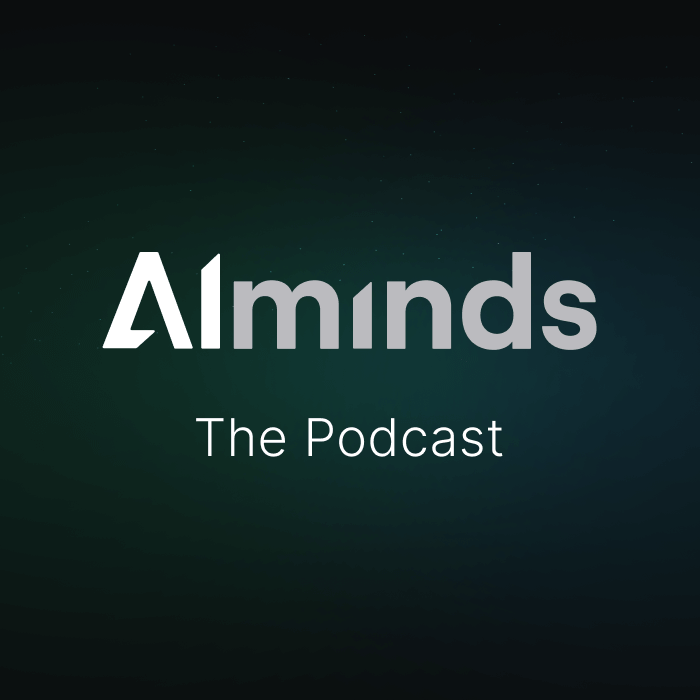
About this episode
Richard is the CEO and Co-founder of Roe AI, a SF-based startup currently focusing on enabling data practitioners in financial services to analyze unstructured data with simple SQL queries. The team is funded by Y Combinator, Google AI ventures, Ardent ventures and key execs from Snowflake.
Prior to Roe AI, Richard was the tech lead in Snowflake Gen AI. Prior to Snowflake, Richard led the Skills and Knowledge Graph related products at LinkedIn.
Listen to the episode on Spotify, Apple Podcast, Podcast addicts, Castbox. You can also watch this episode on YouTube.
In this episode, Demetrios sits down with Richard Meng, the co-founder and CEO of Roe AI, a pioneering startup based in San Francisco. Roe AI, supported by Y Combinator, Google AI Ventures, and Ardent Ventures, is revolutionizing the way data practitioners in financial services interact with unstructured data using intuitive SQL queries.
Richard's journey from growing up in an entrepreneurial environment in China to his roles at LinkedIn and Snowflake provides a rich backdrop for this conversation. He shares his experiences working on innovative projects in Gen AI and natural language processing, which culminated in his creation of a groundbreaking tool during a Snowflake hackathon. This tool allows non-technical users to interact with data through natural language, simplifying complex data operations.
The episode dives into the challenges and solutions of managing unstructured data in business environments and explores how Roe AI is leveraging AI technologies to empower data analysts. Richard's insights offer a comprehensive look at how his startup is transforming data accessibility and utility in today’s data-driven world.
Fun Fact: During a weekend hackathon shortly after joining Snowflake, Richard developed a tool using ChatGPT that allowed for natural language querying within Snowflake's data warehouse. This innovation significantly modified the way users interact with their data, emphasizing simplicity and accessibility.
Show Notes:
00:00 Learned a lot at LinkedIn, seeking change.
04:00 Joined Snowflake, built chat GPT prototype, 2022.
08:19 Empowered non-technical users to independently manage data.
12:05 Snowflake excels at innovation.
15:37 Unstructured data processing is currently too complex.
18:49 Optimizing data extraction from unstructured sources like S3.
22:14 Competitor analysis through web pages and operational data.
23:26 Customized ERP data tool analyzes insightful transcripts.
More Quotes from [Speaker]:
Transcript:
Demetrios:
Welcome back to the AI Minds podcast. This is a podcast where we explore the companies of tomorrow built AI. First, I am your host, Demetrios. And this episode, like every other episode, is brought to you by Deepgram, the number one text to speech and speech to text API on the Internet today. Trusted by the world's top conversational AI leaders, startups, and enterprises like Spotify, Twilio, NASA, and Citibank. We are joined today by my man, Richard, the co founder and CEO of Roe AI, an unstructured data analytics platform. How you doing, Richard?
Richard Meng:
Doing great. Thanks for having me, Demetrios.
Demetrios:
So you grew up in China. You came to the US when you started doing your undergrad at UC Berkeley, but your dad was an entrepreneur himself. What did you take away? I understand that he was always dealing with Americans when he was doing business. So you learned business at a young age. And osmosis, what were some of the things that you were learning?
Richard Meng:
So he started trading. He owns a trading company. He started trading with the metal parts, the iron steels with Americans since 1995. Back then, Internet was just become the thing. E commerce would just become the thing. Right. So all he was doing is sitting in front of his computer and start sending outbound emails, probably. I think at that time, outbound email effective rate is like 100 times more than this is today.
Richard Meng:
But I think what I learned from him is that spirit of being a startup founder going from scratch from zero to one. That spirit really encourages me.
Demetrios:
He had that hustle, and it probably helped that, yeah, sending outbound emails was like shooting fish out of a barrel. So you were at UC Berkeley, you graduated, you went on to work at LinkedIn as your first job, working on recommender systems for the recruiting services. And as I understand it, you grew in your job there, but you felt like it wasn't enough. What made you want to transition out and go start a new adventure?
Richard Meng:
So I had a great time at LinkedIn, and it's my first job after I graduated. And I honestly learned a ton from the people around me. And, you know, especially at a later point, my. The people around me at LinkedIn all become the entrepreneurs themselves. So that gives me an impulse, like a really strong impulse for going out of the world, going out on a yemenite outer world. I know it's. I'm very comfortable, and I'm doing my best, and I believe I'll be even going higher if I stay at LinkedIn. But I think it's just that impulse of change that makes me to, you know, go into some other places, maybe a faster moving place and try out something more adventurous.
Richard Meng:
Right? That makes me push to go join Snowflake and which is one of the most fast growing data warehouse company in the world.
Demetrios:
Is it? So there's a fun story about you at Snowflake, and something you created in a hackathon turned into an excuse for you to go and talk with 30 vps. Give me that story.
Richard Meng:
So, yeah, so I joined Snowflake in 2022, October, and by the time I joined, it was probably at the dawn of chip coming out. You know, one, one weekend. I always remember it was a winter cold winter in Bay area, right? I did not do anything, but I was just one idea came to my mind is, why not we build something, some chat GPT for snowflake? And that was, I think it was December 2022. So I built it in a weekend. I thought I should, you know, not to be the only one who can use it. So I presented to my skip manager. She really liked it. I think she gave me that first burning fuel into what I'm doing, and she encouraged me to keep pushing it and even referred me to her boss.
Richard Meng:
So after a couple of weeks, you know, do some tweaks to my little prototype present to her boss. Turns out he really liked it as well, and he referred me to his boss as well. And that is the SVP of Snowflake, SAP engineer of Snowflake. So we organized a big meeting. I was probably like 2030 people sitting in a room, and I was sitting at the edge of that short edge of that long table, giving up presentation. And I remember that was the first time that I got a stomach pain after I delivered the speech. That was so nervous. That was nerve pain, because I was so nervous.
Richard Meng:
But, and all I remember was that people start to discussing after, before the, like ten minutes or like five minutes before my demo finishes. I can tell how people are excited about it. And later that demo turned into very actionable sprints all the way to like, from February all the way to the Snowflake summit in 2023 in June in Las Vegas. So we worked, we crushed that four month bring multiple teams together within Snowflake, including Streamlit, including native app, and including our partners, like Cybersynovich data, produce high quality data, even land chain, to help build some part of the ecosystem. And we kind of delivered a massive talk in June and showed the world, like, how can you integrate the Gen AI within your snowflake data warehouse? That was a big blast.
Demetrios:
So that I'm clear and for everybody else let me see if I understand correctly, because Snowflake is a data warehouse. So you put in structured data, or you put in Excel spreadsheets with a lot of numbers and columns and rows and all that fun stuff. So it's structured, and what you created in this weekend is the ability to talk to it in natural language. So you no longer need to use this programming language, which is probably SQL. I would imagine some people might use Python. Can you use Python? Yeah. So the idea is that you no longer need to worry about any of that. If you don't know how to program in SQL, you're all good.
Demetrios:
Just say what you want from the data warehouse and Snowflake is going to be able to now understand that and retrieve it for you. Is that what you hacked together?
Richard Meng:
That's exactly correct. Yeah, what I hacked together was exactly that. But also it's like a command. It's like a natural language as a command line for a snowflake. It could write SQL query, execute the SQL query, retrieve the data together, retrieve the data back, and not only that, you can also tell Snowflake to create a worksheet, create a new dashboard. So that was just natural language on everything. Hackathon I did.
Demetrios:
I see. So there was actions that you could take. It wasn't just, hey, give me this data, or how many sales have we made? It was all right, I want to know how many sales we made. And now I want to know. Let's put all these sales of the different branches of our company into a new database or a new worksheet, or whatever it may be, so that I can get a better idea of sales across the organization or whatever your use cases, you no longer needed the SQL mastery. And sometimes people have to lean on their data teams in order to get that information. Or if they want dashboards or if they want to update things, they have to ask a data engineer or a data analyst. And you were able to help empower the non technical users to get in there and get their hands dirty with the data.
Demetrios:
On the technical level, it was just a chat GPT call. Was that what you were doing there? And then integrate a lot of integrations with Snowflake. So when you would, and probably a lot of, a lot of regex in case something you had hard coded, I.
Richard Meng:
Think you're spot on. Demetrios, remember that was like late 2022. We only have GPT-3 right? We don't have a JSON rigid output. The line chain ecosystem is not, it's not even built that, like built yet we don't have streamlet as an interface to talk. Right. So I got to build all those components together into. I remember that was, I used gcps as a backend, essentially. I literally hosted on a little firebase database and firebase front end.
Richard Meng:
So that was fun. So I put together the chat interface. I do leveraged a little bit of a react agent paradigm in Landchain back then. It was very early for them as well, so need to do a lot of regex, of course, to make sure the output is programmable by the computer. But yeah, that was all it takes.
Demetrios:
And you gave this talk at the Snowflake summit, which has tens of thousands of people. You said your stomach hurt when you were presenting for the vps. Was your stomach hurting again when you gave the talk in front of all those people?
Richard Meng:
My hands get cold. I don't think I got another stockbroker pain. Yeah, my edge line was so high at that moment when I start, like literally, you know, start well before I start presenting. Got onto the podium, I remember there was a guy, I forgot his name, but he was like holding, like holding my fist. That was the first time I heard his fist is so warm and that gives me a sense of calm, like about like 10 seconds before I go to the podium, hold my fist for like 5 seconds and close your eyes. Deep breath. And here you go. Yeah, and welcome to the podium.
Richard Meng:
I see the big counter where audience could not see it. That is going to start counting down from like ten or 15 minutes. And I started my. It's a live, like, programming session as well, so I have to talk while I program.
Demetrios:
You are brave to try and live demo in front of all those people. That is incredible. So now you could have been great there. The snowflake decided to invest a lot of money into Genai. It seems like you were really leading the organization to help them think about it differently. Why didn't you just stay?
Richard Meng:
I think Snowflake is probably, for everyone, is probably one of the best place to innovate if you want, especially if you want to build Gen AI. And I think it will keep being so for the next five years, at least five to ten years, and we buy a lot of GPU's, we hire the best team in the world, including the team from Neva and Deepspeed, which has arguably one of the best researchers on the AI domain. So I think, and if I stay there, I believe I'll keep pushing it just how I did at LinkedIn. But I think the reason I came out is that the seed of building a startup has always embedded in my chest. And I think what ends up pushing me is I saw an even bigger future for the unstructured data. And many people, many data practitioners know Snowflake or in general, data warehouse. Data lake houses, including databricks, are a place for, are mostly a place for the structured data we talk about. Delta Lake would talk about iceberg parquet files all the time, but at the end they are just like more fancy CSV files with more time travel and more compressions.
Richard Meng:
But if we're talking about unstructured data, what does it mean to people? Human as human? We process unstructured data all the time. We process like 74gb of unstructured data. According to a report, those are the information processed through our eyes and through our noses and I. But computer could not process them because computer processed one and zero at its best. But LLM transformer based model came out. All of the data in front of him is just like tokens, including a picture. It's a token, it's one and a zero for the transformer models. I see a bright future where data teams can now process, can now have that extended, like eyes and ears, just like human, and they can process all kinds of unstructured data that they were not able to in the last decades, then decided to do something.
Richard Meng:
Well, I guess do something to get skin. The game is at least do it full time. We applied y combinator together with my good friend in New Zealand, Berkeley, Jason, and we got it, fortunately. So that becomes the last pull out of the company. We decided to quit our job full time, cut a golden handcuff, and start something new.
Demetrios:
It's incredible, because I'm sure they were enticing you with all these great teams, all these GPU's, all this freedom to do whatever you needed to do, and you still had the conviction to say, I gotta go and try this on my own. And so now that gives me a clear picture of what the inspiration was. But what is the pain that you're trying to solve with the new product?
Richard Meng:
If I were to talk about one thing, it was about the simplicity of processing unstructured data. And I think I've talked to one of the best data engineering voices in the field. I asked him, what do you think is the best? Why do you think is the reason why data people don't process unstructured app? And what is the future you see? I think he is seeing a similar thing that I do, which is there's simply no easy way for them to process. Even in the gen AI, if you go into a data analyst and ask them, write your own prompt, grab your data from f three bucket, and he or she does not even know where the data are. S three data unstructured are so far from what they can reach, and the current data stack is not optimized for the unstructured data. For example, you could not even visualize the data in the any data warehouse data like house product. Today, you cannot see a video. If you cannot see the video, then you cannot trust what you get from the LMD.
Richard Meng:
And all of the data processing we're doing today still needs some manual wiring. Getting data from s three bucket, shovel it into a large vision model, do some post processing, convert it into some newer metadata and putting it to a new, upload it into my data warehouse. There's a long chain of data processing. So, and I tried to. Our vision is we want to bring the extreme simplicity as like, as how Snowflake solved it, like try to solve it in 15 years ago, ten years ago. Data analyst does not need to learn about anything about, you know, how they're like, how their unstructured data is stored, how do they pull out from the bucket? How do you scale it? All you need to do is come in to Roe AI and start writing SQL queries to query and transform their unstructured data with AI models in SQL.
Demetrios:
And do you see it being something? Because I find this question or what you said fascinating. Wherever the data is so far away from folks, when it's in an s3 bucket, and an s three bucket is basically like a catch all. So data comes in from all these different sources that you have at your company. It could be internal data or things that maybe like HR is generating, or it could be external data. It could be website data, like click events, could be contracts. Whatever it may be, it can get thrown all into s three. And this is a catch all. It can be training videos, too.
Demetrios:
If we're talking about all of the different ways that you can get unstructured data, could also be podcasts like this one. There's many different ways that you can get that. And a data analyst, usually when they're touching data, they'll get the data and it'll be filtered through a few different steps like you mentioned, and it will usually go through, it will come out of this s3 bucket or the catch all, where all the data lives. And it just sits there and you'll pull, you don't pull all of the data, you just pull the relevant data, or what you hope is the relevant data, and then you pull it into some kind of a database that's optimized, ideally for your use. Case, is that how you see yourself living and coexisting with s three? So it's all right, we are optimizing, but it doesn't mean that everything's going to us directly. You're still going to have that s3. It's just going to be a lot easier for us to integrate with this big bucket of data and get you what you need. So at the end of the day, you're helping these data people live closer to the data and be more intimate with it.
Richard Meng:
Exactly. I think, Demetrios, we're capturing the way we do it is like you can imagine. Roe is plugin. Essentially it's a chrome plugin. You could immediately, once you connect it to us to s three, you can immediately get an interface where you can start processing those data, raw data from s three, into something that you can quantitatively analyze. And not only s three, we also tried that extends to all of the bucket storages, including Snowflake internal staging. We're going to be Snowflake native app as well. So that taps directly into snowflakes internal staging, which is also another s3, essentially within stopsake or databricks volume.
Richard Meng:
Those are all the places that we can tap into that we can be an OS system operating system on top of those data. Beyond that, you mentioned about public Internet for a lot of companies, right. Internet has a very cute attribute, which is, it's always refreshing. So we see companies don't always kind of persist those Internet data because by the time you analyze it, it's probably like stale. So what we have, we also have a crawler that allows, allows people to crawl the web pages live into an image or into a lawn, like into HTML, and then that can be further, like processed with the LMS data processors. And they don't need to build a crawler themselves in a side hustle. They can just like do it on Roe with SQL.
Demetrios:
That's super cool. So now the idea is what the end state that you're going for and really what you're trying to do is empower those data analysts and data engineers to better work with the data.
Richard Meng:
That's right.
Demetrios:
And what are some use cases you've been seeing?
Richard Meng:
Yeah. So talking about the web pages, one use case is competitor analysis, or simply merchant merchant. Understand merchant Kyc. For example, if a company wants to serve better for their clients, one of the best information source is their landing pages, right. What they're selling, how they're selling, how they're selling it, and so that the company can better curate different features for that client. And if you flipped around, right, you can also look at your competitors landing pages, you know, see are they selling right. Do they have any promotional events? When it comes down to the enterprise, operational data like gone costs like slack messages or meeting transcripts like what are doing, right. We're seeing a pinpoint where the default summarization tool is not enough, essentially from gong calls or from like I use read AI.
Richard Meng:
We want to do it in a customized way, right? Because as a CEO, for example, I do a lot of prospects costs as a CTO. My CTO, Jason, he does a lot of engineering costs. We care about different things, but today how we're solving it is we just import all of those transmitting transcripts data into our own data tool and start use different LLMs to process extract different insights from those transcripts at scale. So I care about either top pinpoint, the willingness to pay. My CTO cares about the blockers for why you're gonna need another week for a certain feature. So we can now we can extract different things from this different insights in our ERP.
Demetrios:
Incredible. Well, man, I'm very excited about what you're doing. I appreciate you coming on here and chatting with me about this. And I'm going to go and play around with Roe AI. It sounds like it is a really cool tool.
Richard Meng:
Thanks so much. Thanks for having me. Demetrios, asking great questions.