Deepgram Achieves Key Milestone on Path to Delivering Next-Gen, Enterprise-Grade Speech-to-Speech Architecture
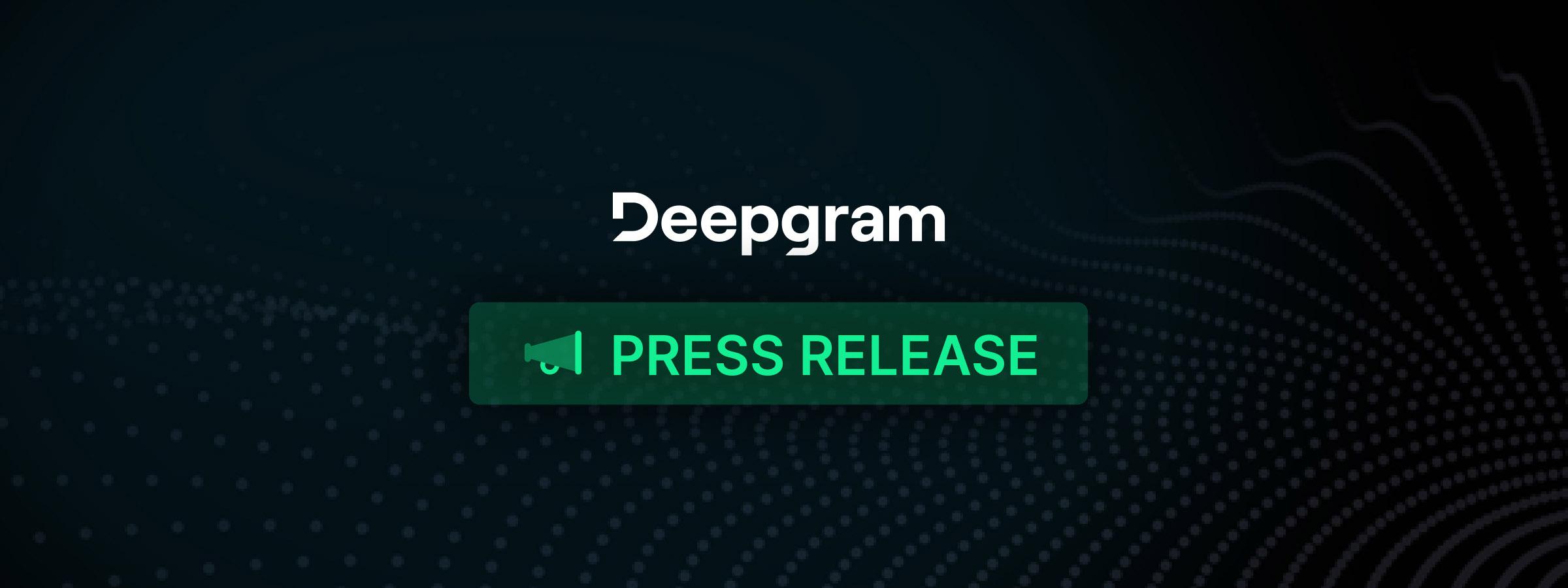
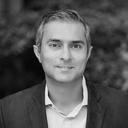
SAN FRANCISCO, February 18, 2025 – Deepgram, the leader in enterprise-grade speech AI, today announced a significant technical achievement in speech-to-speech (STS) technology for enterprise use cases. The company has successfully developed a speech-to-speech model that operates without relying on text conversion at any stage, marking a pivotal step toward the development of contextualized end-to-end speech AI systems. This milestone will enable fully natural and responsive voice interactions that preserve nuances, intonation, and emotional tone throughout real-time communication. When fully operationalized, this architecture will be delivered to customers via a simple upgrade from our existing industry-leading architecture. By adopting this technology alongside Deepgram’s full-featured voice AI platform, companies will gain a strategic advantage, positioning themselves to deliver cutting-edge, scalable voice AI solutions that evolve with the market and outpace competitors.
Advancements Over Existing Architectures
Existing speech-to-speech (STS) systems are based on architectures that process speech through sequential stages, such as speech-to-text, text-to-text, and text-to-speech. These architectures have become the standard for production deployments for their modularity and maturity, but eliminating text as an intermediary offers opportunities to improve latency and better preserve emotional and contextual nuances.
Meanwhile, multimodal LLMs like Gemini, GPT-4o, and Llama have evolved beyond text-only capabilities to accept additional inputs such as images, videos, and audio. However, despite these advancements, they struggle to capture the fluidity and nuance of human-like conversation. These models still rely on a turn-based framework, where audio input is tokenized and processed within a textual domain, restricting real-time interactivity and expressiveness.
To advance the frontier of speech AI, Deepgram is setting the stage for end-to-end STS models, which offer a more direct approach by converting speech to speech without relying on text. Recent research on speech-to-speech models, such as Hertz and Moshi, has highlighted the significant challenges in developing models that are robust and reliable enough for enterprise use cases. These difficulties stem from the inherent complexities of modeling conversational speech and the substantial computational resources required. Overcoming these hurdles demands innovations in data collection, model architecture, and training methodologies.
Delivering Speech-to-Speech with Latent Space Embeddings
Deepgram is transforming speech-to-speech modeling with a new architecture that fuses the latent spaces of specialized components, eliminating the need for text conversion between them. By embedding speech directly into a latent space, Deepgram ensures that important characteristics such as intonation, pacing, and situational and emotional context are preserved throughout the entire processing pipeline. What sets Deepgram apart is its approach to fusing the hidden states—the internal representations that capture meaning, context, and structure—of each individual function: Speech-to-Text (STT), Large Language Model (LLM), and Text-to-Speech (TTS). This fusion is the first step toward training a controllable single, true end-to-end speech model, enabling seamless processing while retaining the strengths of each best-in-class component. This breakthrough has significant implications for enterprise applications, facilitating more natural conversations while maintaining the control and reliability businesses require.
"This achievement represents a fundamental shift in how AI systems can process and respond to human speech," said Scott Stephenson, CEO and Co-founder of Deepgram. "By eliminating text as an intermediate step, we're preserving crucial elements of communication and maintaining the precise control that enterprises need for mission-critical applications."
This technical advancement builds on Deepgram's expertise in enterprise speech AI, with over 200,000 developers using its platform, more than 50,000 years of audio processed, and over 1 trillion words transcribed. Key benefits of the new architecture include:
Optimized latency design for faster, more responsive interactions
Enhanced naturalness, preserving emotional context and conversational nuances
Native ability to handle complex, multi-turn conversations
Unified, end-to-end training across the entire model, creating a more cohesive and inherently adaptive system that fine-tunes its understanding and response generation directly in the audio space.
Utilizing Transfer Learning for Cost-Efficient, High-Accuracy Speech-to-Speech
Deepgram’s research in the space is accelerated by its use of transfer learning and best-in-class pre-trained models, allowing it to achieve high accuracy with significantly less training data than traditional methods. Without latent techniques, training a model at the scale needed for speech-to-speech would require over 80 billion hours of audio—more than humanity has ever recorded. However, Deepgram’s latent space embeddings and transfer learning approach achieve superior comprehension while significantly reducing costs, maintaining interpretability, and accelerating enterprise deployment. This efficiency enables Deepgram to deliver scalable, end-to-end speech AI that meets the demands of real-world voice applications.
Empowering Developers with Full Debbugability
One of the requirements in enterprise speech-to-speech modeling is the ability to understand and troubleshoot each step of the process. This is particularly challenging when text conversion between steps isn’t involved, as verifying both the accuracy of the initial perception and the alignment of the spoken output with the intended response is not straightforward. Deepgram recognized this need and addressed it by designing a new architecture that enables debuggability throughout the entire process.
This architecture allows developers to inspect and understand how the system processes spoken dialogue. The design incorporates speech modeling of perception, natural language understanding/generation, and speech production, preserving distinct capabilities during training. Through the ability to decode intermediate representations back to text at specific points, developers can gain insight into what the model perceives, thinks, and generates, ensuring its internal representation aligns with the model output and stays true to the intent of the business user, addressing hallucination concern in scaled business use cases. This capability allows the user to peer into each step throughout generation, helping refine models, improve performance, and deliver more accurate, lifelike, and reliable speech-to-speech solutions.
Beyond Speech-to-Speech – A Complete, Enterprise-Ready Voice AI Stack
While building an advanced speech-to-speech (STS) model is a major technical achievement, enterprises need more than just a model—they need a complete, scalable platform that ensures seamless deployment, adaptability, and cost efficiency. Deepgram delivers not just cutting-edge STS technology, but an enterprise-ready infrastructure designed for real-world applications.
Seamless Integration & Continuous Improvement – Once Deepgram’s end-to-end STS model moves to production, businesses will be able to adopt this breakthrough directly through our developer-friendly voice agent API from within the current Deepgram platform. Through continued innovation, enterprises will benefit from the latest advancements, ensuring seamless integration and a future-proof platform for their voice AI applications.
Enterprise-Grade Performance & Cost Efficiency – Built for low customer COGS, our platform enables enterprises to deploy high-performance voice AI without excessive costs. This ensures scalability, whether for customer service automation, real-time voice agents, or multilingual applications.
Full-Featured Platform and High-Performance Runtime – Deepgram’s platform includes powerful capabilities such as:
Adaptability - Dynamically fine-tune models for specific industry language, ensuring high accuracy across diverse applications without needing constant retraining.
Automation - Streamline transcription, model updates, and data processing, reducing overhead and accelerating deployment.
Synthetic data generation - Generate synthetic voice data to improve model training, even with limited real-world data, enhancing accuracy for niche use cases.
Data curation - Clean, manage, and organize training data to ensure high-quality, relevant input, improving model performance.
Model hot-swapping - Seamlessly switch between different models to optimize performance for specific tasks.
Integrations - Effortlessly integrate Deepgram’s voice AI with cloud platforms, enterprise systems, and third-party applications, embedding it within existing workflows.
With Deepgram, enterprises don’t just get speech-to-speech—they get the most advanced, enterprise-ready voice AI platform, designed for real-world deployment and long-term innovation.
For more information about Deepgram's novel approach for speech-to-speech, read the technical brief. To learn more about Deepgram's suite of voice AI infrastructure, visit www.deepgram.com.
Additional Resources:
Explore the technical brief on Deepgram’s novel speech-to-speech architecture
Watch a fun demo of Deepgram’s voice agent API
Try Deepgram’s interactive demo
Get $200 in free credits and try Deepgram for yourself
About Deepgram
Deepgram is the leading voice AI platform for enterprise use cases, offering speech-to-text (STT), text-to-speech (TTS), and full speech-to-speech (STS) capabilities. 200,000+ developers build with Deepgram’s voice-native foundational models – accessed through cloud APIs or as self-hosted / on-premises APIs – due to our unmatched accuracy, low latency, and pricing. Customers include technology ISVs building voice products or platforms, co-sell partners working with large enterprises, and enterprises solving internal use cases. Having processed over 50,000 years of audio and transcribed over 1 trillion words, there is no organization in the world that understands voice better than Deepgram. To learn more, visit www.deepgram.com, read our developer docs, or follow @DeepgramAI on X and LinkedIn.
Unlock language AI at scale with an API call.
Get conversational intelligence with transcription and understanding on the world's best speech AI platform.